Annotation Tools Can Make the Difference for Autonomous Vehicle AI
Autonomous vehicles (AVs) can make our roads safer in addition to improving the overall efficiency of transportation and logistics. However, in order for AVs to become widely used they need to consistently prove their reliability and safety. This means that in each case, from vehicles from cars to buses, and in every location computer vision models need to perform flawlessly.
As a result AV AI models need to be trained with accurately annotated data. Data annotation means humans adding information to digital images and videos. Annotated video data is particularly important because it allows AVs to understand and operate in dynamic and complex real world environments.
Consequently, developers of AVs need access to large volumes of precisely annotated video data.
Firstly, this blog will look at why video annotation is particularly challenging. Secondly, we will identify the key AV capabilities that video annotation makes possible. And finally, we will show how the right video annotation tool can give AV developers flexibility whilst improving annotation quality and speed.
Video annotation challenges
Video annotation adds important contextual and semantic information to each frame of video training footage. Human workers use annotation tools to outline and label important objects, or segment pixels into defined classes, frame by frame. Even small pieces of training footage can feature thousands of individual frames.
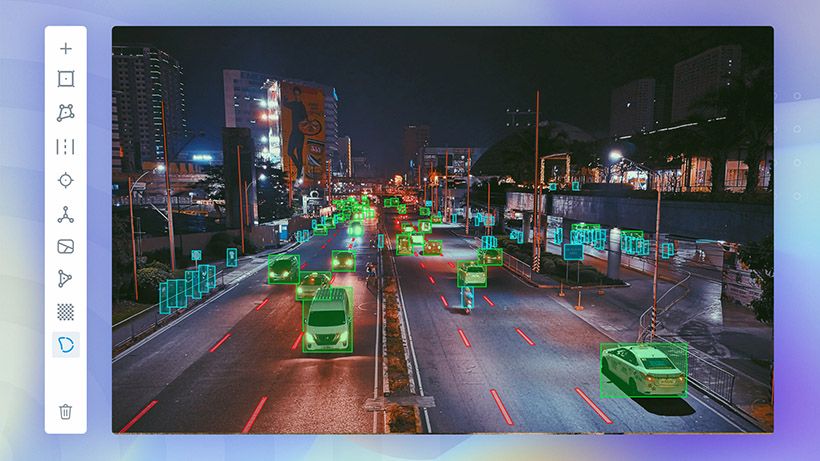
This makes video annotation extremely time consuming and labor intensive. In addition it means that video annotation is a demanding management task, involving potentially hundreds of individual annotators. Leading a large scale video annotation project can be a distraction for engineers and company leaders, who should be focusing on core development goals.
Self driving vehicle capabilities
Despite the challenges video annotation is still vital because it helps to represent the varied movements and interactions that happen on the road every day. Models that learn from annotated training data can successfully navigate busy streets and avoid any obstacle:
- Lane recognition: In order to be used safely on public roads automated vehicle models must be able to recognise lane markings and stay within them. To help them do this annotators locate and define the dimensions of lanes in training videos. These annotation lines sharply identify the shape of roads and lanes, allowing autonomous vehicles to stay within safe limits.
- Object classification: Semantic segmentation helps AV models learn by adding context and detail to video training data. Annotators use annotation tools to assign every pixel in each frame to a particular class, e.g. road, sidewalk, truck. This additional detail helps AVs to navigate safely and precisely.
- Object detection: Object detection allows AVs to correctly identify and move around different objects. Annotators use video annotation tools to place bounding boxes around objects. They then assign the objects a label e.g. car, bus, etc. Computer vision models are able to recognise and navigate around important road objects after being trained with video data annotated in this way.

Video annotation tool options
Video annotation can be difficult, but is vital for AV development. Keylabs is a data annotation tool designed to make video annotation faster and more precise:
- Project management: Keylabs hase unique project management and workforce analytics features. This allows managers to get a full picture of annotation performance and assign video annotation tasks based on performance and fit.
- Easy integration: Keylabs is designed for easy integration of any pre-annotation or custom written workflow automation code.
- Interpolation options: Keylabs makes video annotation faster with effective object interpolation. Algorithms are used to automatically track objects across multiple video frames, so that annotators only need to check and verify.