Automatic Annotation for Video Data
Automated video labeling can speed up video annotation by up to 90%. This saves businesses a significant amount of time and money. Video annotation is essential for machine learning, helping computer vision applications understand and interpret video data accurately. Yet, manually labeling videos is a daunting task, requiring a large team and being both time-consuming and costly.
Many businesses opt to outsource video annotation to cut costs, but this approach often doesn't expedite the process and can lead to quality issues. Automated video tagging addresses these problems, cutting down on manual effort, saving time and money, and enabling the labeling of larger datasets with consistent quality.
Thanks to AI advancements, tools like Keylabs are revolutionizing video labeling. These tools use advanced techniques such as multi-object tracking, interpolation, micro-models, and auto object segmentation. This results in high-quality annotations with minimal human effort.
Key Takeaways
- Automated video annotation significantly reduces time and costs compared to manual labeling
- AI-powered video annotation tools improve the consistency and quality of annotations
- Techniques like multi-object tracking, interpolation, and micro-models accelerate the video labeling process
- Auto object segmentation enhances the accuracy of object boundaries in video annotations
- Automated video annotation enables the processing of large datasets for machine learning applications

The Importance of Video Annotation in Machine Learning
Video annotation is vital in machine learning, especially in computer vision. As the need for smart video analysis increases, so does the demand for precise and efficient annotation methods. By marking and labeling video elements, algorithms can better grasp and interpret the content. This enhances a variety of applications and boosts model efficiency.
Enabling Computer Vision Applications
Accurate annotation is crucial for computer vision applications. It's used in several areas, including:
- Object detection and recognition
- Activity recognition and monitoring
- Facial recognition and emotion analysis
- Autonomous vehicle perception
- Surveillance and security systems
By offering labeled data, models learn to spot and identify objects, detect human actions, recognize faces, and understand complex scenes. This leads to the creation of smart systems that can automatically analyze videos and uncover valuable insights.
Improving Model Performance and Accuracy
Video annotation not only supports computer vision but also enhances machine learning model performance and accuracy. Annotated datasets allow models to learn from a broad, diverse set of examples. This enables them to apply their knowledge well in real situations.
machine learning for video understanding
The growing demand for video-based AI solutions highlights the critical role of video annotation in machine learning. It's essential for creating strong, precise models that can address real-world challenges and foster innovation across industries.
Automatic Annotation for Video: A Game-Changer
The advent of automatic video annotation has transformed machine learning and computer vision. AI-based algorithms and advanced machine learning techniques are now key to labeling and processing video data. This technology brings numerous benefits, including faster video labeling, enhanced accuracy, and substantial cost reductions.
Accelerating the Speed and Quality of Video Labeling
Automatic video annotation significantly speeds up the labeling process. Traditional methods are slow and require a lot of effort, often taking days for a single video. In contrast, automated annotation makes this process quick and efficient, processing large amounts of video data rapidly. This not only saves time but also ensures the quality and consistency of the labeled data.
Tools like Keylabs use advanced automation. They include active learning and model-assisted labeling to cut down on annotation work. These tools support various annotation types, ensuring precise and thorough labeling of videos.
Reducing Manual Inputs and Saving Costs
Automatic video annotation also reduces the need for manual work in labeling. Tasks like object detection, tracking, and segmentation are automated, lessening the workload for human annotators. This approach not only eases the workload but also leads to significant cost savings for companies handling large video datasets.
Traditional Manual Annotation | Automatic Video Annotation |
---|---|
Time-consuming and labor-intensive | Accelerated labeling process |
Reliance on labeler expertise | Improved accuracy and consistency |
High costs associated with labor | Significant cost savings through automation |
By adopting automatic video annotation, businesses and researchers can fully utilize their video data. This leads to faster and more efficient development of computer vision applications. With ongoing advancements in AI and machine learning, the future of video annotation looks promising, promising even more sophisticated and efficient solutions for video data processing and analysis.
Key Techniques for Automating Video Annotation
The demand for annotated video data is soaring, prompting researchers and developers to explore advanced automation techniques. These techniques, powered by cutting-edge algorithms and AI, are transforming the annotation process. They make it more efficient, accurate, and cost-effective.
Multi-Object Tracking (MOT) for Continuity
Multi-Object Tracking (MOT) is essential for automating video annotation. It ensures objects are consistently tracked and labeled across frames. MOT algorithms use sophisticated methods to maintain object continuity, even in challenging scenarios. This technique is crucial for applications like autonomous vehicles, where tracking multiple objects in real-time is vital for safe navigation.
Interpolation to Fill in the Gaps
Interpolation is a powerful technique for filling gaps between manually annotated keyframes. By using advanced interpolation algorithms, the system can automatically propagate labels to intermediate frames. This technique is invaluable for large video datasets, where manual annotation is impractical. It saves time and ensures smooth transitions between frames, resulting in accurate and consistent annotations.
Micro-Models for AI-Assisted Labeling
Micro-models are small, specialized AI models that assist in the labeling process. They can be quickly trained on a small subset of manually annotated frames and then applied to the rest of the video. This technique is particularly useful when dealing with domain-specific objects or scenarios. Micro-models adapt to the specific characteristics of the video data, enabling more accurate and efficient annotations.
Auto Object Segmentation for Improved Quality
Auto object segmentation automatically aligns object outlines with their actual contours in video frames. This technique uses advanced image segmentation algorithms to improve the quality of object annotations. It reduces manual effort required to refine object boundaries, allowing annotators to focus on higher-level tasks. This technique is crucial in applications where precise object boundaries are essential, such as medical imaging or industrial inspection.
Technique | Key Benefits | Suitable Applications |
---|---|---|
Multi-Object Tracking | Ensures object continuity across frames, reduces manual effort | Autonomous vehicles, surveillance, sports analytics |
Interpolation | Fills in gaps between keyframes, saves time, ensures smooth transitions | Large video datasets, animation, video editing |
Micro-Models | Enables AI-assisted labeling, adapts to specific video characteristics | Domain-specific applications, limited training data |
Auto Object Segmentation | Improves quality of object annotations, reduces manual refinement | Medical imaging, industrial inspection, precision-critical applications |
By combining these techniques, researchers and developers can create powerful automated video annotation pipelines. These pipelines significantly reduce the time and cost associated with manual labeling. As these techniques evolve, we can expect more advanced and efficient methods for annotating video data. This will enable the development of cutting-edge computer vision applications across various industries.
Automated video annotation is not just about speed and efficiency; it's about unlocking the full potential of video data and enabling breakthrough innovations in computer vision and AI.
Choosing the Right Video Annotation Tool
When selecting a video annotation tool, focus on the annotation tool features that meet your needs. Ensure the tool supports various annotation types, including bounding boxes and semantic segmentation. Automation features like object tracking can significantly boost efficiency and save time.
Essential Features to Look For
Other key factors to consider include:
- Compatibility with different video formats
- User-friendly interface and intuitive workflow
- Collaboration features for seamless teamwork
- Customizable annotation options and templates
- Integration with machine learning frameworks and APIs
By evaluating these features, you can find a tool that meets your project's needs and streamlines your video annotation process. Keylabs provides enterprise-level features for teams to quickly and efficiently take on complex workflows.
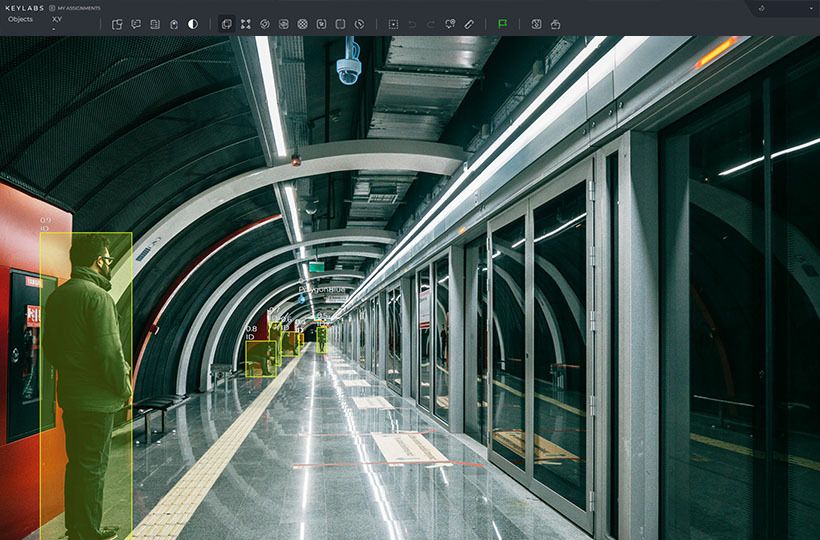
Implementing Automated Video Annotation in Your Workflow
Integrating automated video annotation into your workflow demands meticulous planning and execution. This ensures a seamless transition and maximizes the automation benefits. By adhering to best practices and focusing on data quality, you can effectively implement automated video annotation. This will streamline your annotation process.
Best Practices for Successful Integration
To integrate automated video annotation successfully, consider these best practices:
- Define clear annotation guidelines and standards for consistency across your team.
- Select a video annotation tool that meets your project's specific needs and supports automation.
- Provide thorough training to your annotation team on the chosen tool and guidelines.
- Initiate a small pilot project to test the automated annotation workflow and refine it before expansion.
- Continuously monitor the automated annotation system's performance and offer feedback to enhance its accuracy.
Ensuring Data Quality and Consistency
High data quality and consistency are vital for the success of automated video annotation. Implement these measures for data integrity:
- Implement quality control checkpoints throughout the annotation process to swiftly identify and rectify issues.
- Perform regular audits and spot checks on annotated videos to confirm accuracy and guideline adherence.
- Employ validation tools and techniques, such as cross-validation and consensus scoring, to evaluate annotation reliability.
- Offer ongoing feedback and training to your annotation team to uphold quality standards and bridge understanding gaps.
Real-World Applications of Automatic Video Annotation
Automatic video annotation has become crucial in many real-world applications, changing how we handle and analyze video data. It's essential in enhancing surveillance and security, transforming healthcare, and aiding in medical imaging. Let's delve into the areas where this technology is significantly impacting.
Surveillance and Security
In surveillance and security, video annotation applications are vital for spotting and tracking important objects. They use advanced computer vision to automatically identify and monitor suspicious people, cars, or actions in real-time. This helps security teams react quickly to threats, ensuring safety.
Healthcare and Medical Imaging
The healthcare sector has seen a major shift with automated video annotation. Doctors can now review surgical videos and medical imaging with much greater precision and speed. AI tools for labeling videos can annotate data up to 10 times faster, speeding up diagnosis and treatment plans.
Top healthcare and medical companies use advanced tools like those from Supervisely. These tools combine cutting-edge models like MixFormer and interactive segmentation models. This simplifies annotation and cuts down manual labeling work.
Autonomous Vehicles and Robotics
Automatic video annotation is revolutionizing autonomous vehicles and robotics. It's crucial for training models that let these systems detect obstacles and navigate safely. Techniques like multi-object tracking and auto segmentation help create strong datasets for training.
Robotics also gains a lot from automated video annotation. Researchers can label motion capture data with actions like running and waving. This helps build smart robots that can do various tasks.
Application Domain | Key Benefits |
---|---|
Surveillance and Security | Real-time object detection and tracking |
Healthcare and Medical Imaging | Faster diagnosis and treatment planning |
Autonomous Vehicles | Accurate perception models for safe navigation |
Robotics | Development of intelligent robotic systems |
The need for high-quality video data is growing across industries, making automatic video annotation vital. By using these technologies, companies can improve their processes, innovate, and streamline their workflows.
Advancements in Deep Learning Techniques
Deep learning models, such as convolutional neural networks (CNNs) and recurrent neural networks (RNNs), are becoming increasingly sophisticated. These advancements promise to cut manual annotation time in half while boosting accuracy.
Key advancements in deep learning for video annotation include:
- Improved object detection and tracking
- Enhanced semantic segmentation
- Advanced temporal modeling for understanding video context
- Transfer learning for adapting pre-trained models to new domains
Integration with Cloud Computing and Big Data
Video annotation tools are increasingly being integrated with cloud computing platforms. This trend offers scalable storage, processing, and collaboration capabilities, making it easier to manage large video datasets. Cloud-based solutions enable teams to collaborate seamlessly, regardless of their location, and access resources on-demand.
Big data analytics can further enhance the insights from annotated video datasets. By analyzing patterns, trends, and anomalies, organizations can uncover valuable insights. These insights drive innovation in domains like autonomous vehicles, healthcare, and retail.
The following table highlights the benefits of integrating cloud computing and big data with video annotation:
Benefit | Description |
---|---|
Scalability | Cloud platforms enable seamless scaling of storage and processing resources to handle growing volumes of video data. |
Collaboration | Cloud-based annotation tools allow teams to collaborate in real-time, regardless of their location. |
Cost-efficiency | Pay-as-you-go pricing models offered by cloud providers help optimize costs based on actual usage. |
Advanced Analytics | Big data analytics techniques can uncover valuable insights from annotated video datasets, driving innovation and decision-making. |
Summary
Automatic video annotation is revolutionizing machine learning workflows, offering significant benefits over manual methods. It speeds up the process of video labeling, reduces manual effort, and lowers costs. This automation enables businesses to efficiently handle large volumes of video data. Techniques like multi-object tracking and micro-models ensure accurate and consistent annotations, enhancing video analysis capabilities.
The future of automatic video annotation looks bright, driven by advancements in deep learning and the integration of cloud computing and big data. These trends will fuel innovation across fields like surveillance, healthcare, and autonomous vehicles. By adopting automated video annotation, companies can fully leverage their video data, boosting machine learning workflows and staying competitive. Automatic video annotation will be crucial in shaping the future of AI and machine learning.
FAQ
What is automatic annotation for video?
Automatic annotation for video leverages AI and machine learning to label and tag video elements automatically. This process speeds up video labeling, enhances quality, and cuts down manual input needs.
Why is video annotation important in machine learning?
Video annotation is crucial for machine learning as it supports computer vision applications. It boosts the performance and accuracy of models trained on video data.
What are the challenges of manual video annotation?
Manual video annotation is fraught with challenges. It's time-consuming and requires a large team. Ensuring consistency and quality across annotators is hard, especially with large datasets. Scaling annotation for extensive videos is a major challenge.
What are the benefits of automated video annotation?
Automated video annotation cuts down the time and effort needed for labeling. It ensures consistency and quality, supports scalability for large video collections, and frees up human resources for complex tasks.
What are the key techniques used in automating video annotation?
Key techniques for automating video annotation include Multi-Object Tracking (MOT) for continuity, interpolation to fill gaps, micro-models for AI-assisted labeling, and auto object segmentation for better quality.
What features should you look for in a video annotation tool?
When selecting a video annotation tool, focus on features like support for various annotation types, automation capabilities (object tracking, interpolation, AI-assisted labeling), collaboration tools, user-friendliness, and compatibility with different video formats.
How can you successfully implement automated video annotation in your workflow?
To implement automated video annotation well, set clear annotation guidelines. Choose the right tool for your needs. Train your team properly, establish quality control, and regularly check and give feedback to quickly address any issues.
What are some real-world applications of automatic video annotation?
Automatic video annotation is used in real-world scenarios like surveillance, healthcare, and autonomous vehicles. It's crucial for these applications.
What are the future trends in automatic video annotation?
The future of automatic video annotation is bright, with advancements in deep learning and cloud computing. These will lead to more accurate and efficient video annotation, driving innovation across various fields.
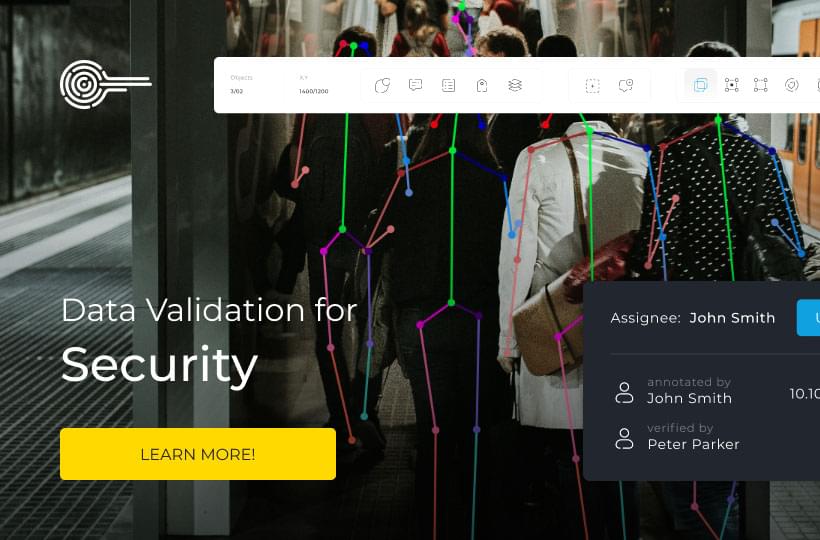