AI and Annotation Tools in Agricultural Robotics: Improving Crop Management and Efficiency
Agricultural robotics data annotation leads to enhanced crop efficiency. These technologies enable farms to adopt data-driven strategies, ensuring precision in planting, monitoring, and harvesting. Advanced systems, powered by artificial intelligence, analyze vast amounts of data from sensors to satellite imagery. This ensures optimal crop development and resource use.
AI facilitates precise crop management through predictions and automates machine control. This reduces manual labor, boosting productivity and sustainability in agriculture.
For example, algorithms have achieved a 99.31% success rate in detecting tomato maturity and a 90.4% accuracy in diagnosing apple black rot. These smart farming tools significantly improve agricultural efficiency. The "Weed Terminator" system, which delivers precise herbicide amounts, showcases the importance of precision in modern farming.
Key Takeaways
- AI and annotation tools drive data-driven farm management, boosting crop efficiency.
- Algorithms achieve over 90% accuracy in identifying and diagnosing various crop diseases.
- Precision weed removal systems contribute to sustainability and environmental friendliness.
- Historical and contemporary data integration enables precise crop management forecasts.
- AI adoption in agriculture mitigates labor shortages and increases productivity.

Introduction to Agricultural Robotics
Agricultural robotics is transforming farming by introducing advanced technologies like autonomous tractors, drones, and robotic planters. These innovations automate critical farming tasks, reducing the need for extensive human labor. This leads to increased efficiency and sustainability in modern agriculture.
Overview of Robotics in Agriculture
Robots in agriculture have a wide range of applications. They can plant seeds, plow fields, control weeds, and pick fruits and vegetables. This showcases how robotic solutions can simplify farming practices. Through robotic data labeling, these systems learn to perform tasks with high precision. They adapt to various agricultural environments, improving crop management.
Benefits of Automation in Farming
Automation in farming offers significant benefits. It reduces labor costs and minimizes human error, leading to more consistent and accurate operations. Automation also increases precision in planting and harvesting, resulting in higher crop yields. By integrating machine learning in agriculture, these systems optimize their functions, adjusting to specific field conditions. This enhances resource efficiency, supporting sustainable farming practices.
Companies like John Deere and AgEagle Aerial Systems provide advanced, autonomous farming technologies. These technologies leverage autonomous farming data labeling and AI frameworks to manage agricultural processes seamlessly. They address labor shortages, improve monitoring, and enhance productivity growth. This ensures a sustainable and profitable future for the agricultural industry.
Feature | Impact |
---|---|
Precision Planting | Improves crop stands and reduces waste |
Weed Control | Uses less herbicide, lowers labor costs |
Fruit Picking | Prevents damage, ensures timely harvest |
Aerial Imaging | Monitors crop health, soil conditions, and growth |
The Importance of Data Annotation
In the evolving field of agricultural robotics and AI, data annotation's significance is immense. Precision agriculture AI tagging and agricultural automation data labeling are key to these advancements. They enable more intelligent and efficient farming practices. By mid-century, the demand for food is expected to surge by 70% due to population growth. Accurate data annotation is critical to enhance AI models in addressing climate change and food security challenges.
Defining Data Annotation in Agriculture
Data annotation in agriculture involves tagging relevant information from diverse sources like sensors, drones, and aerial images. These tags label data according to contextual parameters such as crop type, health status, and environmental conditions. Data annotation services are in high demand, with a focus on image and video annotation for AI projects. This process is essential for training AI models, supporting smarter decision-making tools for precision farming, predictive analytics, and effective crop disease management.
Role of Data Annotation in AI Training
High-quality data annotation directly influences AI system performance. For instance, AI in farming has boosted productivity by 67% in recent studies. Automated weed control using AI can reduce herbicide usage by up to 90%. The accuracy of agricultural automation data labeling is, then, of utmost importance. Accurate data ensure AI models can interpret and react to various agricultural scenarios. This is vital for innovations like computer vision-based crop production and monitoring systems or intelligent spraying for pest and disease detection. Proper annotation allows AI to achieve high accuracy rates, such as 95% in detecting apple scab and 92% for tomato leaf blight.
Types of Agricultural Robotics
Modern agriculture is undergoing a significant transformation with the introduction of autonomous robotic systems. These specialized robots are boosting efficiency and precision in various farming tasks. By utilizing agricultural drone data annotation and computer vision, we are entering a new era in crop management and yield enhancement.
Autonomous Tractors and Harvesters
Autonomous tractors and harvesters are transforming the agricultural sector. Equipped with GPS and computer vision, they enable precise navigation and operation across large fields. A leading autonomous vehicle maker saw a 20% boost in production speed and a 30% drop in errors with improved data annotation.
Drones and Aerial Imaging Technologies
Drones with aerial imaging technologies are vital for crop monitoring and health assessment. They rely on agricultural drone data annotation for high-resolution data, key for precision agriculture. For example, a startup cut pesticide use by 25%, improving crop health and yields.
Robotic Weeders and Planters
Robotic weeders and planters automate the tasks of weed control and crop planting. These robots, using computer vision, distinguish between crops and weeds for efficient operations. Advanced image annotation is vital for their development, ensuring tasks are performed with high accuracy. This efficiency reduces labor costs and optimizes resource use in agriculture.
The agriculture industry greatly benefits from detailed and precise data annotation, including image, video, and sensor data labeling. These tools are essential for tasks like crop type identification, disease detection, growth monitoring, and yield estimation. They highlight the significant impact of AI on agriculture.
Data Collection Techniques in Agriculture
Modern farming relies heavily on effective data collection techniques. Advanced sensor-driven farming and the Internet of Things (IoT) in agriculture make data acquisition seamless and accurate. This combination is essential for improving efficiency and precision in farming.
Sensor Technologies and Their Applications
Sensors are now a key part of farms, collecting important information on soil properties, environmental conditions, and crop health. These methods ensure precise data collection, leading to smarter agricultural management. Here are some key applications:
- Soil Sensors: Measure moisture, pH levels, and nutrient content, optimizing irrigation and fertilization processes.
- Climate Sensors: Track temperature, humidity, and precipitation to predict weather changes and protect crops.
- Crop Health Sensors: Monitor plant growth and detect diseases early, facilitating timely interventions.
These data points help farmers make informed decisions, leading to better crop yields and sustainable farming practices. Technologies like AI in aerial crop analytics and automated harvesting systems benefit from annotation platforms like Keylabs. These platforms enhance the precision of data collected by these sensors.
Internet of Things (IoT) in Agricultural Robotics
The integration of IoT in agriculture has transformed data flow between systems. IoT-enabled devices enable real-time monitoring and automated control of various agricultural processes. Some key advantages include:
- Real-Time Monitoring: Continuous data feed from multiple sensors provides a complete overview, enabling proactive management.
- Automated Operations: IoT integration synchronizes tasks like irrigation, harvesting, and pest control, reducing human labor and increasing accuracy.
- Enhanced Data Flow: Seamless data exchange between devices facilitates better decision-making and efficient farm management.
Incorporating agricultural technology annotation services, such as those provided by Keymakr, ensures the accurate training of AI models used in these IoT systems. These annotation services are critical for tasks like image segmentation and labeling, essential for autonomous tractors, drones, and robotic weeders.
Below is a table that outlines how sensor technologies and IoT integration enhance various aspects of agriculture:
Technology | Application | Benefits |
---|---|---|
Soil Sensors | Measure soil moisture, pH, and nutrients | Optimizes irrigation and fertilization |
Climate Sensors | Track environmental conditions | Predicts weather changes, protects crops |
Crop Health Sensors | Monitor plant growth and detect diseases | Facilitates timely interventions and boosts yield |
IoT Integration | Real-time monitoring and automated control | Enhances efficiency, accuracy, and data flow |
The Role of AI in Agricultural Robotics
AI's impact on agricultural robotics is profound, transforming farming through AI-driven crop management and machine learning. These technologies enable precise predictions of crop health and soil conditions. This is key to boosting efficiency and sustainability in agriculture.
Machine Learning Algorithms for Crop Analysis
Machine learning in agriculture uses complex algorithms to analyze vast datasets from farms. This analysis supports real-time crop health monitoring and early disease detection. AI-powered robots, for example, can distinguish between healthy and diseased plants, enabling timely interventions.
Automated weed control systems, like Blue River Technology's 'See & Spray', reduce herbicide use by up to 90%. This highlights AI's role in sustainable agriculture.
Using data annotation techniques like image segmentation is critical for training AI models. This precise data enables automation of tasks such as sorting, grading, and monitoring crop health. It expands the possibilities in farming.
Predictive Analytics in Agriculture
Predictive agriculture combines historical data with real-time inputs for future condition forecasts. AI algorithms analyze variables like weather, soil health, and crop yield to enhance forecasting accuracy. AI-assisted crop maturity prediction, for example, leads to more accurate and cost-effective management, boosting profits.
AI also aids in market demand analysis, guiding farmers on the best crops to grow and sell. This strategy increases profitability and reduces resource waste. AI's risk management tools detect defects, diseases, and quality issues early, ensuring a resilient and productive agricultural practice.
AI-driven crop management and predictive analytics are essential in modern agriculture. By continually improving these technologies, we are moving towards a more efficient, sustainable, and profitable farming future.
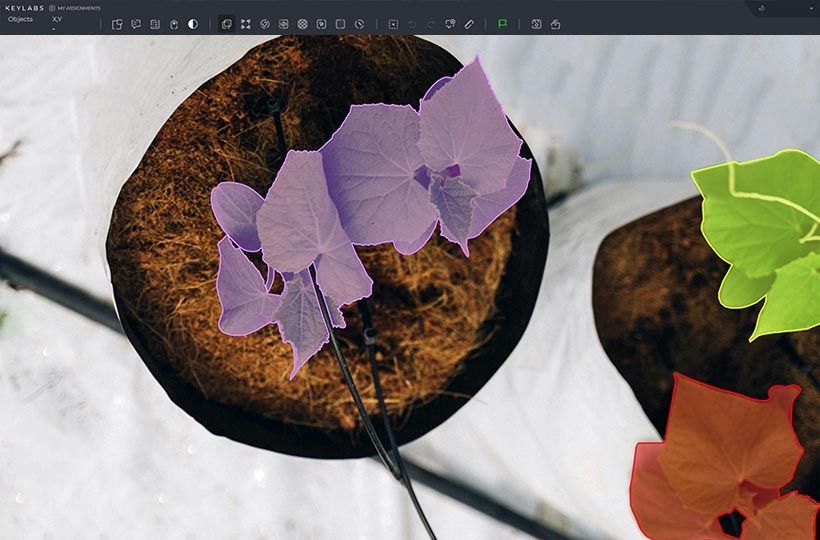
Data Annotation Techniques Used in AgTech
Data annotation is key to modernizing agriculture. We'll dive into manual and automated annotation techniques. These are vital for advanced image segmentation and labeling, leading to more precise farming.
Manual vs. Automated Annotation
Manual and automated annotation have their own strengths and weaknesses in agriculture:
- Manual Annotation: Human experts label data with high accuracy. Yet, it's labor-intensive, time-consuming, and expensive. Despite this, it ensures detailed and precise labeling, essential for tasks needing expert insights.
- Automated Annotation: AI-based systems label data quickly and efficiently. They need extensive training data to work well. Automated systems are scalable and fast, cutting labor costs and speeding up data processing.
Challenges in Agricultural Data Annotation
The field of agricultural data annotation faces numerous complexities. The variety in crops, growth stages, and environmental conditions makes achieving uniform and accurate data labeling a significant challenge. These obstacles are critical for improving AI's performance in agriculture, as any inconsistencies can affect the accuracy of AI models.
Variability in Crop Types and Conditions
The variability of agricultural environments poses a significant challenge. Different crops, growth stages, and environmental conditions like lighting and weather impact data consistency. For instance, fruit detection in AI applications is complicated due to varied harvesting conditions. This requires sophisticated annotation methods to handle such diversity.
Ensuring Accuracy and Consistency
High-quality annotated data is vital for consistent AI training, including tasks like 2D and 3D box annotation and semantic segmentation. These tasks are key for automated systems to identify ripe fruits in complex environments. Technologies like LiDAR scans and computer vision cameras help perceive and interact with the environment accurately. Continuous improvement of AI models and annotation processes is necessary to adapt to agricultural settings' dynamic nature.
AI models trained through accurate data annotation can predict crop yields, enabling farmers to make informed decisions about resource allocation and planning. This predictive capability is critical for efficient farming operations.
Area of Application | Impact |
---|---|
Autonomous Tractors | Optimized planting patterns, increased crop yields, reduced errors |
Drones for Crop Monitoring | Enhanced crop health tracking, optimized resource allocation |
Livestock Monitoring | Improved animal productivity, precise health management |
Crop Yield Prediction | Efficient resource planning, informed decision-making |
The successful AI implementation in various sectors of farming, from crop and livestock monitoring to yield prediction, showcases the profound impact of annotation on farming efficiency and sustainability.
Future Trends in Agricultural Robotics
The future of agricultural robotics looks bright, thanks to ongoing AI innovations in agriculture. These advancements will make robotic systems more efficient and effective. New AI algorithms are on the horizon, promising to improve decision-making and adaptability in agriculture.
Innovations in AI and Robotics Technology
Advancements in agricultural robotics focus on four key areas: CNN-based machine vision, autonomous mobility, electric drive, and affordable robotic arms. These technologies are essential for automating various farming tasks. They are used in dairy farming, fruit harvesting, organic farming, and more.
Deep learning-based image recognition is becoming more sophisticated. It goes beyond just identifying objects. It helps in localization, yield prediction, and disease detection. These advancements boost productivity and support sustainable farming by reducing chemical use and ensuring targeted actions.
- Autonomous robots are changing machinery designs to support swarm concepts, improving productivity.
- Speed, ruggedization, imaging, energy, load capacity, and vision technologies are driving agricultural robot evolution.
Predictions for Data Annotation Evolution
The evolution of data annotation is critical for AI-powered agricultural robotics to reach their full capacity. Semi-supervised learning is making it possible to create new datasets with less human input. This is seen in advancements in semantic segmentation and the development of open datasets for various environments.
Improvements in data annotation enhance the accuracy and efficiency of automated systems. This leads to more advanced, responsive, and sustainable farming practices. The aim is to meet the growing global population, expected to reach 9.8 billion by 2050.
Technology | Benefit | Application |
---|---|---|
CNN-based Machine Vision | Enhanced image recognition and diagnosis | Localization, Yield Prediction, Disease Detection |
Autonomous Mobility | Operational flexibility and efficiency | Autonomous Tractors, Field Robots |
Electric Drive and Powertrains | Eco-friendly and sustainable energy | Electric Farm Vehicles and Tools |
Affordable Robotic Arms | Cost-efficient automation | Plant-specific Actions, Harvesting |
The Role of Stakeholders in Agricultural Data Annotation
In agricultural technology, collaboration among various stakeholders is key to improving data annotation accuracy and efficiency. This teamwork brings together insights and resources from different fields. It drives innovation and tackles the real challenges farmers encounter.
Collaboration Between Farmers and Tech Companies
The partnership between farmers and tech companies is vital for creating effective AI solutions. Farmers offer real-world data and insights into crop dynamics and management. Tech companies provide AI tools that boost farm productivity and precision.
Importance of Research Institutions
Research institutions are critical in advancing agricultural research and ensuring data annotation techniques are valid and reliable. They explore new methods and provide the theoretical basis for AI innovations. By tackling data bias, they refine AI models.
Their work helps eliminate disparities, making AI benefits available to all farmers, regardless of farm size. This collaboration is essential for sustainable agricultural growth.
Conclusion: The Future of AgTech through Robotics and Annotation
The fusion of artificial intelligence (AI) and data annotation tools in agricultural robotics heralds a new farming era. This era is marked by enhanced efficiency, sustainability, and productivity. AI has transformed various sectors, now revolutionizing agriculture by improving crop management and operational efficiency.
Exploring the future of AgTech reveals several critical insights. The transformative effect of AI on traditional farming is profound. For example, AI-powered irrigation systems can cut water use by up to 50% while preserving crop yields. This is a significant leap toward sustainable farming, given agriculture's 24% share of global greenhouse gas emissions.
FAQ
What are the main benefits of using AI and annotation tools in agricultural robotics?
AI and annotation tools in agricultural robotics enhance crop management and efficiency. They enable data-driven decision-making. This leads to optimized planting, health monitoring, and harvesting, boosting productivity and sustainability.
How do robotic data labeling and machine learning in agriculture enhance farm operations?
Robotic data labeling and machine learning enable autonomous systems to adapt to various agricultural environments. They improve precision in tasks like planting, weeding, and monitoring crop health. This reduces labor costs and increases crop yields.
Why is data annotation important in agricultural AI applications?
Data annotation labels agricultural data from sensors and aerial images, providing context for AI models. Accurate annotations are essential for AI systems to interpret data correctly. This enhances predictive analytics, precision farming, and crop disease management.
What types of agricultural robotics are commonly used on modern farms?
Modern farms use autonomous tractors and harvesters for efficient harvesting. Drones and aerial imaging technologies monitor crops. Robotic weeders and planters ensure precision in planting and weed control.
How do sensor technologies and IoT contribute to modern farming?
Sensor technologies and IoT collect real-time data on environmental conditions, crop health, and soil properties. This facilitates immediate and informed decision-making. IoT integration enhances synchronized operations and data flow, boosting farming efficiencies.
What role does AI play in agricultural robotics?
AI transforms farming by analyzing data from operations. It helps understand crop health, predict yields, and detect issues. Predictive analytics enable preemptive decisions, improving crop management and reducing waste.
What are the differences between manual and automated annotation in agriculture?
Manual annotation is accurate but time-consuming and costly. Automated annotation uses AI for quicker labeling but requires high-quality training data. Both methods have unique applications and benefits in agricultural data processing.
What challenges do agricultural data annotation face?
Challenges include variability in crop types, growth stages, and environmental conditions. These affect data labeling consistency and accuracy. Overcoming these requires continuous refinement of AI models and annotation processes.
Can you provide examples of successful AI implementations in agriculture?
Successful implementations include AI-driven autonomous tractors optimizing planting patterns, increasing yields. Precise data annotation improves pest detection models, leading to effective pesticide application and better crop health.
What future trends are expected in agricultural robotics?
Future trends include advancements in AI algorithms for automation and more sophisticated robotic systems. Improvements in automated data annotation efficiency and accuracy are also expected. These aim to enhance sustainability, responsiveness, and productivity in agriculture.
How do stakeholders contribute to the development of agricultural data annotation and AI technologies?
Stakeholders like farmers, tech companies, and research institutions collaborate to drive innovation. They ensure practical applicability and address challenges in agriculture. Research institutions provide foundational knowledge for technologies developed by companies and used by farmers.
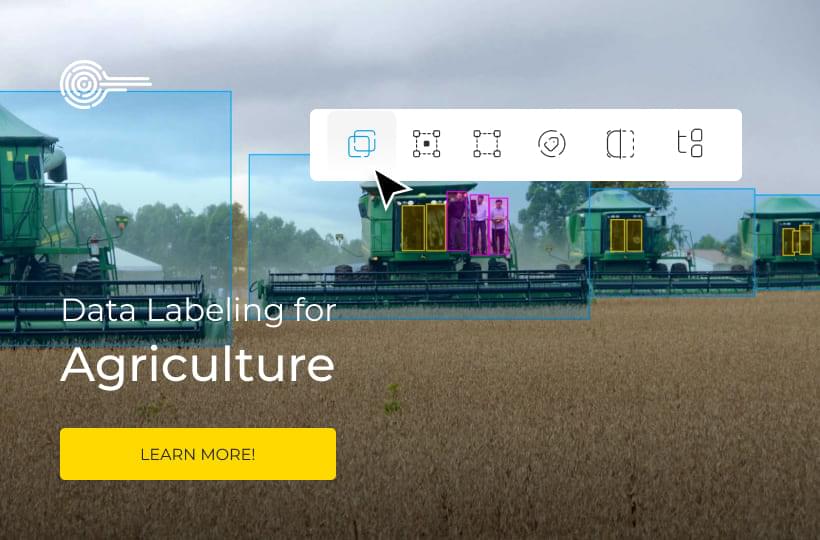