AI-Powered Data Annotation: Enhancing Quality Control in Manufacturing
Manufacturing image annotation has a great effect on companies in the industry. AI systems, driven by AI data annotation, play a key role in quality control. They enable predictive maintenance and optimize supply chains. By using machine learning for image annotation, manufacturers can improve quality standards while cutting costs and increasing efficiency.
Recent trends show AI's integration in manufacturing enhances product quality and gives companies a competitive edge. With the right data annotation, manufacturers can see major improvements in quality control automation. This ensures high-quality production outcomes.
Key Takeaways
- AI in manufacturing is projected to grow to $16 billion by 2025.
- Machine learning algorithms enhance operational efficiency and reduce costs.
- Quality control automation is significantly improved through AI data annotation.
- Predictive maintenance and supply chain optimization are key benefits of AI in manufacturing.
- Proper implementation of data annotation techniques can significantly boost product quality and competitiveness.

Understanding Manufacturing Image Annotation
Image annotation is the process of adding labels to visual data to make it understandable for AI models. In manufacturing, it's critical for machines to accurately identify and assess parts and processes. This enhances quality control significantly.
There are various types of annotations used:
- Image classification: Simple text labels, class numbers, or one-hot encodings categorize images by class.
- Object detection: Annotations include bounding boxes, defining extreme coordinates and class IDs.
- Image segmentation: Uses segment or binary masks to mark object segments by class ID.
- Semantic segmentation: Divides images into pixel regions, grouping similar objects under common categories.
- Instance segmentation: Focuses on separating and segmenting object instances from the image.
- Panoptic segmentation: Combines semantic and instance segmentation to segment both object categories and instances.
High-quality image annotations are essential for reliable machine learning models. These models provide accurate insights and perform well across various applications. For instance, in automated quality inspections, AI uses annotated data to detect and classify production errors with remarkable precision. This significantly reduces costly manufacturing errors.
Image annotation also plays a key role in monitoring infrastructure, identifying equipment failures, and improving maintenance practices in manufacturing. By leveraging annotated images, manufacturers can ensure their operations are both efficient and effective.
Application | Type of Annotation | Outcome |
---|---|---|
Quality Inspection | Object Detection | Detects and classifies errors with high precision |
Infrastructure Monitoring | Semantic Segmentation | Identifies equipment failures |
Maintenance Improvement | Instance Segmentation | Enhances maintenance practices |
Understanding what is image annotation and its strategic application in manufacturing is vital. It's essential for any organization aiming to optimize operational efficiencies and maintain stringent quality control standards.
The Role of AI in Image Annotation
Understanding how AI algorithms work is key to grasping their impact on image annotation across sectors like automotive and healthcare. AI algorithms analyze data through models trained on vast annotated image datasets. This training enables them to spot and learn from patterns. Such continuous learning enhances their ability to detect defects and anomalies in manufacturing.
AI in image annotation brings significant benefits, mainly in manufacturing. It greatly improves defect detection efficiency and accuracy. As AI algorithms learn from new data, manufacturing processes become more precise and reliable. This leads to a decrease in the need for human inspection, optimizing production cycles and cutting costs.
To grasp the advantages fully, it's helpful to look at key techniques and sectors benefiting from these advancements:
Techniques | Benefits | Industries |
---|---|---|
Bounding Box Annotation | Facilitates object detection and classification | Automotive, Retail, Healthcare |
Semantic Segmentation | Enhances image analysis and scene understanding | Manufacturing, Healthcare |
Landmark Annotation | Improves location precision | Autonomous Vehicles, Robotics |
3D Cuboids | Provides spatial understanding and depth | Automotive, Manufacturing |
Further, the algorithms used in supervised machine learning, like classification and regression, are critical in annotation. These methods ensure high-quality annotations, vital for AI model performance and training. Model validation, carried out by experienced annotators and engineers, ensures the reliability and accuracy of AI models. This validation confirms predictions and guarantees top-notch annotations.
Key Benefits of Image Annotation in Manufacturing
Image annotation brings significant advantages to the manufacturing sector. It enhances quality control, leading to better defect detection and higher product standards.
One key advantage is enhanced data accuracy. Advanced image annotation techniques precisely identify flaws, reducing human error. This precision is vital for maintaining high quality standards and ensuring components meet specifications.
AI technologies also enable real-time data processing, improving manufacturing quality. They quickly spot bottlenecks and allow for immediate corrective actions. This leads to smoother, faster production, essential for meeting demand and keeping schedules on track.
Image annotation also reduces waste and boosts customer satisfaction. By ensuring only defect-free items are sold, it enhances brand reputation and customer trust. This results in cost savings and efficiency gains.
Adopting Improved Quality Control Measures through image annotation tools can transform manufacturing. It offers operational efficiency and superior product quality.
Industry | Use Case | Benefit |
---|---|---|
Manufacturing | Defect Detection | Reducing Errors |
Autonomous Vehicles | Object Detection | Improved Navigation |
Surveillance | Face Identification | Enhanced Security |
Agriculture | Livestock Management | Increased Yield |
Common Types of Image Annotations
Understanding the different types of image annotations is key in manufacturing, where object detection is critical. Each annotation type meets specific needs, boosting production efficiency with AI. We explore the main annotation types used in manufacturing.
Image classification is a foundational annotation type. It labels entire images, making it easier to sort materials and components on production lines. This method uses AI to streamline production workflows by categorizing images efficiently.
Object detection focuses on identifying and outlining objects in images with bounding boxes. Accurate detection is vital for automated visual inspections, ensuring all elements are correctly tracked and managed. It's essential in areas like autonomous vehicles and surveillance, pinpointing objects' exact locations.
Semantic segmentation is another critical type, essential for detailed applications like automated defect detection. It divides an image into segments for a more detailed analysis of each component. Unlike basic segmentation, it labels pixels, providing detailed insights into each product part.
Annotation Type | Description | Applications |
---|---|---|
Image Classification | Assigns predefined labels to entire images | Sorting materials, quick categorization on production lines |
Object Detection | Identifies objects and delineates them within images using bounding boxes | Automated inspections, autonomous vehicles, surveillance |
Semantic Segmentation | Divides images into segmented regions with pixel-level labels | Automated defect detection, precise component analysis |
These image annotation types boost AI's ability to process and analyze visual data efficiently. From image classification and object detection to semantic segmentation, each offers unique benefits. They drive accuracy and excellence in manufacturing operations.
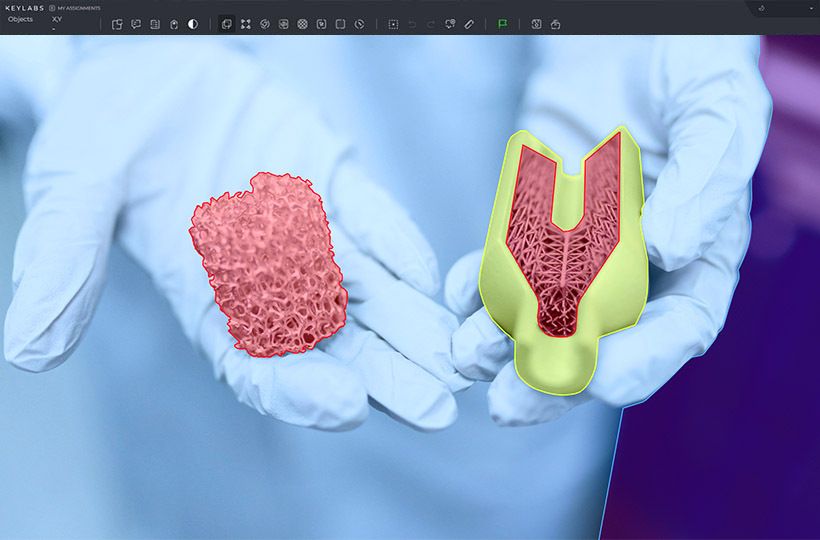
Training AI Models Using Annotated Data
High-quality annotated data is key for AI models to perform well, mainly in manufacturing. The process of dataset preparation starts with collecting images and annotating them accurately. This step is critical for the AI to learn from reliable data, leading to better results.
Key steps in dataset preparation for AI model training include:
- Image collection: Gathering diverse and high-resolution images representing various scenarios encountered in manufacturing environments.
- Data annotation: Using techniques like Image Bounding Boxes and Object Tagging to highlight and classify specific features within the images.
- Quality control: Verifying and refining annotations to eliminate errors and inconsistencies.
Alongside dataset preparation, using advanced model optimization techniques is vital for AI performance. These include algorithm tuning and validation. They help fine-tune the model's parameters and ensure it works well in real-world scenarios.
The table below compares different data annotation techniques used in training AI models:
Annotation Technique | Application | Benefits | Challenges |
---|---|---|---|
Image Bounding Boxes | Object detection and tracking | High precision in identifying objects | Time-consuming |
Object Tagging | Classification and categorization | Enhanced model understanding of different object classes | Potential for human error in tagging |
Image Classification | General categorization of images | Efficient for large datasets | Less detailed than bounding boxes |
Ensuring high-quality dataset preparation and leveraging effective model optimization techniques are essential strategies for achieving robust and reliable AI models in manufacturing.
Challenges in Image Annotation for Manufacturing
In manufacturing, the hurdles of dealing with large volumes of data are significant, mainly in image annotation. Deep learning algorithms need precise annotations to perform well. Without them, these models may falter, making data management and quality a top priority.
Ensuring annotation quality is a major challenge for companies. The sheer volume of data requires meticulous attention to detail. Inaccurate labels can severely impact AI performance and efficiency. To address this, companies must implement strict quality control measures, like clear guidelines and expert annotators.
Managing massive datasets also comes with a high cost and time investment. The need for substantial storage and processing capabilities is resource-intensive. Outsourcing can offer efficiency but raises concerns about data security and confidentiality. Pricing models for third-party services vary, with options like pay-as-you-go and subscription plans.
Despite these obstacles, accurate image annotation is vital. It provides essential context to AI systems, improving their functionality in areas like autonomous driving and quality control. Many companies are using image annotation to automate tasks, boosting efficiency and cutting costs.
Quality assurance is critical in manufacturing's complex environments. Companies often use multiple annotators and reconciliation methods to ensure data accuracy. Advanced tools like Keylabs streamline the annotation process. This, along with open communication and continuous improvement, maintains high data quality.
High-quality data annotation services from human experts can greatly enhance ML and AI in manufacturing. This leads to better analysis and predictive capabilities. The ongoing evolution of AI and machine learning demands a persistent effort to overcome these challenges and innovate in data annotation.
Future Trends in Manufacturing Image Annotation
The future of manufacturing image annotation is set to see major advancements thanks to emerging technologies. Predictive analytics and AI are evolving, set to change how we annotate data in manufacturing. This shift will be significant.
Automation in image annotation is a trend we're all looking forward to. Automated tools will handle large datasets efficiently, enabling real-time decision-making. This is key for areas like autonomous driving and surveillance. The move to automatic data labeling will boost efficiency and cut costs, benefiting the industry greatly.
Deep learning technologies, like CNNs and GANs, will also play a big role. They're great at spotting complex patterns, leading to more accurate annotations. Transfer learning is another trend; it shortens training times and reduces data needs, making advanced annotation tools more accessible.
Self-learning annotation tools will be key in the future. They get better with each use, perfect for dynamic manufacturing settings. 3D annotation techniques, using cuboids or voxels, will also grow in importance. This is true for sectors needing precise spatial info, like automotive and industrial automation.
Emerging technologies will also link image annotation with the Internet of Things (IoT). This will lead to big leaps in smart cities, healthcare, and industrial fields. The combination will boost operational capabilities and offer deeper insights into production, helping industries avoid problems before they start.
Data augmentation methods, like geometric transformations, will also grow. They'll expand training datasets, making deep learning models more robust. Techniques like AutoML and uncertainty estimation will make data annotation faster and more efficient.
The future of manufacturing image annotation looks very promising. It's driven by ongoing tech innovations and the increasing use of AI. As these trends develop, manufacturing will see huge improvements in efficiency, accuracy, and productivity.
Best Practices for Effective Image Annotation
Image annotation is key for developing strong AI models, essential in manufacturing and other sectors. To get the best results, we need to follow best practices that ensure our data is both high-quality and efficient. Workflow Optimization is central to this effort.
First, continuous monitoring and improvement are vital. Regular checks help spot and fix errors quickly, keeping our annotated data accurate and trustworthy. By using feedback loops and review stages, we can refine our annotations, making them more precise and effective.
To optimize workflows, using advanced annotation tools and methods is critical. A top-notch image annotation tool should handle a large number of objects and labels per image efficiently. This is essential for keeping the process both fast and productive.
Customization is another key practice, allowing us to adjust the label editor to fit our specific needs. This personalization boosts the speed and accuracy of image annotation, as users work in a framework that suits their tasks.
Model-assisted labeling also plays a significant role in reducing labeling time and costs. By having the model suggest labels, human annotators can focus on the tricky parts, making the process more efficient. This method speeds up data annotation without compromising quality.
"A streamlined user interface reduces the cognitive load on labelers, contributing to faster annotation speeds and better user experience."
For smooth integration, connecting data via Python SDK or API is beneficial. This makes transferring data into AI models like TensorFlow and PyTorch easier, improving Workflow Optimization and productivityy adopting these best practices for image annotation, we ensure high standards and optimize our workflows.
Measuring the Impact of Annotation
Evaluating the impact of data annotation in manufacturing requires focusing on specific key performance indicators (KPIs) for quality control. Metrics such as accuracy, consistency, completeness, and annotator agreement are essential. These KPIs not only measure the success of data annotation but also pinpoint areas for enhancement.
- Accuracy: It checks if annotations match the actual data, ensuring the dataset's reliability.
- Consistency: This metric evaluates how uniform and dependable annotations are among different annotators.
- Completeness: It assesses if all required information is annotated in the dataset.
- Annotator Agreement: Shows the degree of agreement among annotators, often measured with Cohen's kappa and Fleiss' kappa.
Implementing these metrics allows organizations to conduct a detailed ROI analysis. This analysis reveals the cost-effectiveness and benefits of AI investments. It guides future decisions and resource allocation, ensuring AI model quality continues to rise.
ROI Analysis Factors:
- Data Annotation Quality: Crucial for developing dependable AI models.
- Accuracy Improvement: Essential for creating more reliable AI outcomes, affecting areas like autonomous vehicles.
- Consistency in Data Annotation: Ensures uniform, high-quality labeled datasets.
- Prevention of Biased Models: Ensures accurate predictions by avoiding incomplete or incorrect annotations.
By using these KPIs for quality control, manufacturers can grasp the effectiveness of their annotation processes. This understanding enables them to make informed decisions, boosting the performance of their AI models.
The Future of Manufacturing Image Annotation
Looking ahead, AI-powered image annotation will play an increasingly vital role in the manufacturing sector. The automation of image annotation and the creation of advanced tools for annotating videos and 3D images will significantly boost efficiency and precision. This is critical for tasks like segment mask creation, where auto annotation tools and pre-trained algorithms are proving indispensable.
Annotated images are key in training supervised AI models, greatly impacting AI and machine learning quality. They enable models to identify and forecast objects, essential for defect detection, anomaly spotting, and product feature recognition. The trend of multi-modal data annotation, which includes text, audio, and sensor data, enhances AI's capabilities further.
FAQ
What is image annotation in manufacturing?
Image annotation in manufacturing is the process of adding labels to visual data. This makes it understandable for AI models. It helps machines accurately identify and assess parts and processes. This enhances quality control through advanced data annotation practices.
How do AI algorithms work in image annotation?
AI algorithms process data through models trained on large sets of annotated images. They identify patterns. By learning and adapting from new data, these algorithms improve efficiency and accuracy in detecting defects. This optimizes production cycles and reduces costs.
What are the key benefits of image annotation in manufacturing?
Image annotation improves quality control, enhances data accuracy, and speeds up production. It allows for precise defect detection, reduces human error, and ensures quality standards are met. This leads to better products and processes.
What common types of image annotations are used in manufacturing?
In manufacturing, common image annotations include object detection, semantic segmentation, and image classification. These techniques help identify and locate objects, divide images for detailed analysis, and categorize images for faster sorting. They are essential for efficient material and component management.
What are the steps for successful integration of image annotation in manufacturing?
Successful integration starts with understanding specific needs and choosing the right AI tools. Training staff on AI systems and planning and testing are key. This ensures AI integration improves quality and efficiency without disrupting workflows.
How is dataset preparation important for training AI models?
Dataset preparation is vital as it involves collecting and annotating high-quality images. Proper preparation enhances the AI's ability to generalize from training data to real-world applications. This maintains high accuracy and reliability of AI systems in manufacturing.
What challenges are associated with image annotation in manufacturing?
Challenges include managing large data volumes and ensuring high-quality annotations. Robust storage and processing are needed for extensive datasets. Quality assurance is essential to ensure AI models perform accurately without frequent errors.
What are the future trends in manufacturing image annotation?
Future trends include augmented reality and advanced predictive analytics. These technologies will enhance AI capabilities in manufacturing. They provide deeper insights into production processes and foresee issues, streamlining operations and increasing productivity.
What are the best practices for effective image annotation?
Effective practices include developing a streamlined workflow and ensuring annotation consistency. Continual monitoring and regular improvements based on feedback are key. This adapts to evolving manufacturing needs and enhances AI systems.
How can manufacturers measure the impact of annotation?
Manufacturers can measure impact using key performance indicators (KPIs) for quality control in AI applications. ROI analysis provides insights into the cost-effectiveness and benefits of AI investments. This guides future decisions and strategies for technology adoption.
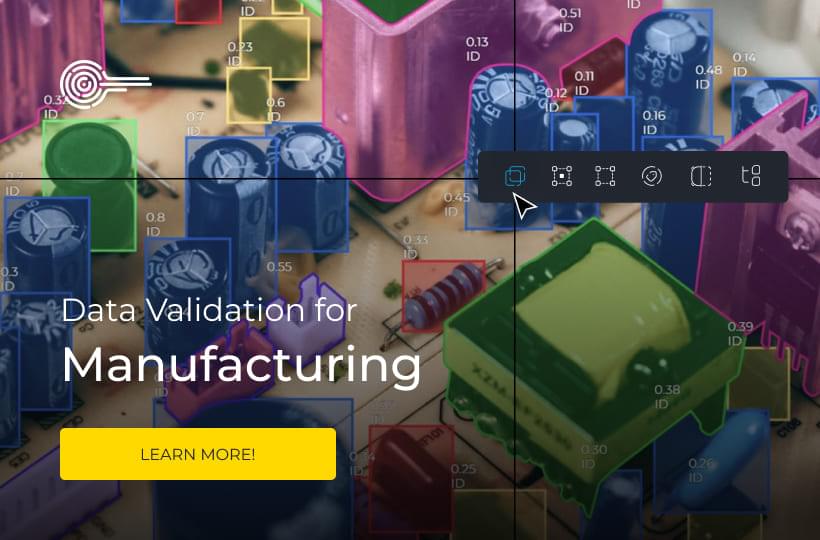