Automotive: Overcome the Toughest Data Challenges With Our Powerful Annotation Tool
Keylabs is an image and video annotation tool with a track record of producing high-quality datasets that help automotive AI developers tackle the biggest problems. Computer vision is transforming the automotive industry and making a new generation of autonomous vehicles a reality. This technology has the potential to make road travel safer and more efficient.
Automotive AI models must be able to interpret and navigate the world around them with absolute precision. In a sector where driver safety is key it is vital that computer vision based systems are reliable 100% of the time. Accurately annotated image and video training data is an important piece of the puzzle when it comes to developing the highest performing autonomous vehicle models.

Autonomous vehicles
Annotated digital images and video form the basis of datasets that train AI models to understand the complexity of busy streets and roads. Annotators use tools like Keylabs to fill images and video frames with information. This information enables autonomous vehicles to understand a chaotic world and move safely around potential obstacles. The following capabilities rely on specific annotation techniques:
- Object detection: Object detection allows autonomous vehicles to identify and respond to specific objects. During video annotation bounding boxes are placed around objects, which are then assigned a label e.g. car, bus, etc. Through exposure to training data annotated in this way computer vision models are able to recognize and navigate around important road objects.
- Object classification: Semantic segmentation helps to contextualize and add detail to video training data. Annotators use annotation tools to assign every pixel in each frame to a particular class, e.g. road, sidewalk, sky. This additional granularity helps self-driving vehicles to travel with precision.
- Lane recognition: In order to be deployed safely on public roads automated vehicle models must be able to recognize lane markings and stay within them. To achieve this video training data is annotated with polylines. These lines can define the parallel shapes of lanes, allowing autonomous vehicles to stay within safe limits.
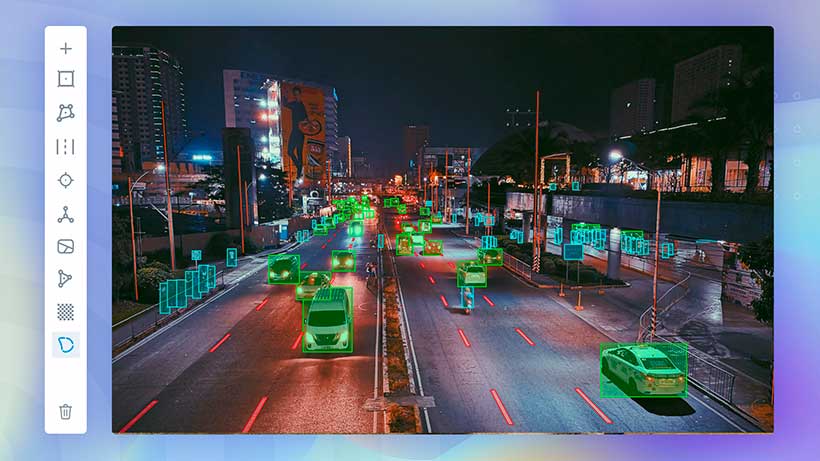
Varied datasets
Precisely annotated image and video data can lead to higher performing AI models. However, it can be difficult to create datasets that reflect the wide variety of conditions that are present in the real world.
If automotive AI models are trained with data that is biased in some way then self-driving vehicles may not function well when faced with diverse road environments. It is vital for developers to have access to varied, unbiased data in order for autonomous vehicles to gain the trust of the buying public.
Keylabs case study
A Keylabs client in the automotive AI sector needed training data for a lane recognition use case. Keylabs was able to help the client build a dataset that featured varied and accurately annotated video and image data. Keylabs was used to overcome bias challenges in a number of specific ways:
- Lighting and weather conditions: Drivers often need to travel in low light and in poor weather conditions. Therefore, if autonomous vehicles are only trained with images and video taken during the day in high visibility conditions they may be less functional in “non-ideal” scenarios.
The client required annotated data of road lanes in different weather conditions from thick fog to heavy snow. Keylabs’ quick outlining functions allowed road shapes and line markings to be efficiently captured by the annotation team, accelerating the pace of dataset construction.
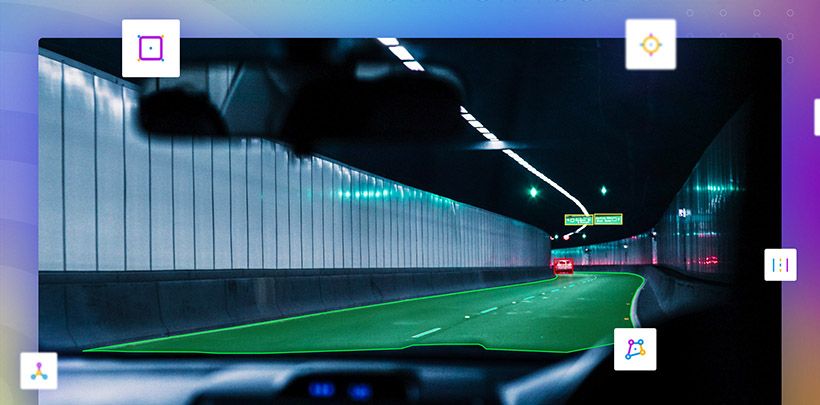
- Image and video quality: The quality of training images and video can significantly impact autonomous vehicles. In order to perform with the highest degree of precision autonomous vehicle AIs need to be trained with high definition images and video that reflect the full complexity of the real world.
Keylabs features object interpolation options that allow annotators to quickly track objects in video training data. - Different road markings and signage: Different countries use different road signage and markings to indicate rules like speed limits and driving conventions. Effective AI training data should contain images and video of diverse road signage so that autonomous vehicles can drive safely wherever they are.
The client needed training data for lane recognition that reflected road markings in a range of countries. Keylabs can support the labeling of varied datasets thanks to its unique project management options. Keylabs gives managers a high level overview of annotation performance and allows jobs to be assigned to workers with a proven track record of precision. - Diverse road systems: Of course road conditions vary significantly between regions. Overtaking conventions, side of the road used, and general driving etiquette can be wildly different from one country to another. Good training data should take these differences into account.
The Keylabs client needed training data that allowed their lane recognition model to function in different driving ecosystems. Keylabs allows developers to annotate video data for autonomous vehicles efficiently thanks to video annotation sharing features. Multiple annotators can work on a piece of digital video footage simultaneously, and their results can be seamlessly merged.