Applications of Image Classification in Different Industries
Global photo capture amounts to about 1.72 trillion a year. These images are crucial for training digital systems, especially in self-driving technologies. Here, computer vision and image recognition play a vital role. These systems, powered by artificial intelligence and deep learning, are reshaping industries, and various applications of image classification enable it. They do this by interpreting visuals to boost productivity and creativity.
AI image recognition software is revolutionizing sectors like healthcare, manufacturing, retail, and agriculture. For healthcare, it helps in spotting diseases early. In manufacturing, it guarantees product quality and sorts items. The security field uses these tools for face identification. Meanwhile, in retail, they refine stock control and the customer journey.
The success of AI image systems heavily relies on the quality and variety of their training datasets. Therefore, broad and detailed datasets are vital. Tasks like resizing images and eliminating noise also enhance the model's accuracy. Today, advanced methods such as convolutional neural networks have taken image classification beyond human-level skill in certain tasks. This demonstrates the remarkable real-world impact of these advances.
Key Takeaways
- Approximately 1.72 trillion photos are taken globally each year, aiding the training of image recognition technologies.
- AI image recognition is transforming sectors like healthcare, manufacturing, and retail by enhancing efficiency and innovation.
- Diverse and high-quality training data are crucial for the optimal performance of image classification models.
- State-of-the-art methods such as convolutional neural networks are instrumental in achieving high accuracy in image classification.
- Image classification has significant applications in security, agriculture, and autonomous driving systems.

Image Classification in the Retail Industry
The impact of image classification in retail is immense. Retailers now apply cutting-edge object detection and image recognition technology. This shift allows them to revolutionize their processes and elevate customer experiences. With AI-powered image recognition, retail analytics, data annotation, and customer behavior tracking reach new heights.
Improving Customer Experience
Within retail, image recognition technology is a game-changer for customer experience. Take Ulta, with virtual try-on tech, seeing a 700% brand engagement boost. Meanwhile, Adidas achieved a 36% cut in product returns using similar solutions. These AI-driven advancements aren't just boosting personalization. They're also analyzing customer behavior deeply. This leads to precise product recommendations, driving up customer satisfaction and loyalty.
Inventory Management
Top-notch inventory management is key in retail. Image recognition tech steps in, curbing stockouts, boosting accuracy, and cutting shrinkage. Through object detection, misplaced items or theft are swiftly identified, enhancing stock levels. These real-time systems also help prevent stockouts, saving huge revenue losses. The result? More sales and fewer chances missed.
Preventing Theft
AI image recognition is also a star in theft prevention, upping the game in retail security. AI cameras boast 99.9% accuracy in spotting counterfeit goods. This stands out, considering the $500 billion counterfeit market impact. Retailers quickly detect stolen or fake items, tightening loss prevention measures. Various data sets also address tech issues, improving theft system reliability.
Adopting advanced image recognition and object detection tech is turning the retail industry's future bright. This move promises superior customer interactions, streamlined inventory, and sharper theft preventive measures. By leveraging real-time analytics and tracking customer actions closely, retailers can secure their spot in a cutthroat market, driving growth and profits.
Enhancing Healthcare with Image Classification
Image classification technologies are transforming healthcare. They enhance early disease detection and diagnostic accuracy, while also reducing medical costs. This is achieved through the use of advanced tools like machine learning and neural networks in analyzing medical images.
Early Disease Detection
Image recognition applications bring a new dawn in spotting diseases early. They boost the accuracy and speed of diagnosing conditions ranging from tumors to heart issues. This advancement is particularly life-saving, allowing timely actions to be taken.
The heart of this innovation lies in the precision of convolutional neural networks. These networks excel at finding small irregularities in medical images. This ability facilitates early treatments, often preventing health deterioration.
Improving Diagnostic Accuracy
AI's role in diagnostic accuracy stands out remarkably. It lessens the impact of human error, especially in pinpointing issues like brain tumors and lung nodules. The involvement of deep learning models processes medical data fast, aiding in clinical decisions.
Reducing Healthcare Costs
Image classification and machine learning have a positive financial impact on healthcare. They optimize diagnostic and treatment pathways, decreasing the need for extra tests. This streamlined approach contributes to quicker patient care, reduced hospital crowding, and substantial savings.
Application | Benefit |
---|---|
Early Disease Detection | Improved accuracy, faster diagnosis of tumors |
Diagnostic Accuracy | Reduction in human error, enhanced disease detection |
Cost Reduction | Streamlined processes, reduced unnecessary tests |
The journey of AI in medicine is ongoing. And with continued advancement, its integration with image recognition stands as a key change-maker. It is set to redefine the precision and efficiency of healthcare worldwide.
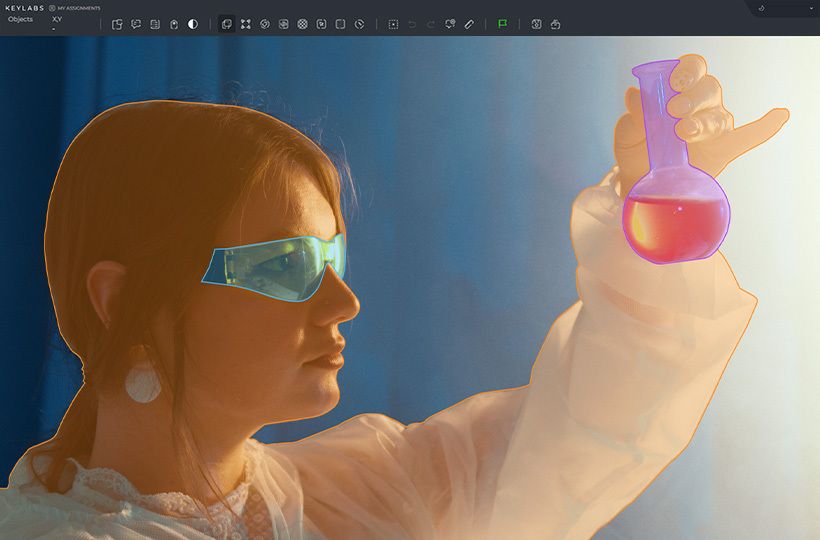
Applications of Image Classification in the Manufacturing Sector
Image classification is at the forefront of innovation in the manufacturing sector. Applying cutting-edge computer vision, it enhances quality control, identifies defects, and boosts production efficiency. These technologies leverage machine learning models and algorithms for precise and reliable results.
Quality Control
Quality control is paramount in ensuring products meet top standards. With the application of computer vision, error rates can drop significantly. McKinsey & Company reports show that automation can decrease errors by up to 90%. This is achieved through systems that use advanced image recognition to flag and classify defects, allowing only flawless items to reach consumers.
Defect Detection
Spotting defects is critical for upholding product quality within manufacturing. Machine learning algorithms quickly pinpoint imperfections, halting flawed products from advancing. This method boosts the accuracy of defect detection and cuts down on the inefficiencies and costs of manual checks.
Production Efficiency
The convergence of AI and image classification technologies significantly increases production efficiency. Real-time monitoring, feedback, and adjustments are possible, thanks to automated systems. Tractica predicts that by 2025, the use of image recognition in manufacturing will lead to annual savings of $27 billion. Such remarkable cost reductions highlight the advantage of deploying computer vision and machine learning to streamline operations and minimize downtime.
Aspect | Impact |
---|---|
Quality Control | Reduces error rates by up to 90% |
Defect Detection | Enhances product integrity and reduces labor costs |
Production Efficiency | Expected to save $27 billion annually by 2025 |
Security Industry Benefits from Image Classification
The marriage of computer vision with security has forever changed threat spotting and surveillance. Thanks to image recognition software driven by AI, sophisticated monitoring tools have become available, making it easier to spot unusual activities in real-time video feeds. This tech boost has drastically improved the performance of surveillance systems in both public and private domains.
Facial recognition technology stands out by giving an accurate picture of who's who. It not only enhances security by swiftly flagging potential dangers but also makes access control smoother through more dependable automated checks. The application of deep learning allows systems to sift through data, spot trends, and foresee outcomes, amping up the precision of image analysis.
A crucial role is played by algorithms such as Convolutional Neural Networks (CNNs), Support Vector Machines (SVMs), and K-Nearest Neighbors (KNN) in this area. CNNs' abilities in extracting features from images are especially lauded. SVMs and KNNs uphold critical roles too, employing varied classification techniques to improve how images are scrutinized.
AI’s entrance into security has also meant that many tasks can now be done without hands-on involvement, cutting down on errors and time. The real-time nature of this AI-powered image analysis answers the call for quick decision-making and insight. With an ever-increasing volume of visual data, these AI strides are pivotal in taming the vast amount of unstructured information, enhancing security overall.
AI in security has already made significant strides, even outperforming human accuracy in certain tasks. This underlines the potential for further threat detection improvements. Recent attention on Edge AI is changing the game, allowing machine learning to process data where it originates. This step forward leads to more privacy, speed, and better results, reinforcing today’s security methods.
Algorithm | Description | Application in Security |
---|---|---|
Convolutional Neural Networks (CNNs) | Extracts hierarchical features from input images through multiple layers. | Essential for real-time image recognition and facial identification. |
Support Vector Machines (SVMs) | Classifies images by identifying the hyperplane that best splits the data. | Used in analyzing surveillance feeds for threat detection. |
K-Nearest Neighbors (KNN) | Classifies based on the majority class of nearest data points. | Effective in identifying similar patterns of suspicious activity. |
The introduction of image recognition software into security goes beyond deterring intrusions; it's about evolving data analytics for betterment. Image classification has transformed surveillance systems, significantly improving the safety of our spaces. With ever-changing threats, the tech that defends us will also continue to progress, ensuring a future of fortified digital and physical security.
Automotive Industry Innovations
The automotive industry is changing rapidly thanks to AI image recognition and deep learning. These technologies are transforming vehicle manufacturing, improving safety, and enhancing customer experiences.
Vehicle Identification
AI image recognition is key in accurately identifying vehicles. Through deep learning, autonomous cars understand road signs, lane markings, and other cars. This improves their ability to navigate, crucial for the success of self-driving vehicles such as Uber's $7.25 billion fleet.
Safety Enhancements
Ensuring passenger safety is a top priority. Daily, 3,287 people die in car accidents worldwide, making automotive computer vision pivotal. These systems check part assembly, detect flaws, and maintain safety regulations. By spotting aesthetic defects, manufacturers mitigate risks, making vehicles safer.
Enhanced Customer Experience
AI-powered image recognition enhances the customer experience. It allows manufacturers to customize features based on each driver's preferences. The journey towards fully automated vehicles is marked by five levels of automation, a path being shaped by pioneers like Tesla and Apple. Elon Musk highlights the role of AI and cameras in advancing this technology.
Transforming Agriculture with Image Classification
The agriculture sector has seen a significant shift thanks to image classification technologies. These technologies use deep learning and computer vision to enhance crop management. They also help in improving farm productivity through innovative strategies.
Crop Yield Analysis
Deep learning models show a lot of promise in predicting crop yields. They rely on advanced machine learning algorithms for accurate estimates. These models use a thorough image preparation pipeline, including transformations and resize steps.
This process ensures the data is well-annotated. By analyzing vast datasets for different crop types, farmers get insights into potential yields. This helps in making informed decisions for precision agriculture.
Weed Detection
Agricultural computer vision is also crucial for weed detection. It allows for targeted crop management. Models typically consist of convolutional neural networks (CNN).
They include components like Conv2d layers, max pooling, and ReLU activation. These models are trained over multiple epochs to achieve high accuracy. For example, a weed detection model reached 94.6% accuracy, using fine-tuned ResNet50.
Weather Pattern Prediction
Accurate weather prediction is vital for crop management. Image classification techniques help in this, allowing farmers to anticipate weather changes. This strategy involves data visualization using Matplotlib to analyze weather datasets.
It supports the reduction of manual labor and aligns with precision agriculture. This is essential for managing crop growth and health effectively.
Algorithm/Model | Application | Accuracy |
---|---|---|
MobileNetV2 | Agricultural Product Classification | Up to 99.96% |
ResNet50 | Tomato Defect Detection | 94.6% |
VGG-16 | Plant Disease Recognition | 96.75% |
Today, agriculture is managed in a more data-driven way. As machine learning algorithms improve data analysis, they're increasingly applied in agriculture. This shows the growing role of technology in sustainable and effective farming.
Revolutionizing Transportation with Image Classification
Transportation is experiencing profound change thanks to image classification technologies. These systems, powered by machine learning and cutting-edge computer vision, promise huge leaps in both efficiency and safety.
Object and Person Identification
Identifying objects and people in traffic scenes is vital and image classification shines here. With the help of convolutional neural networks (CNNs), crucial features in images are extracted. This allows for precise object detection and person recognition, essential for traffic management and keeping pedestrians safe.
Improving Traffic Flow
AI and machine learning are transforming how we manage traffic. By processing data from security cameras and sensors in real-time, transportation systems can fine-tune traffic signals and handle congestion better. This leads to a smoother flow of vehicles and shorter travel times for everyone.
Traditional Methods | AI-Driven Methods |
---|---|
Lack scalability and efficiency | Handle large-scale analysis tasks |
Manual intervention needed | Automated image classification |
Limited real-time capabilities | Real-time traffic optimization |
Enhancing Security Measures
AI is significantly improving security in transportation. Equipped with security cameras and smart detection systems, authorities can keep watch for any signs of trouble. This preemptive strategy helps create a safer setting for everyone on the road.
In summary, image classification is revolutionizing how we manage traffic and enhance security in transportation. It's introducing smarter, more proactive systems. These developments underscore the vital part AI and CNNs play in reshaping the future of this sector.
Summary
The infusion of image classification into diverse sectors ushers in significant enhancements in operational fluidity and creativity. It spans industries from finances and retail to logistics, greatly improving the process of gathering data from documents. This shift simplifies tasks, boosts accuracy, and supports swift data analysis. These aspects are vital for informed decision-making based on data.
Deep learning, primarily through Convolutional Neural Networks (CNNs), is pivotal in identifying features and making predictions. Its use of structured grid data extracted from images significantly refines the ability to recognize objects. This makes these networks crucial in various fields. Complementary techniques like Support Vector Machines (SVM) and Decision Trees enhance the power of image analysis, expanding its utility.
Key software such as TensorFlow and Keras aids in the development and refining of CNN models for practical use. Additionally, the evolution of the YOLO series from YOLOv7 to YOLOv10 mark significant progress in both speed and precision. Keylabs helps created accurately annotated data for all these models, enabling their value.
FAQ
What are the primary applications of image classification in different industries?
Artificial intelligence and machine learning power image classification. It's found in multiple fields like retail, healthcare, and security. In retail, it tracks customer behavior for improved strategies. For healthcare, it's key in detecting diseases early, enhancing treatment accuracy. And in security, it helps spot threats efficiently.
How does image classification improve the customer experience in the retail industry?
In retail, image classification is a game-changer. It tracks customer behavior and uses AI to understand it. This leads to strategies that dramatically improve the customer experience. As a result, customer satisfaction and profits go up.
What role does image classification play in inventory management for retailers?
For retailers, image recognition simplifies inventory tasks. It automates shelf stocking, improving product availability. This boosts operational efficiency and cuts down on mistakes.
How does image classification help in preventing theft in retail stores?
AI image recognition fights theft in retail. It spots suspicious activities and theft attempts via video analysis. This technology is pivotal in cutting down on crime.
What advancements in healthcare have been achieved through image classification?
Healthcare has seen great strides thanks to image classification. Early disease detection has significantly improved. These improvements, paired with precise diagnostic tools, lead to better patient care and treatment outcomes. This is all due to the application of convolutional neural networks in medical images.
How does image classification contribute to reducing healthcare costs?
Image classification plays a big role in lowering healthcare costs. By making diagnoses more efficient, it promotes timely treatment. This reduces unnecessary resource use.
What are the primary applications of image classification in the manufacturing sector?
In manufacturing, image classification is used for quality control. It quickly spots defects in products, ensuring high quality. This cuts down on time and resources spent on flawed items.
How does image classification enhance security in public and private sectors?
In security, image recognition is a powerful tool. It aids in real-time threat assessment and surveillance. Its facial recognition and computer vision technologies are essential for monitoring and detecting abnormal behavior.
What innovations has image classification brought to the automotive industry?
For automobiles, image classification is crucial in developing autonomous vehicles. It identifies vehicles and enhances safety for occupants. Deep learning allows vehicles to interpret surroundings, making user experiences safer and more personalized.
How is image classification transforming agriculture?
In agriculture, image classification boosts crop yields and reduces manual labor. It's used for weed spotting and predicting weather patterns. This approach provides farmers with insights for better crop management and increased productivity.
What role does image classification play in the transportation industry?
In transport, image classification improves traffic management and security. It better identifies objects and people. This boosts safety and efficiency in transportation.
How does the marketing industry leverage image classification for better results?
Marketing uses image classification to understand consumer actions. It finds patterns and uses deep learning for precise marketing. With this, brands can create ads that truly resonate with their target audience.
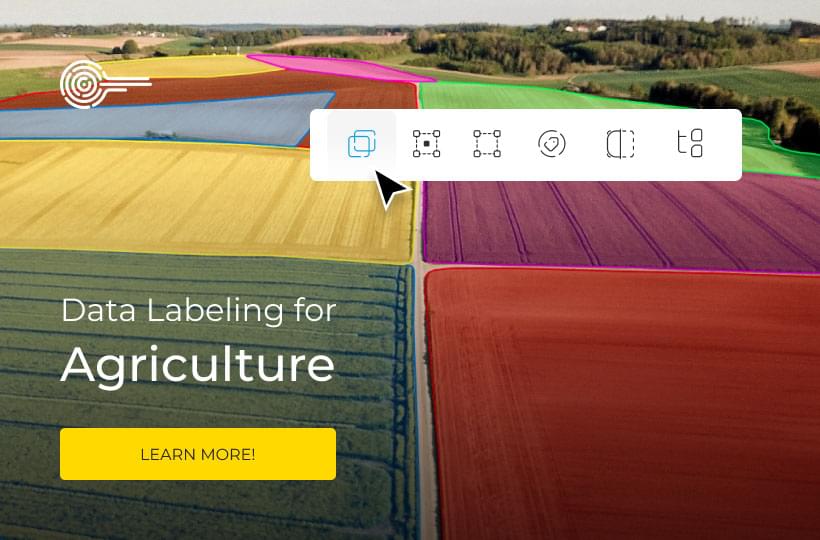