5 Signs Of A Healthy Labeling Tool Project [High-Quality Practices]
How can we evaluate annotated data? If a project is ongoing, how can we keep it from derailing? Data annotation is complex. Complicated systems have many points of failure. It’s a shame to compare something to a home’s foundation for the millionth time, but it applies here. There are a lot of core components that collaborate to form a high-quality dataset.
So how do we check the pulse of a healthy data annotation project? Here’s a list of the five major attributes of a successful data labeling project. It’s not an exhaustive list, and these are a few tell-tale signs. We’ll talk about some best practices and the professionals that make them possible.

Data Annotation Instructions Are Understood By The Data Labeling Team
The annotators clearly understand the task at hand. They move through the images or video, labeling accurately. When the project leader reviews their results, they can see that their instructions were understood.
How To Make This Happen
It’s best, to begin with a small scope. Give the annotation team simple tasks and have an “open door policy” for all questions. Everybody should understand what’s expected. Creating clear outlines is useful for annotators to refer to.
The Consequences Of Not Giving Clear Annotation Instructions
Smaller miscommunications have a snowball effect. Mislabeling at the beginning of a project will cause major reliability issues later on. That might create data that can’t be trusted, wasting effort and budget along the way.
The Data Annotators Are Quality-Screened With High Accuracy Scores
Hiring an inaccurate team of annotators could leave a project with questionable results. That sort of inconsistent data could create a range of data biases for automated programs. Expert annotators are even more crucial in specialized domains.
It’s common to use hybrid human-machine tools to speed up labeling. Automation platforms pair the best data-labeling tools with a supervised auto-labeling tool.
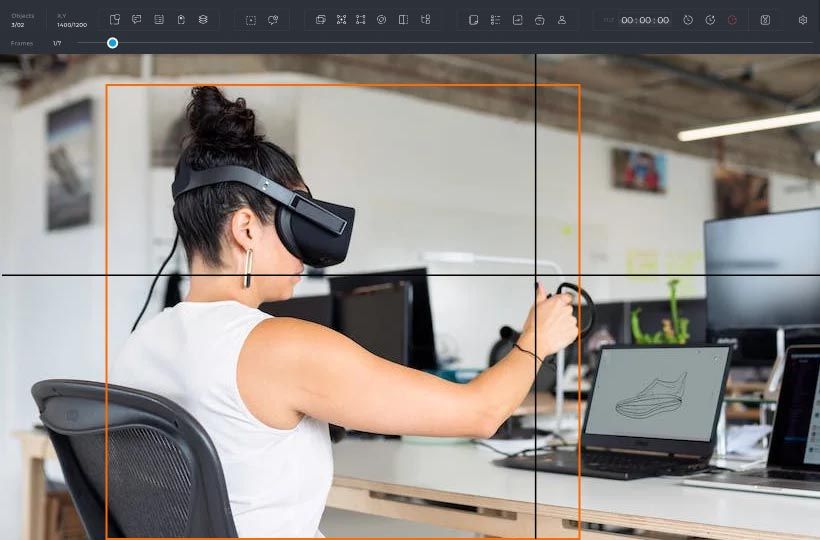
How To Build A Team Of Expert Annotators And Measure Their Value
Project leaders should be screening applicants and measuring their accuracy. Perhaps their portfolio already demonstrates a high level of accuracy. If it doesn’t, set a rate of accuracy for them to meet. It’s vital for the project’s data quality.
Inaccurate Data Annotators Undermine Projects And Create Data Bias
There’s no doubt about it. Inaccurate data labeling is bad news for data annotation projects. This is not an area to cut corners or save money. Hire annotators individually or get in touch with a data labeling company.
The Work Of Data Annotators Is Constantly Reviewed And Monitored
A data annotation project is not something you can ever let off the leash. Compiling accurate data means constant review, sometimes by a secondary layer of annotators. These team members don’t annotate. They’ll check the data labeling done by the first layer of personnel, correcting errors and adding labels.
Supervising Annotators Will Help Projects Meet Higher Accuracy Demands
It’s easiest to select the best annotators from the initial hiring and give them supervisory roles. The standards for data quality are higher than ever. Building in an extra layer of redundancy undeniably raises the quality of the final dataset.
The Project Uses A Consensus Pipeline With Input From Multiple Sources
This is very similar to the last measure of a successful data annotation project. The difference is a consensus pipeline has even more eyes to review labels. Instead of one or two, there might be four separate annotators coming to a consensus on a single label.
It’s not a review process. It’s multiple annotators completing the same task. As a benefit, project managers can measure individual data annotators against the accuracy of their coworkers. If five annotators tag an object as a “tree,” but one tags it as a “shrub,” then you can safely eliminate that selection.
Forming A Consensus Pipeline In-House Or By Outsourcing
Project managers can use existing annotators for this or outsource the review process. It all depends on how specialized the domain is. Outsourcing might be more budget-friendly and allow your annotators to focus on other things.
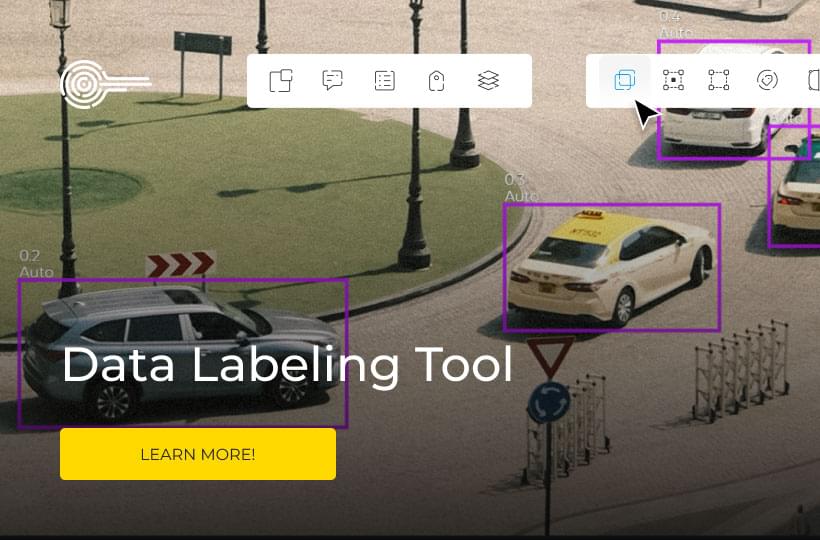
The Project Is Evaluated With Benchmarks And Ongoing Accuracy Tests
There’s no better way to give a data annotation project a health check. You’re going to ask questions that you already know the answer to. If your program can arrive at the right answer, the project is on track. It’s a good idea to do this at various points throughout the project.
Keeping annotators aware of the benchmark and periodically checking it will keep a project on track. Using a benchmark also highlights annotators with lower data labeling tool accuracy. Project managers should reiterate quality standards and provide training to those labelers.
How To Create Benchmark Data With Examples
Also known as “golden” or “benchmark” datasets, benchmarks are tests with answers. Creating this example dataset is easy, but they need a broad range. The project won't be truly evaluated if the benchmark isn’t large enough.
Each class of data must be represented. For example, let’s take a look at autonomous driving. In a benchmark evaluation, which categories should be checked? Different driving scenarios should be evaluated, like snowy, wet, or dry road conditions. Then there’s driving in traffic, pedestrian awareness, and countless other situations to monitor. There needs to be a benchmark dataset for each area.