Agriculture
Boost agriculture AI with Keylabs' expertly accurate annotation services.
Data annotation tools have become an essential component in various industries, including agriculture. With the rise of precision agriculture and the use of artificial intelligence (AI) and machine learning (ML), accurate data has become critical for farmers to make informed decisions. Data annotation involves the process of labeling, categorizing and tagging data to make it usable for machine learning algorithms. In agriculture, this process is crucial for identifying crop types, detecting diseases and predicting yields.
The agriculture industry is constantly evolving and technology is playing a crucial role in its development. At Keylabs, we understand the importance of data annotation in agriculture.
In this article, we'll explore the various ways in which Keylabs' data annotation platform can benefit the agriculture industry. We'll look at real-world examples of how our platform has been used to develop successful agriculture apps and we'll explain why our platform is the perfect choice for farmers and developers alike.
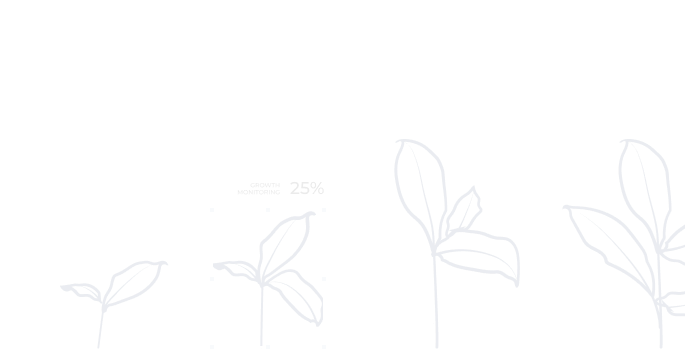
Annotation tools in agriculture applications
Aerial crop analytics
Aerial crop analytics has become an essential tool in precision agriculture. Powered by AI technology and drone geospatial intelligence platforms, these tools enable farmers to monitor their crops more efficiently and detect pest infestations and plant diseases early on.
By using smart spraying and seeding techniques based on data collected through aerial surveys and imaging, farmers can increase their agricultural efficiency while reducing costs.
Automated harvesting and fruit picking
Accurate detection and localization of fruit are crucial for successful automation in agriculture. This is where data annotation platforms like Keylabs come into play. Keylabs provides annotations for all types of images and videos to create the best training data for computer vision projects. With high-quality training data, automated harvesting systems can accurately detect ripe fruit without harming unripe or overripe ones.
Grading and sorting
Automating produce grading and sorting with AI computer vision can save time, reduce labor costs and increase accuracy. Keylabs data annotation platform is a perfect tool for agriculture apps looking to implement this technology.
In addition to traditional image datasets, Keylabs also offers perfect video annotation that supports object tracking for grading and sorting purposes.
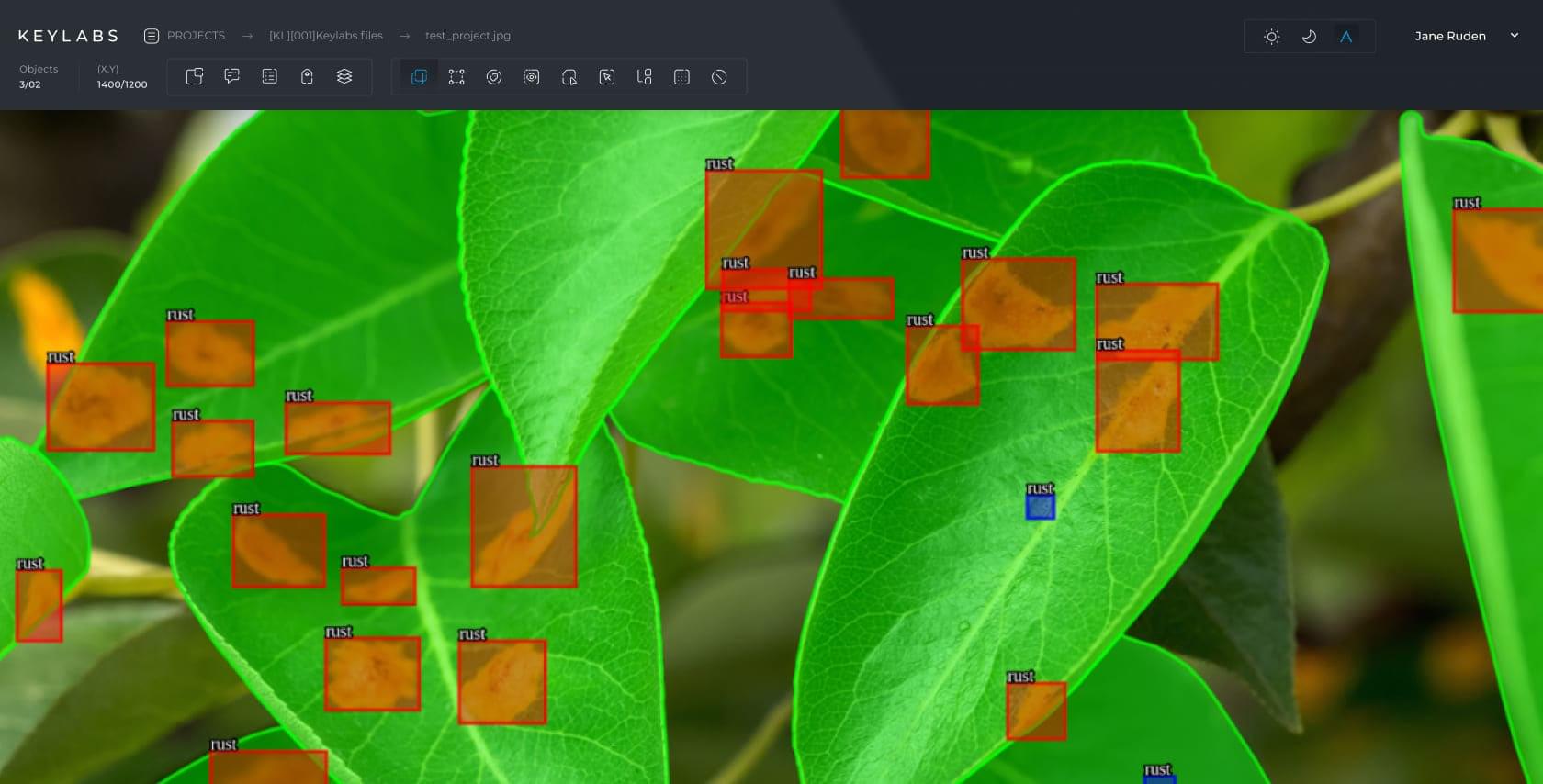
Pest and plant disease detection
Precision agriculture is a field that aims to use technology to optimize crop production and increase yield. One area of research that has gained significant importance in this field is plant disease and pest detection using machine vision.
This method utilizes deep learning approaches to train models on large datasets of images of diseased or pest-infected plants. These models can then be used for early detection and prevention, leading to improved crop yield.
Yield estimation
Accurate yield estimation is critical in agriculture as it enables farmers to make more informed decisions on resource management. Remote sensing (RS) systems have emerged as a powerful tool to improve yield production and nitrogen management.
Sophisticated models such as crop simulation and yield estimation models can provide accurate estimates of crop yields based on various inputs such as soil moisture, temperature, rainfall records and nutrient levels.
Data annotation tools are used to label various elements within the collected data, such as lane markings, road boundaries and other important features. This annotated data is then used to train AI models responsible for lane recognition in self-driving cars. Accurate and diverse labeled data helps these models learn to recognize and interpret different types of lanes, road conditions and lighting situations, allowing the car to make appropriate adjustments to its driving behavior.
Key features
There are several key features that a robust data annotation tool for self-driving cars should possess:
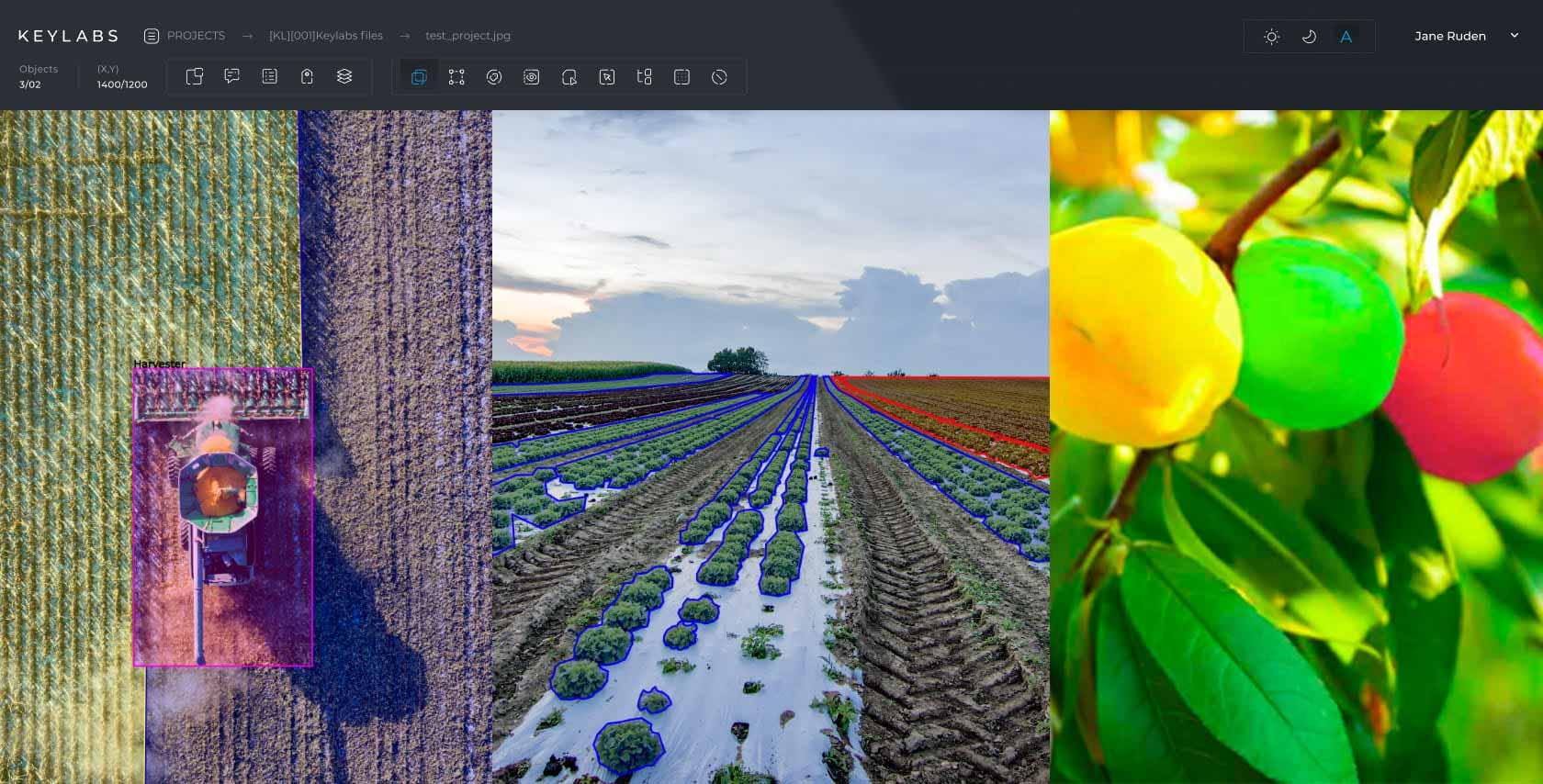
Versatility
The tool should be capable of handling different types of data, including 2D and 3D images, videos and point clouds generated by LiDAR sensors.
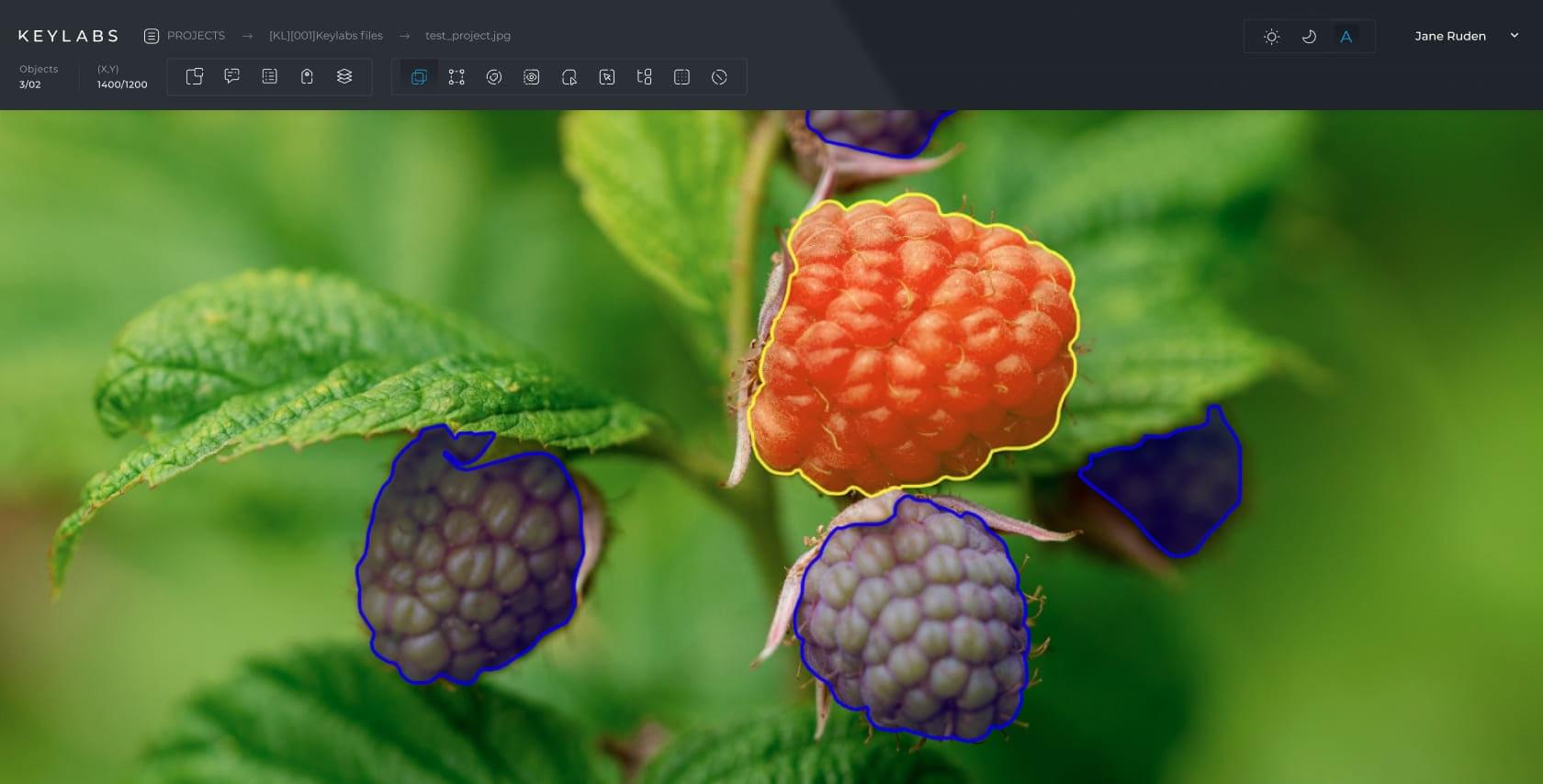
Precision
High-quality annotations are crucial for the accuracy of AI models in aerial management and disaster management. The tool should enable precise labeling of objects and features, minimizing the chances of misinterpretation.
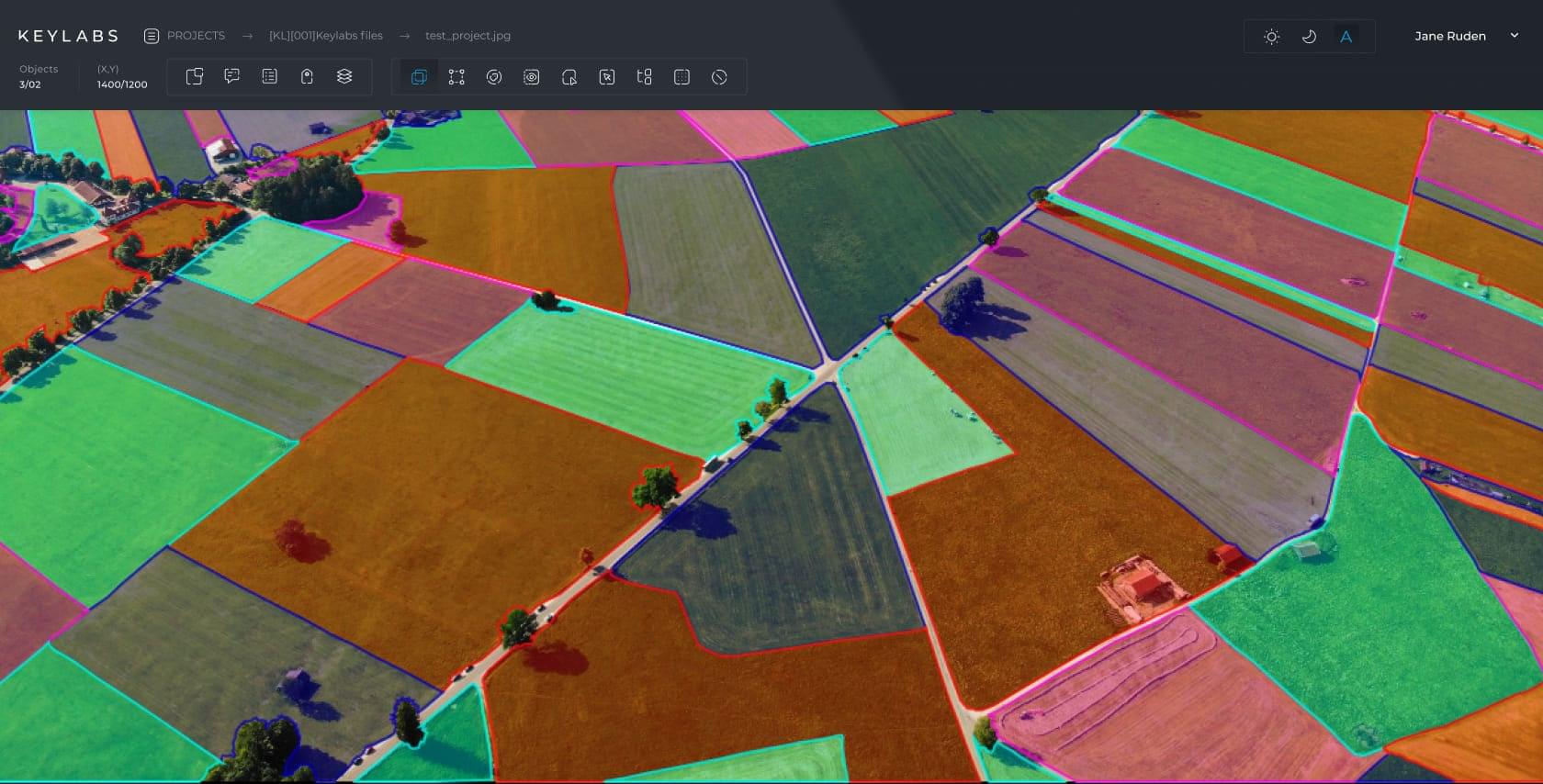
Scalability
A data annotation tool should be scalable to handle large datasets efficiently, streamlining the annotation process and reducing the time required for model training.
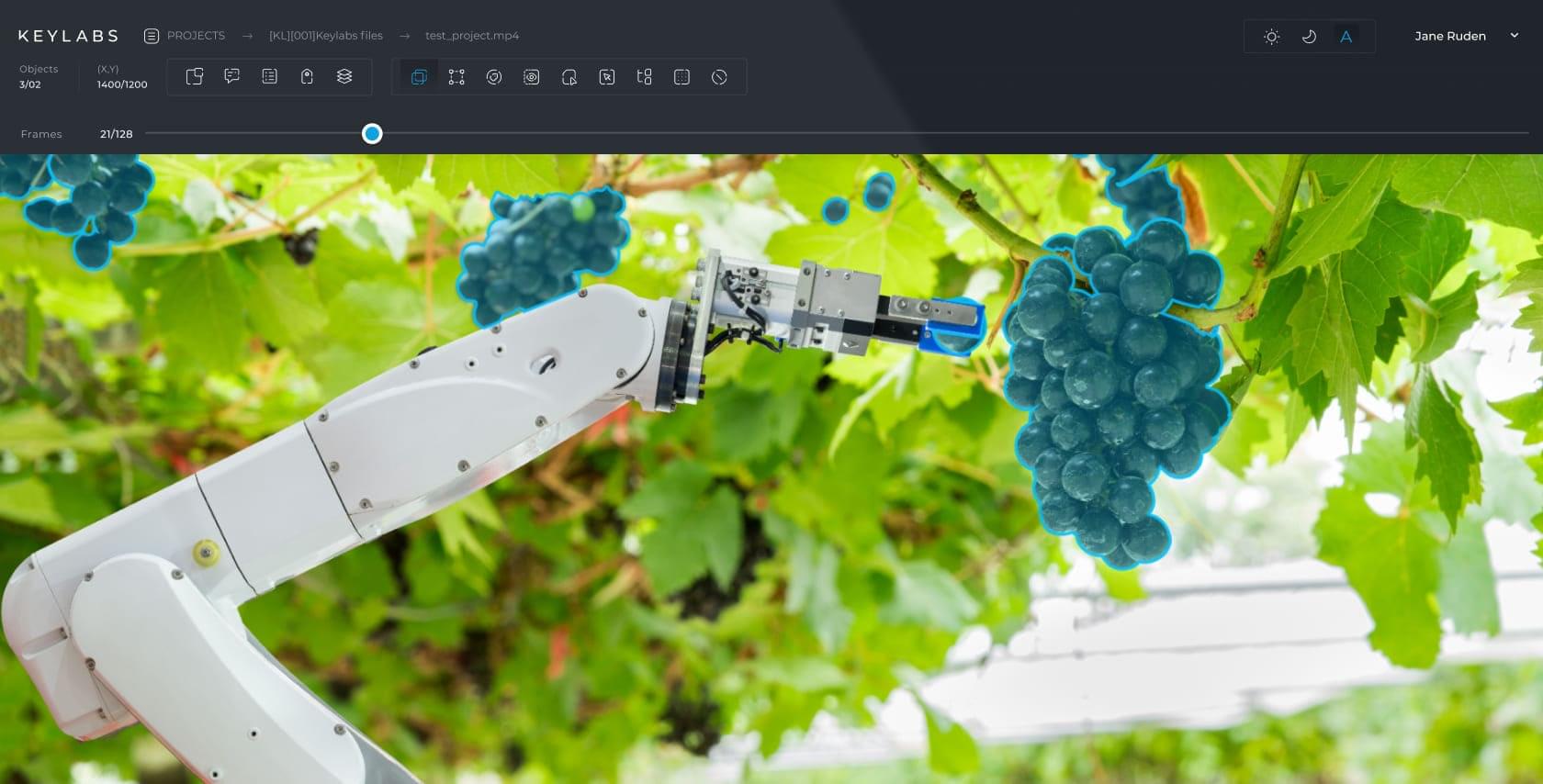
Automation
AI-powered data annotation tools can leverage machine learning algorithms to automate parts of the annotation process, speeding up the workflow and increasing overall efficiency.
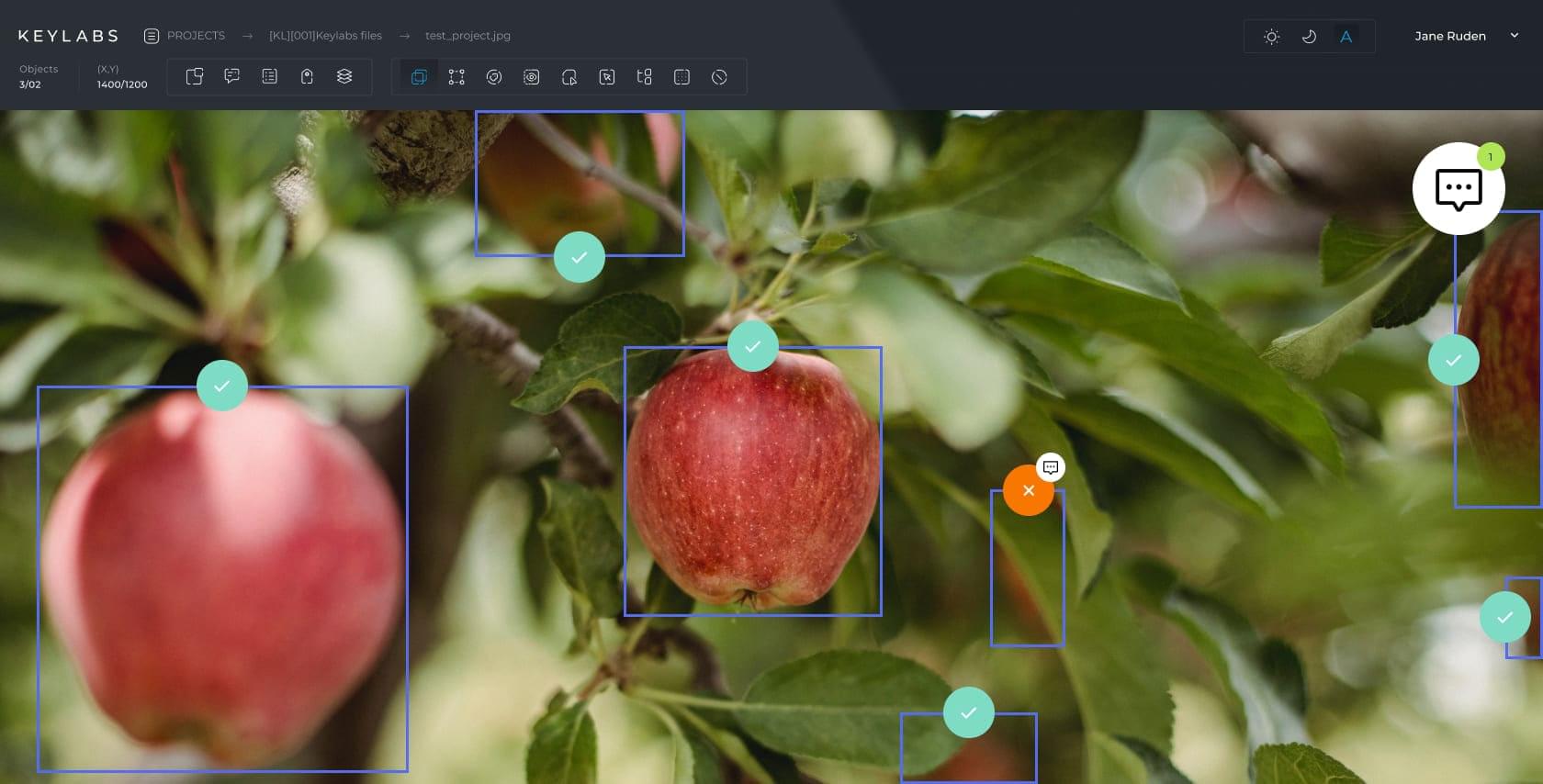
Collaboration
A good data annotation tool should facilitate collaboration among team members, enabling multiple annotators to work together on the same dataset. This ensures consistency in labeling and accelerates the annotation process.
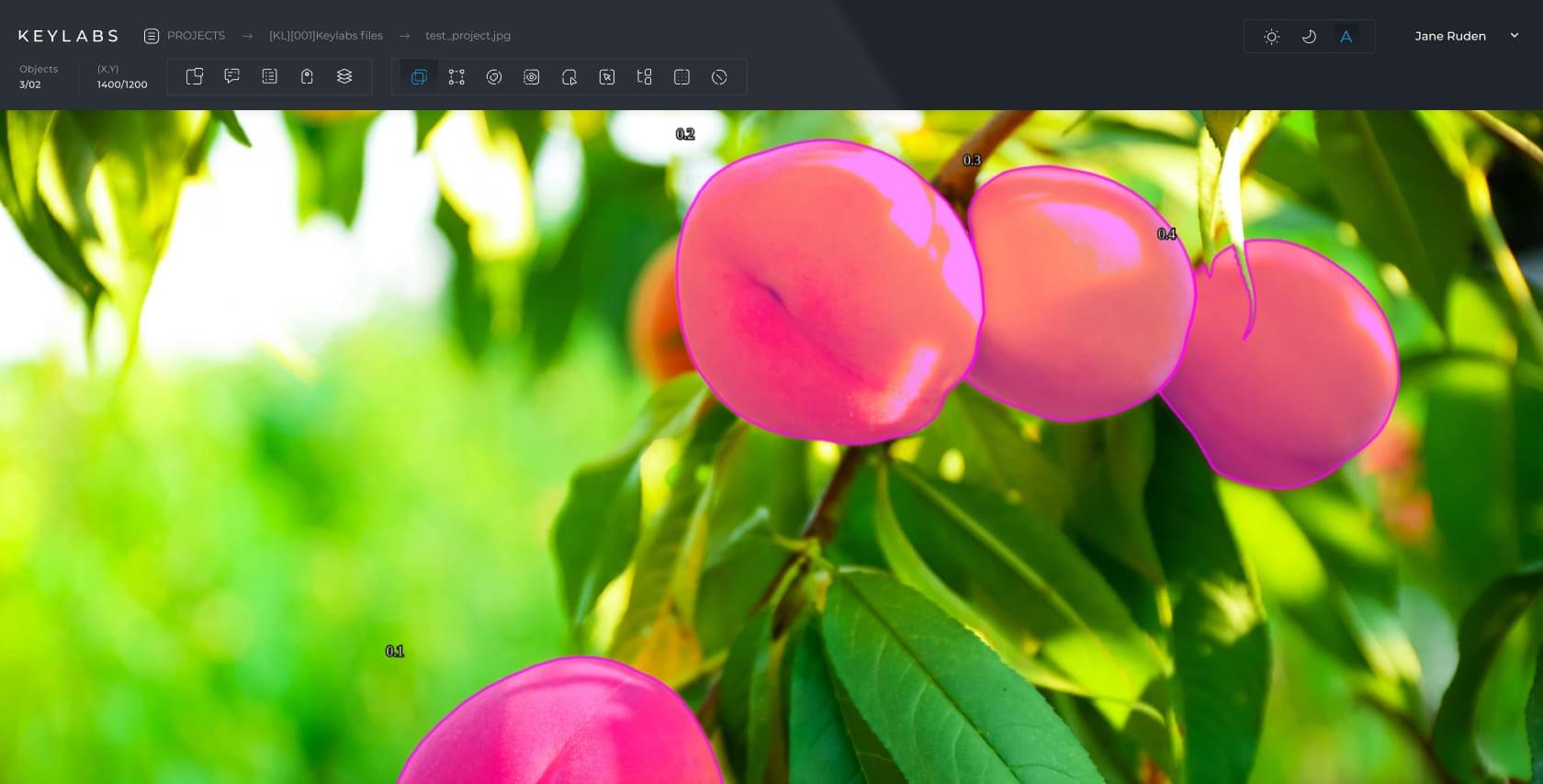
Quality control
To ensure the highest level of accuracy, the tool should have built-in quality control features that allow for easy review and verification of annotated data. This helps maintain data integrity and improves the overall performance of the AI models being trained.
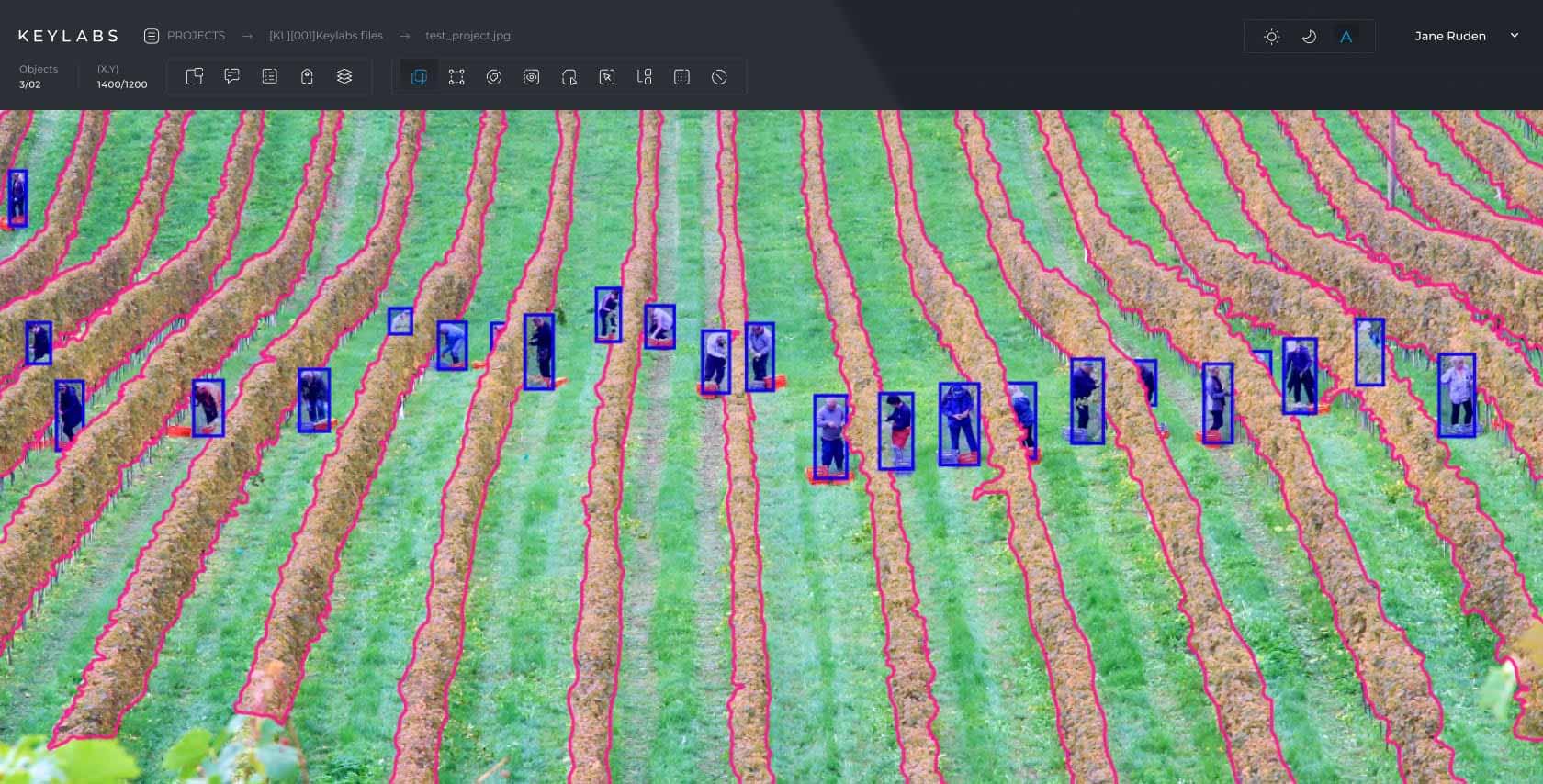
Customization
The annotation requirements may change or become more complex. A flexible data annotation tool should allow for customization to meet the unique needs of each project and adapt to new challenges in the industry.
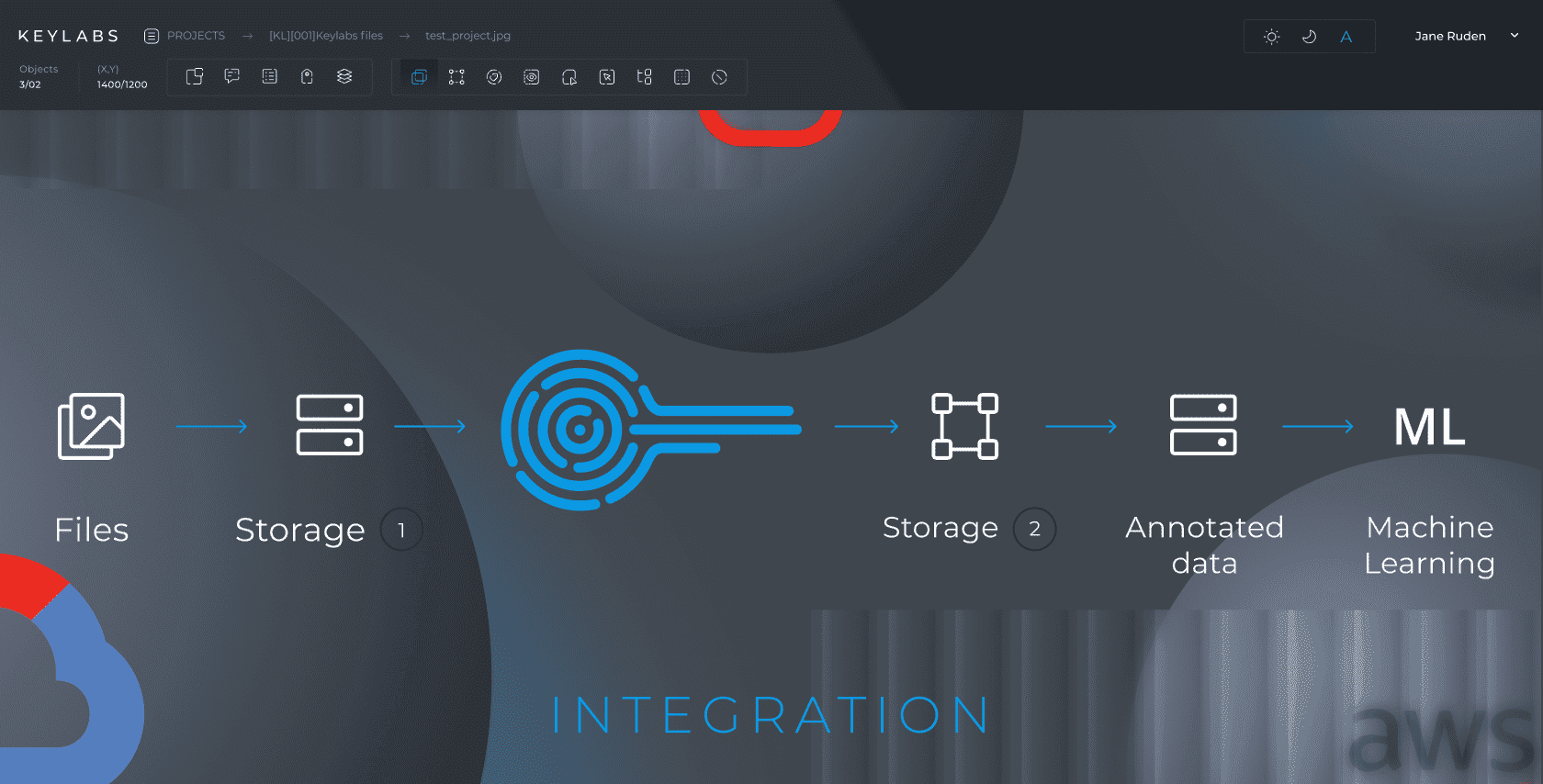
Integration
The data annotation tool should be able to integrate seamlessly with various machine learning frameworks and platforms, making it easier for developers to use the annotated data for model training and evaluation.
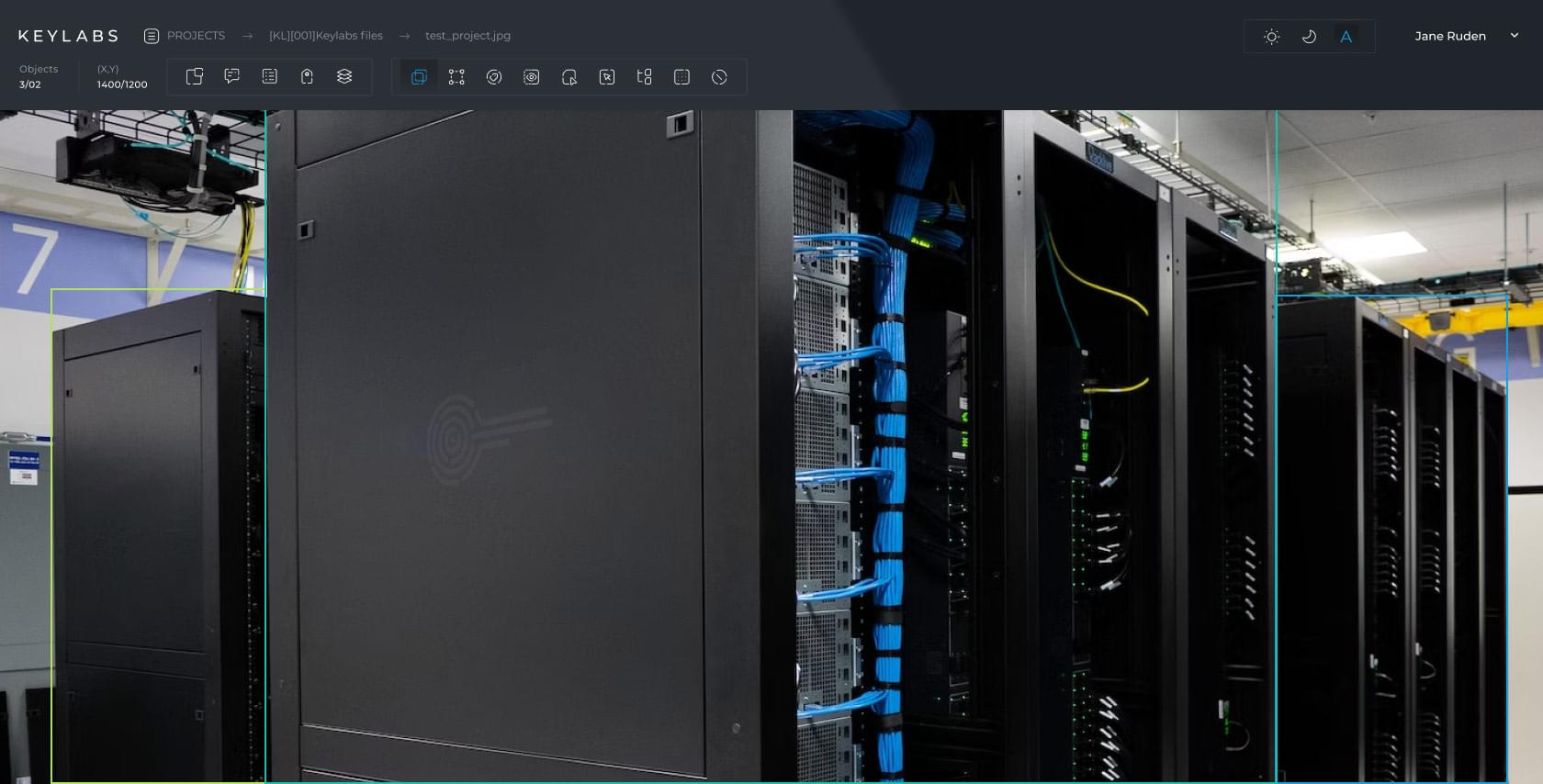
Data Security
A data annotation tool must prioritize data security and privacy, ensuring that the information is protected at all stages of the annotation process.
Versatility
Precision
Scalability
Automation
Collaboration
Quality Control
Customization
Integration
Data Security
Use cases
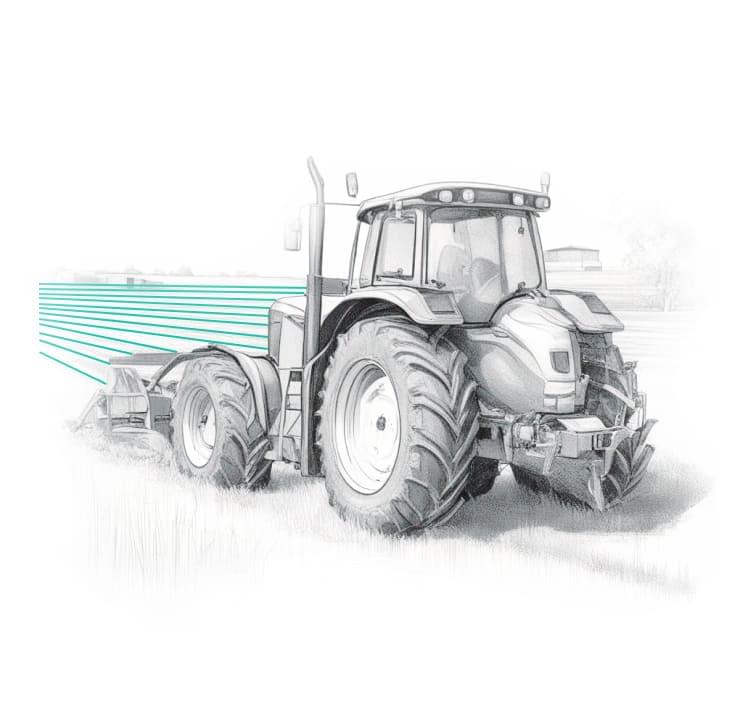
Aerial crop analytics
Fully compatible with all data formats, our platform ensures meticulous annotation, critical for accurate crop health assessments and yield predictions.
Automated harvesting and fruit picking
The data annotation platform powers advanced harvesting and fruit picking applications. With a diverse suite of labeling tools, we provide high-quality datasets specially tailored for the precise identification of ripe produce.
Grading & sorting. Disease detection
Keylabs platform, adept in handling diverse data formats, provides precise annotation, accurate pest and plant disease detection, leading to more effective crop management.
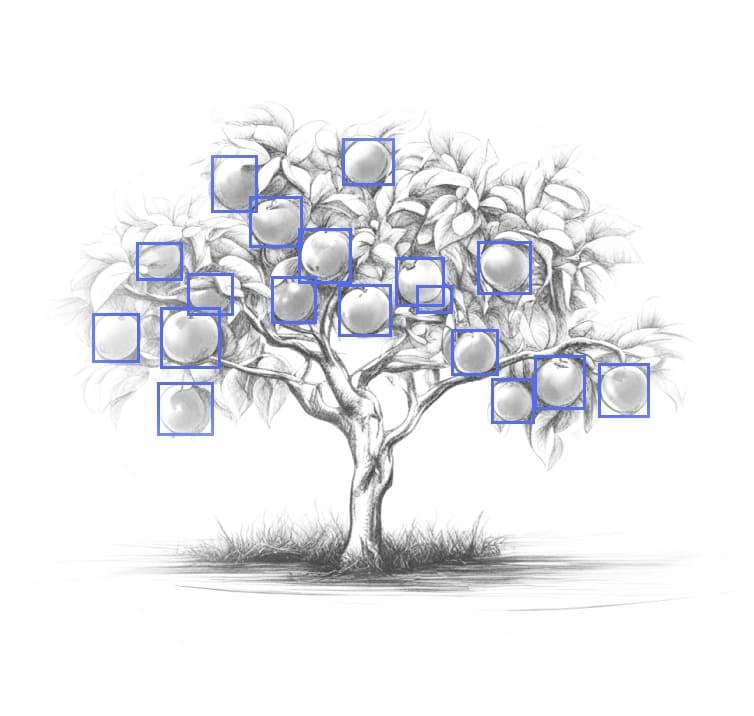
Yield estimation
Keylabs platform, compatible with all data formats, ensures meticulous annotation — enabling reliable yield predictions that empower smarter, more efficient farming.
Starter’s guide
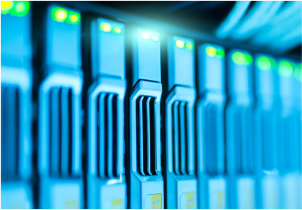
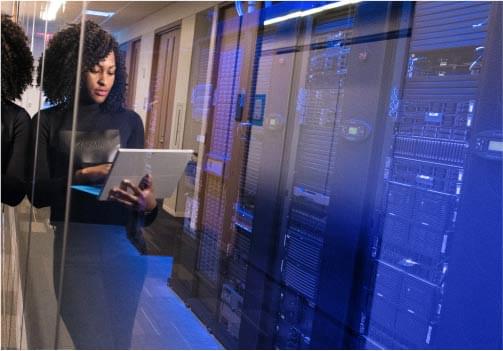
Data security
Using the Keylabs annotation tools comes with a commitment to data safety. Keylabs employs a range of security measures to protect valuable and sensitive data. This includes comprehensive infrastructure security if you choose to access Keylabs through the cloud. Alternatively, Keylabs can be installed on premises, guaranteeing you total control over access. We will continue to emphasize data protections as a priority by utilizing a diverse array of security measures and industry best practices.
Top Features
Keylabs is created as a platform that incorporates state-of-the-art, performance oriented tools and processes.
ML assisted data annotation
ML assisted data annotation
Keylabs is a streamlined data labeling platform with AI-enhanced annotation.
Tailored for easy Integration with any client model and time & cost efficiency.
Keylabs’ advanced algorithms provide quick, accurate data prep for superior model training.
3D tool
3D tool
Keylabs is a super-fast tool, soaring through Lidar files at ultra speeds. It seamlessly handles all file formats, ensuring a consistent, efficient workflow regardless of file complexity.
High performance video annotation
High performance video annotation
With the Keylabs platform's technical and software capabilities, video annotation is highly accurate (precision of up to 99,9% depending on project needs) and fast. Thanks to the geolocation adaptation of servers, even big-sized videos are loaded and processed quickly.
Magic wand
Magic wand
Speeds up the annotation process by automatically detecting closed shapes of the same color or color gradient in a highly precise manner.
Object interpolation
Object interpolation
Object interpolation in the data annotation process is used to accelerate the annotation of objects across a sequence of frames in video annotation.
Annotators label the shape of an object in the first and the last keyframe of desired sequence and the object interpolation algorithm automatically generates the labels for the object in the intermediate frames.
It saves time and also ensures consistent labeling across frames.
A-Z order
A-Z order
Objects can be placed on different leveled layers, which allows operators to correctly detect and work with those objects and their boundaries.
Multilayer annotation
Multilayer annotation
Multilayer annotation is a complex yet valuable process in data annotation where different types of materials are layered onto a single item.
This allows the addition of multiple, diverse annotations to a single piece of data such as an image or video frame.
Each layer might provide a different dimension of information, enriching the dataset with multiple facets of detail.
This allows the addition of multiple, diverse annotations.
Object linking
Object linking
Object linking in the data annotation process is a valuable function that connects different instances of the same object across multiple frames or images.
For example, in video annotation, an object appearing in different frames is linked throughout the video, ensuring the continuity and consistency of the annotation.
Hierarchical atributes
Hierarchical atributes
The attribute is a type of tag that can be applied to a class or property to provide metadata about it.
Using attribute hierarchies, it is possible to define structures of metadata for each item in dataset.
It is achieved by using dependent attributes, which allows logical forming of metadata information for frame or object individually.
Workflow and task distribution
Workflow and task distribution
Workflow includes custom stages of one of 4 project stage types: annotation, verification, miscellanious and final.
Good workflow and task distribution ensure that the data annotation process is smooth, efficient and completed within the required timeframe.
Data management
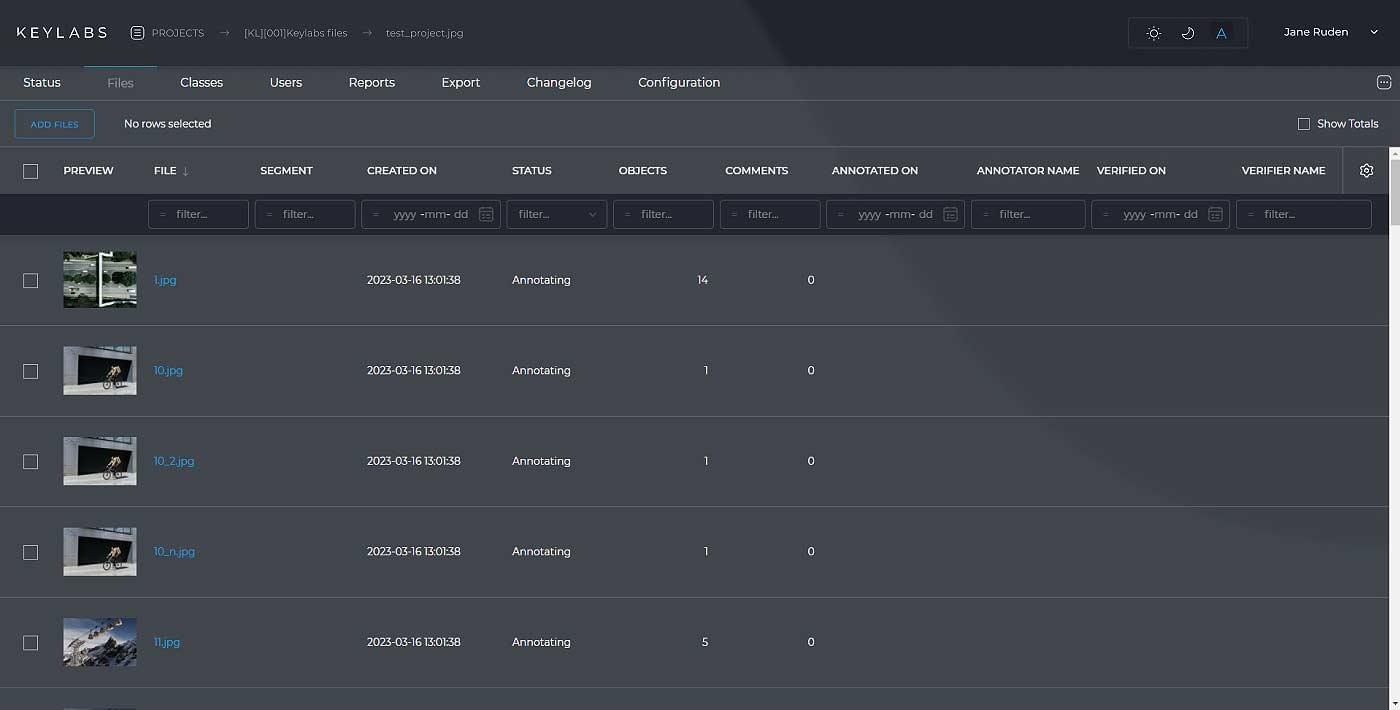
Data management
Data management in the context of the data annotation process is about strategically handling and organizing the data throughout its lifecycle.
Effective data management helps to uphold data integrity and ensure that the final annotated data is accurate, consistent and ready for use in AI and machine learning projects.
Attributes interpolation
Attributes interpolation
Attributes metadata can also be interpolatable (changed) between the frames. For instance, In a self-driving car video annotation, this can label a car as a "sedan" going "30 mph" in the first frame & automatically estimate its type & speed in subsequent frames until the next key frame. This eliminates the need for manual annotation in each intervening frame, saving time & effort.
Annotation types
Keylabs gives developers access to a full suite of annotation techniques:
Bounding Box
A rectangular box defined by coordinates that encapsulates an object of interest within an image
Oriented bounding box
A rotated rectangle that tightly encloses an object, accommodating its orientation and shape more precisely than a standard bounding box
Polygon
A closed plane figure made up of several line segments that are joined together, used to define irregular shapes in an image
Points
The Point Annotation Tool places dots on images or videos, ideal for highlighting details like facial features, expressions and body postures
Lines & Multilines
A data annotation tool used to draw single or multiple interconnected lines on images, capturing linear features or paths
Skeleton
A thin version of a shape, representing its central structure and providing a simplified representation of its form, commonly used in understanding object morphology or structure
Instance Segmentation
The process of classifying and delineating each individual object instance in an image
Semantic Segmentation
The classification of each pixel in an image based on its semantic category, without distinguishing between individual object instances
Bitmask
A binary representation where each pixel value indicates whether it belongs to the object (1) or the background (0)
Cuboid
A 3D rectangular prism annotation, often used to represent objects in spatial dimensions
Mesh
A collection of vertices, edges and faces that define the shape of a 3D object in space, often used in 3D modeling and computer graphics
3D Point Cloud
A collection of data points in a three-dimensional coordinate system, representing the external surface of an object
Custom
A tailored data annotation tool designed to cater to specific annotation needs not covered by standard tools