Annotating Agricultural Imagery: Improving Crop Monitoring and Yield Prediction
Thanks to the annotations of agricultural images, artificial intelligence models assess the condition of plants and predict yield. This develops the field of agriculture and increases work efficiency. Agricultural image annotation is the basis for training artificial intelligence models.
By combining these models with visualization methods, we can accurately diagnose plant health and predict the environment's impact on yield.
Key Takeaways
- Precision farming technologies reduce herbicide use.
- Annotated data trains artificial intelligence models to make agricultural decisions.
- Data annotation improves crop yield prediction.
- Machine learning models trained on annotated data improve crop health assessment.

Defining Agricultural Image Labeling
Agricultural image labeling is the process of annotating images of agricultural objects (fields, crops, animals, machinery). Thanks to this data, their analysis, machine learning, and automation of farming processes are carried out.
Importance in Modern Agriculture
Agricultural image annotation improves plant condition monitoring, optimizes farm resource management, and increases agricultural performance.
The agricultural sector gains growth monitoring, pest detection, and livestock management advantages. This leads to making the right decisions, which increases yields.
Technologies Used in Image Labeling
Integrating image recognition technology and computer vision in agriculture revolutionizes data processing and insights generation. This transformation drives the growth of precision agriculture, which is now applied in crop monitoring, land management, and yield prediction.
Overview of Image Processing Techniques
- RGB analysis helps to obtain information about the color of crops and soil for accurate condition assessment.
- Multispectral analysis investigates crop health and soil condition.
Keylabs offers accurate object identification through robust data annotation processes. These include crop analytics, automated crop and fruit harvesting, grading and sorting, plant pest and disease detection, and yield assessment.
Role of Artificial Intelligence
Agriculture AI automates the processing of large amounts of data, enhancing precision in farming analytics. Machine learning algorithms and neural networks improve image recognition accuracy, such as disease detection, seed counting, and field monitoring. Automatic annotation is practical for crops such as corn and cotton. This method solves the problem of increasing data volumes.
Applications in Crop Monitoring
Agricultural remote sensing uses drones and satellite imagery to collect images of crop fields.
Monitoring informs farmers about plant health, optimizes resource use, and increases yields.
Image annotation methods
Choosing annotation tools for agriculture. They should include different annotation methods, such as bounding boxes and polygons. Integrate well with existing systems and be easy to use. User-friendly interfaces help annotators learn and navigate quickly. This speeds up work and ensures correctly annotated data.
Staff training and onboarding
- Conduct workshops and refresher courses.
- Use e-learning modules and virtual simulations.
- Implement quality checks and controls.
- Set up feedback loops for annotators to share insights during the annotation process.
High-quality training improves the accuracy of data annotation and makes model predictions reliable in agriculture. Well-trained teams produce high-quality maps and improve decision support systems.
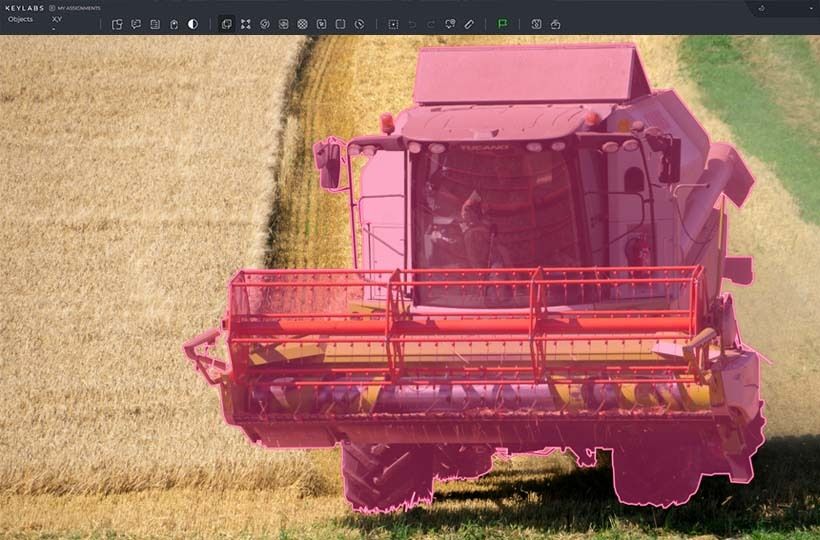
Benefits of Image Labeling for Farmers
Image labeling technology reduces the need for frequent on-site inspections and saves costs. It also ensures the economical use of resources such as water and fertilizer based on accurate annotated data. NAIR (National Agricultural Image Repository) has access to agricultural image repositories. This prevents technology monopolization and accelerates the development of agriculture. It improves resource management, making agriculture more efficient and sustainable.
Agricultural Imagery Labeling Challenges and Solutions
There is high variability of objects. Plants can look different depending on the variety, growth stage, climate, lighting, or health status. Weeds, diseases, and soil conditions vary, making it difficult to create universal models. This leads to inconsistencies that give incorrect predictions and reduce the reliability of crop monitoring.
Improvements in imaging technologies, such as hyperspectral and multispectral cameras, recognize objects regardless of external conditions. Artificial intelligence and machine learning algorithms are better at processing images and ensuring accurate data annotation.
Technical and Operational Barriers
High cost and labor intensity. Annotating agricultural images requires human resources, which is required for manual annotation. Agronomists who understand plant characteristics and can accurately label data are needed, which is expensive for small-scale farmers. Also, constant technical equipment updates are required, increasing the operational workload.
Optimizing advanced models requires resources and expertise often unavailable in agricultural settings. Semi-automated annotation tools reduce the time spent on manual labeling. Experts only review a small percentage of samples and correct inaccuracies rather than starting from scratch. Outsourcing annotation to regions with lower labor costs will be cheaper than labeling in countries with higher wages.
Future Trends in Agricultural Image Labeling
Combining IoT in agriculture with image labeling systems will advance data analytics and field management, ensuring data flow and analysis accuracy.
Changes in drone technology for agriculture are improving how agricultural imagery is captured and analyzed. High-resolution satellite imagery and advanced drones are reproducing detailed data. Combined with computer vision and AI, these technologies automate pest and weed detection tasks.
FAQ
What is agricultural image labeling?
Agricultural image labeling is the detailed annotation of imagery from satellites, drones, and cameras. It identifies plant health, crop type, and growth stages.
Why is agricultural image labeling important in agriculture?
To improve crop monitoring, yield prediction, and optimization of farming practices.
What technologies are used in agricultural image labeling?
Image processing techniques like RGB and multispectral analysis are employed. These are paired with AI technologies, including machine learning algorithms and neural networks.
How does AI contribute to agricultural image labeling?
AI automates the processing of vast agricultural data, providing real-time insights and predictive capabilities. Machine learning algorithms and neural networks enhance image annotation accuracy and AI model predictive capabilities.
What are the applications of image labeling in crop monitoring?
Image labeling is used in remote sensing and data acquisition for continuous crop health monitoring.
What are the practices for image annotation?
When choosing tools and software, consider their ease of use and integration capabilities. Also, annotator training and regular updates on technology and industry practices are necessary.
How does image labeling enhance yield prediction accuracy?
Advanced machine learning algorithms analyze historical data and current conditions in annotated images. They predict agricultural yields with higher accuracy. Research institutes have shown significant yield prediction improvements through image labeling.
What are the benefits of image labeling for farmers?
Image labeling reduces costs by minimizing physical field checks. It optimizes resource usage, such as water, fertilizers, and pesticides, through the precise application. It also improves decision-making with detailed, real-time insights into crop health and soil conditions.
What are the challenges in agricultural image labeling?
Data quality is a significant challenge due to image capture conditions and variability in plant appearances. Technical barriers include the high costs of advanced imaging equipment and AI systems. Operational challenges involve continuous updates and maintenance of annotation systems.
What are the future trends in agricultural image labeling?
Integrating IoT devices with image labeling systems improves data flow and analytical capabilities. Advancements in satellite and drone technologies are expected to enhance image resolution and frequency. This will further refine image labeling accuracy.
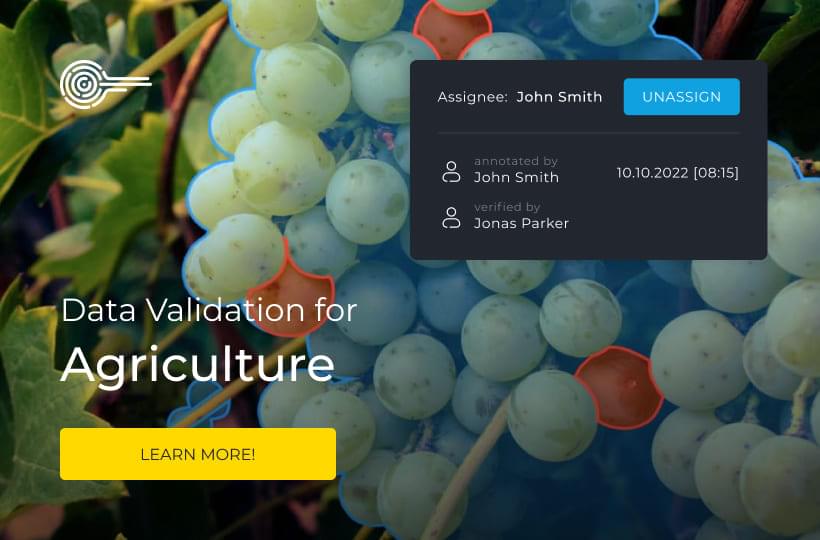