AI-Driven Video Annotation for Personalized Media Streaming and Content Recommendation
AI-driven video annotation empowers streaming platforms to offer personalized media streaming and content recommendations. These recommendations are based on viewer behavior and preferences. This improvement leads to more engaging and relevant video content, essential for keeping subscriptions and increasing viewership.
Tools like Keylabs provide advanced video content tagging capabilities. They enable creators and businesses to systematically optimize their visual projects. With AI, platforms can now analyze extensive media libraries. This allows them to curate content that perfectly matches user interests, significantly improving the user experience.
Key Takeaways
- AI-driven video annotation significantly improves video content quality and audience engagement.
- Personalized media streaming and content recommendation technology offer tailored viewing experiences.
- AI technologies allow for efficient analysis and optimization of vast media libraries.
- Enhanced personalized recommendations lead to increased subscription retention and viewership growth.

Introduction to Media Streaming Video Annotation
The advent of AI in multimedia processing has revolutionized our interaction with video content. Video annotation is key in media streaming, labeling essential elements in video frames. This includes characters, scenes, and actions. It aids in managing large video libraries and powers content recommendation engines, vital to streaming.
Current systems for video annotation only reach the first level of effectiveness. Yet, developing a language for video content representation is vital. It aims to integrate automatic, semi-automatic, and human annotation efforts. Video annotation extracts insights from video files by adding annotations, notes, labels, and tags to specific timestamps or segments.
The role of video annotation in streaming is immense. Over 2500 iconic primitives can be combined in the Director's Workshop for video annotation. Keywords are used to describe the complex temporal structures of video and audio information. Automation is suggested to accelerate the annotation process, with automated sending of videos to the next step or notifications in platforms like Slack or Teams.
Statistics show a significant increase in the number of annotations added over time, with a rise in replies. Participants mainly placed annotations 10 to 15 seconds after the relevant part of the video. Most found MRAS effective for taking shared notes on streaming video over the Web and aiding in class discussions. Clearly, video content's popularity and usefulness are growing across various industries and applications in the digital era.
Understanding the Basics of Video Annotation
Video annotation is the detailed process of labeling video content to make it understandable to AI models. It involves different techniques and technologies, each tailored for specific industries. This process is essential for making video content recognizable and accessible to AI systems.
Compared to image annotation, video annotation provides more data per unit. This is because it includes temporal context, which helps in understanding object movement. This is critical in fields like autonomous vehicles, where accurate video data is key for object identification and collision prevention.
There are several types of video annotation methods:
- Bounding Boxes
- Polygon Annotation
- Semantic Segmentation
- Key Point Annotation
- Landmark Annotation
- 3D Cuboid Annotation
- Rapid Annotation
Let's look at the main technologies used in video annotation:
Technology | Description | Applications |
---|---|---|
Machine Learning Video Tagging | Automated tagging of video content using ML models | Content filtering, Automatic metadata tagging |
Semantic Segmentation | Partitioning video frames into semantically meaningful segments | Compliance monitoring, Medical imaging |
Advanced technologies like machine learning and semantic segmentation make video annotation more efficient. They ensure data collection is accurate and reflects real-world scenarios. Industries such as smart traffic surveillance, medical imaging, and pose estimation benefit from these annotated datasets.
Challenges in video annotation include the time-consuming nature of labeling frames and handling large data volumes. Overcoming these involves setting clear objectives, preparing data, and choosing the right annotation methods. It also requires using expert annotators, implementing quality control, and ensuring data privacy.
By grasping the basics of video annotation and using advanced technologies, businesses can enhance AI model accuracy and efficiency. This leads to more innovative solutions across various sectors.
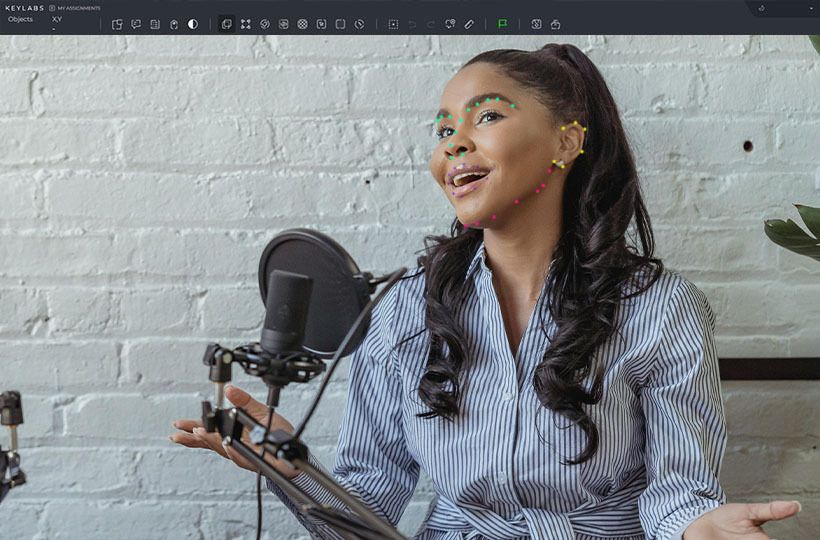
The Role of AI in Video Annotation
The integration of Artificial Intelligence (AI) in video annotation is a game-changer. It transforms how we understand and use video data. By using advanced Machine Learning Algorithms for Annotation, we can greatly improve the accuracy and speed of identifying and labeling video elements.
Natural Language Processing (NLP) takes AI to the next level. It extracts valuable descriptions from audio and text metadata. This technology enables us to automatically understand complex video content. It also generates annotations that are both precise and contextually relevant.
- Polygon Annotation: Essential for managing agriculture, monitoring plants, and using livestock AI systems.
- Lane Annotation: Helps car makers label roads, pipelines, and rails.
- Skeleton Annotation: Used in sports analytics, fitness tracking, and security.
- Cuboid Annotation: Offers precise 3D measurements for various video projects.
- Semantic Segmentation: Labels each pixel in an image, improving situational awareness.
- Instance Segmentation: Identifies specific objects in video frames.
AI's role in video annotation is significant across different sectors. In entertainment, AI tailors media recommendations by accurately tagging and categorizing content based on viewer tastes. The security sector uses Machine Learning Algorithms for Annotation to analyze surveillance footage. This enhances threat detection and response times.
AI also has a profound impact in retail, e-commerce, medical research, and autonomous systems. It automates object detection, facial recognition, and motion tracking. This boosts operational efficiency and decision-making in these fields. AI not only enhances video annotation accuracy but also scales the process. This supports a wide range of real-world applications.
In conclusion, the strategic use of Machine Learning Algorithms for Annotation and Natural Language Processing showcases AI's transformative role in video annotation. It leads to more effective and efficient use of video data across various industries.
Benefits of AI-Driven Video Annotation
In today's fast-paced technological world, AI-driven video annotation stands out as a symbol of efficiency and innovation. A key benefit is the Enhanced Personalization of Content. AI analyzes and labels vast video data, creating media experiences that match individual preferences. This boosts user engagement and keeps viewers coming back for more.
Traditional video annotation is slow and labor-intensive, requiring manual labeling of thousands of frames. This process is prone to errors and can lead to annotator fatigue. AI annotation, on the other hand, processes data consistently and accurately, reducing mistakes. This improvement enhances the performance of AI models.
AI video annotation saves time and money. It automates video segmentation, reducing the need for human effort. This allows media companies to focus on innovation and profitability. For big projects, crowdsourced video annotation offers a cost-effective solution.
AI video annotation shines in real-time applications. It can label data instantly, which is critical in security and autonomous systems. As AI models improve, they become better at detecting objects, tracking movements, and annotating scenes with minimal human help.
AI-assisted manual annotation combines human skill with machine learning, making the process faster and more accurate. Fully automated systems, powered by deep learning, can handle tasks from object detection to temporal annotation. This hybrid method ensures high accuracy and efficiency, making AI-driven video annotation essential in many industries.
Challenges in Implementing Video Annotation
The complexity of accurately labeling videos is a fundamental challenge. The current infrastructure often fails to meet the needs of advanced AI models. For instance, annotating videos frame by frame requires identifying and labeling objects over time. This task is complicated by the spatial and temporal sequence of data, even with human annotators.
The annotation process is also resource-intensive, requiring both time and expertise. Automated image and video annotation faces scalability and dynamic labeling challenges, necessitating human input for accurate results. Industry experts agree that video annotation is an AI-hard problem, involving multiple detection levels that machines cannot manage alone.
Privacy concerns are heightened when annotating videos with individuals. Adherence to data privacy laws like GDPR and CCPA is critical to prevent misuse or unauthorized access of personal information. Balancing annotation quality and speed is a challenging task. Integrating human verification steps can mitigate risks while ensuring accuracy.
The dynamic nature of data necessitates continuous retraining of models to maintain their reliability. Addressing the AI drift problem, where misclassifications may occur, is essential. This highlights the need for ongoing expert human supervision.
Implementing video annotation systems for applications like autonomous vehicles, security systems, and content recommendation is demanding. Yet, following best practices can help. Organizing data into manageable chunks and combining auto-annotation with human input efficiently addresses these challenges.
Ultimately, overcoming these hurdles ensures that annotated video data remains a cornerstone of AI development. It drives technological advancements while protecting data privacy.
For a deeper dive into the challenges of video annotation, visit Toloka's blog on the subject.
Future Trends in Media Streaming Video Annotation
The rise of user-generated content (UGC) on video platforms is undeniable. This trend demands more advanced video annotation tools to handle and utilize UGC efficiently. AI advancements are expected to improve annotation software's accuracy and functionality. Computer vision, a key AI field, allows computers to interpret visual inputs deeply, leading to more precise video annotations.
- Video production, media, and design companies employ these tools to detect unusual transitions, gather feedback, and identify problems.
- Legal departments depend on video annotation for redaction, like blurring faces or censoring sensitive footage for security.
- The retail sector uses video annotation to monitor buyer behavior and identify objects like price tags and store assistants.
- In healthcare, video annotation highlights specific parts of medical videos, aiding in diagnostic processes.
- Regulatory compliance in media ensures ads and video content meet mandated guidelines for digital or televised distribution.
The future of video annotation will witness further specialization, with tools tailored to specific needs. This includes:
- Status tracking and movement analysis for on-screen subjects
- Human pose description and perceived intent monitoring
- Object detection and recognition
AI's capability to dissect videos into frames, treat each frame as a static image, and annotate individually or continuously is driving these advancements. For instance, 2D and 3D bounding boxes are widely used for object identification and localization. Splines and lines annotation aid autonomous vehicles in identifying lanes.
The media and entertainment industry is forecasted to reach USD 2.9 trillion by 2026. This highlights the immense opportunity and the increasing significance of user-generated content in shaping the future of video annotation.
Best Practices for Video Annotation
Effective video annotation in modern media streaming requires precision, user engagement, and seamless integration. To achieve this, we must focus on several key video annotation best practices. These practices ensure accuracy in annotations. Here's a detailed look at optimizing this process:
Platforms like Keylabs are improving video annotation with advanced AI tools and quality checks. In the professional world, video annotation tools are essential for various projects. These include customer onboarding, marketing materials, internal communications, and AI model training. The demand for these tools is increasing, driven by the rise in video advertising and high-quality content from brands.
Ensuring accuracy in annotations goes beyond technology; user feedback is key. Platforms that use user insights in their algorithms improve precision and functionality. For example, Keylabs's features like object tracking and pose estimation cater to both simple and complex annotations.
To choose the best video annotation tool, consider factors like intended use, supported video types, project sizes, compatible devices, and pricing. Ease of use is essential for optimizing workflow and collaboration. Use free trials and demos to verify usability and meet project requirements.
FAQ
What is AI-driven video annotation and how does it impact personalized media streaming?
AI-driven video annotation uses artificial intelligence to label video elements. This boosts personalized media streaming. It recognizes viewer preferences, leading to tailored content recommendations. This improves user experience and engagement.
Why is video annotation important in media streaming?
Video annotation is vital for managing large video libraries. It powers content recommendation engines and ensures quality. It categorizes and tags content efficiently, improving discoverability and user satisfaction.
What is video annotation?
Video annotation labels and tags content for AI recognition. It identifies characters, scenes, and actions. This is key for content filtering, automatic tagging, and compliance monitoring.
How do machine learning algorithms contribute to video annotation?
Machine learning algorithms are essential in video annotation. They improve the accuracy and efficiency of content identification. Their ability to learn from data makes them indispensable in video processing.
What are the benefits of AI-driven video annotation?
AI-driven video annotation offers several benefits. It personalizes content, improves engagement, and is cost-efficient. It provides precise recommendations and reduces manual tagging time, boosting profitability for media companies.
What challenges are involved in implementing video annotation?
Implementing video annotation faces challenges like data privacy and technical limitations. Balancing quality with speed is also a challenge. Addressing these ensures accurate and efficient annotation without compromising sensitive information.
What are the future trends in media streaming video annotation?
Future trends include the rise of user-generated content and AI advancements. These will enhance annotation tools' accuracy and capabilities. This will lead to better content customization and viewer experiences.
What are the best practices for video annotation?
Best practices include maintaining high accuracy and using advanced AI tools. Incorporating user feedback and integrating with media platforms are also key. These ensure seamless content management and delivery.
What tools and technologies are available for video annotation?
Various annotation software and AI tools are available. They offer different functionalities, suited to specific streaming service needs. Key technologies include machine learning, semantic segmentation, and natural language processing.
What is the impact of AI-driven video annotation on media streaming?
AI-driven video annotation has a significant impact on media streaming. It promises personalized, efficient, and interactive content delivery. Innovations in this field will redefine viewer engagement and set new content quality standards.
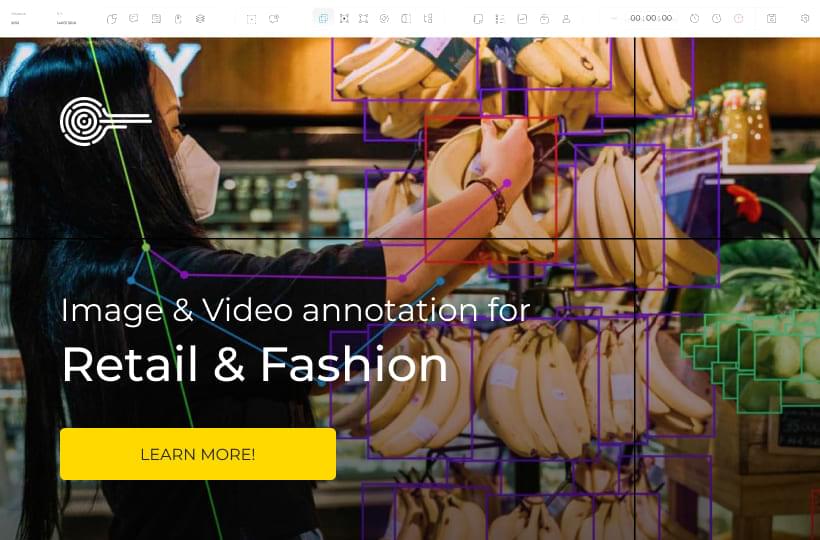