Benefits of Automatic Annotation for AI Projects
Delving into computer vision projects reveals an interesting fact: automation can slash up to 80% of the total time needed. A quarter of that time is just for data annotation. The impact of Automatic Annotation for AI Projects is profound. It speeds up development and maintains data quality, vital for building advanced AI systems.
Automatic annotation isn't just a time-saver; it enhances precision. With it, AI teams can work more efficiently and make better models. This approach quickens the annotation process, which leads to faster model training. It also helps with testing theories, tackling bias issues, and generally improving the AI model. Auto-labeling makes the machine learning workflow consistent and less reliant on manual tasks.
When we talk about avoiding manual work, automatic data annotation comes to the rescue. It spares people hours and resources. Developing an annotation tool from scratch could take years, costing millions. But, ready-made solutions are there, reducing the setup time to mere hours. The latest in automated labeling is the top choice for data annotation, speeding up the process and ensuring it can handle lots of data without trouble.
Key Takeaways
- Automation can save up to 80% of the time allocated for computer vision projects.
- Building an in-house annotation tool can be costly and time-consuming, whereas buying a solution can lead to quicker implementation.
- Automatic annotation reduces the need for extensive manual efforts and minimizes errors, ensuring a streamlined machine learning process.
- Auto-labeling facilitates quicker model training, theory testing, and overall model improvements.
- Technology advancements make automatic annotation the gold standard in the data annotation market, boosting efficiency and scalability.

Introduction to Automatic Annotation
The data labeling domain is transforming rapidly, thanks to auto-labeling techniques. These methods use machine learning to automatically add labels to data. They cut the need for manual processes, improving both speed and accuracy in data analysis.
Definition of Automatic Annotation
Automatic annotation encompasses using advanced algorithms to label datasets without human input. Labels are generated by algorithms, reducing the need for manual work. This technique ensures labels are applied consistently and accurately, thanks to the tools provided by annotation platforms.
How Automatic Annotation Works
Integrating ML models into the labelling process speeds up data preparation. By utilizing these models, the time taken for manual annotation decreases significantly. For example, Keymakr’s projects show significant time and cost savings during data preparation.
Automated annotation lessens the time needed to make a model ready for production. But, creating an in-house tool for this can be both costly and time-consuming, taking between six months to a year and a half. On the other hand, using available annotation platforms can offer a more cost-effective and scalable alternative.
Advantages | Statistics |
---|---|
Time Efficiency | 80% of project time; 25% on annotation |
Cost Reduction | 6-7 figure savings |
Faster Turnaround | Significantly reduced preparation time |
Scalability | Manage large volumes of data efficiently |
Automatic annotation is becoming a standard in the industry, with accuracy as a focal point. Despite the powerful auto-labeling tools offered by annotation platforms, integrating manual checks is crucial. Keymakr, for example, uses a blend of auto tools and expert annotators to ensure data labeling accuracy. This mix of automation and human skill is key to creating dependable AI models.
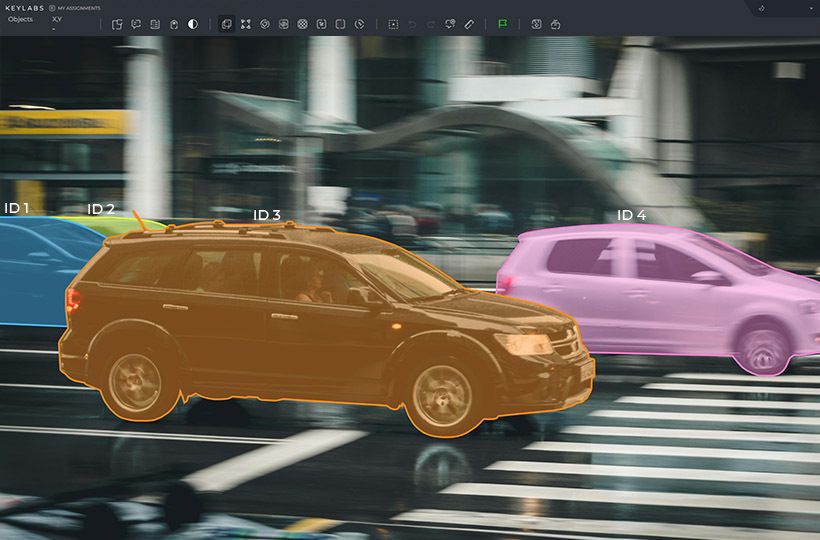
Accelerating AI Development
Automatic annotation is drastically changing the AI development scene, making the data labeling process faster. This advance is key to quickening AI projects, which is important for meeting tough business deadlines. It boosts the efficiency of machine learning (ML) endeavors across the board.
Speed Improvements
Up to 80% of time on computer vision projects is often spent preparing, with 25% of that focused on annotation and labeling. By using automatic annotation, teams cut their manual tasks significantly. This saves time, boosts accuracy, and improves annotation quality, setting high standards for AI model training.
Creating an automated data annotation tool in-house can take 6 to 18 months and costs a lot. But, this investment pays off over time by lowering manual errors and speeding up the annotation process. These gains are key for meeting the often tough deadlines in AI development.
Meeting Business Deadlines
Rapid, accurate data annotation is a game-changer for AI teams, helping them enhance their datasets quickly. This is vital for outpacing competitors in a cutthroat industry. Companies that adopt automated annotation tools often exceed their project deadlines, showing exceptional agility in delivering ML solutions promptly.
This practice also allows for a hybrid approach: a human annotation team works on critical data parts manually, while AI tools cover the rest. It’s more efficient than manual labeling of an entire dataset. This approach optimizes speed and accuracy, improving AI project timelines.
By speeding up data annotation, AI projects become more agile and reliably meet tight deadlines. This positions companies to lead in an ever-changing landscape, ensuring their AI models are developed effectively and up to the highest standards.
Enhancing Annotation Consistency
Keeping data labeling consistent is key for AI model training reliability. As machine learning datasets grow more complex, maintaining high-quality annotations becomes critical. This ensures accuracy and success in AI projects.
Reducing Human Error
Consistent data labeling is made easier through automated methods. Such techniques cut down on human errors. They save significant time in computer vision projects, where annotations often take a quarter of the effort. By making sure labels are consistently assigned across datasets, these tools speed up tasks and make results more foreseeable.
Uniform Data Processing
Automated annotation not only reduces errors but also ensures a consistent approach. Whether using rule-based logic or supervised learning, these tools maintain the same quality standard across all points. This approach quickens training while improving dataset efficiency. It lets human teams tackle challenging cases, leaving repetitive labeling to AI. Thus, it finds the perfect mix of automation and human vigilance.
Let's look at how automated annotation impacts projects:
Criteria | Manual Annotation | Automated Annotation |
---|---|---|
Time Consumption | High | Significantly Reduced |
Error Rate | Higher | Lower |
Consistency | Variable | Uniform |
Cost | Higher | Lower (after initial setup) |
Scalability | Limited | High |
Adopting consistent data labeling and automation makes AI development more efficient. It leads to more accurate and sturdy AI models. This lays a strong foundation for AI innovations and scalability.
Resource Efficiency
The move towards automated data labeling marks a turning point in resource efficiency for AI ventures. It saves both money and time, not just in dataset annotation, but in the broader project context. This approach significantly reduces costs and labor requirements.
Cost and Labor Savings
Embracing automated data annotation dramatically cuts AI project costs. Unlike the lengthy and expensive process of creating a tool from scratch, pre-built solutions are swift to deploy, often within hours. The speed of implementation not only reduces costs but also speeds up the overall project progress.
Optimizing Resources
Through automated annotation, businesses can free up both human and financial resources. These resources can then be directed towards strategic areas, improving the project's efficiency. Keylabs is a prime example of this, enhancing annotation rates and workflows of our team significantly.
These tools enhance the management of annotation teams. They offer features for evaluating skills, reviewing performance, and distributing tasks intelligently. This fosters a productive atmosphere, key for efficient and precise data annotation in any AI context.
Scalability of AI Projects
In the fast-paced world of artificial intelligence, scalability is crucial. As we rely more on data, especially in machine learning, the need to handle and annotate big datasets grows. Let's delve into how automatic annotation copes with enormous data and adapts to the increasing needs in AI projects.
Handling Large Volumes of Data
Automatic annotation is key to managing huge datasets efficiently. By 2030, McKinsey predicts AI could boost global economic activity by $13 trillion. This highlights the importance of systems that can handle big data well.
The global market for data annotation is forecasted to hit $6.45 billion by 2027. This growth shows the rising need for scalable solutions in data labeling. Such systems are a huge help for machine learning models, especially those that need millions of annotated items for training.
Adapting to Increasing Demands
McKinsey also found that up to 75% of AI projects require fresh data every month. An additional 24% need updates daily.
Automatic annotation for AI projects supports this constant data improvement. It helps maintain accuracy and consistency in models. As the need for annotated data grows, platforms that blend annotation with machine learning model support become crucial.
Automatic annotation is crucial for handling the increasing demands of AI projects. It meets both the challenges of big data and the growing need for annotations. Thus, helping organizations leverage AI to its maximum extent.
Integrating Human-in-the-Loop AI
Automatic annotation methods have revolutionized efficiency in data processing. However, integrating Human-in-the-Loop (HITL) AI is crucial for ensuring top-notch annotation quality control. This union of automation and expert input guarantees the precision and trustworthiness of AI training data.
Balancing Automation and Human Expertise
Combining automated processing with human oversight strengthens the annotation process. By 2025, as predicted by Gartner, ‘human-in-the-loop’ solutions will enrich 30% of new legal tech automation offerings. This integration allows experts to address nuanced cases, enhancing the quality of data annotation automation. The dynamic cycle created by Human-in-the-Loop AI encourages ongoing enhancements through expert annotation review and feedback loops.
Quality Assurance in Annotation
HITL significantly elevates quality assurance efforts in AI initiatives. The input from human annotators results in meticulously labeled datasets. This approach refines AI's sensitivity to subjective and contextual aspects. Organizations like CloudFactory leverage human oversight to scrutinize and refine annotations, boosting AI model credibility. Additionally, the hands-on quality assurance enforces adherence to standards, which promotes ongoing enhancement and trust in AI applications.
Method | Benefits | Challenges |
---|---|---|
Automatic Annotation | Speed, Efficiency | Edge Case Handling, Contextual Understanding |
Human-in-the-Loop AI | Accuracy, Quality Control | Slower Annotation Time Compared to Full Automation |
Automatic Annotation for AI Projects
Automatic annotation is changing how AI projects are done, making them more efficient, consistent, and scalable. AI training data is crucial for enhancing machine learning. High-quality annotations are key. That's where automatic annotation techniques shine, offering a fast and reliable way to label data.
For computer vision projects, the preparation stage can take up to 80% of the time, with a quarter of that on annotation alone. By using efficient annotation methods, this time can be cut significantly. This allows AI teams to focus more on development. Creating an internal automated annotation tool is expensive and time-consuming. Instead, ready-made solutions offer a quick setup, sometimes in just hours or days, which is more feasible for many organizations.
Advanced AI projects see big advantages with automated data annotation. It minimizes manual work and cuts down errors. For example, automated image annotation is essential for computer vision models, making workflows faster and quality better. Video annotation, especially in fields like medicine, requires special tools to handle native formats for precise labeling across frames.
Automatic annotation is also cost-effective. It lets human annotators focus on crucial data, with AI tools speeding up the process. This enhances accuracy and efficiency. It also shortens the time to start training models, which is great for testing theories, fixing biases, and improving models overall faster.
Image annotation in AI models can include many tasks like object recognition and segmentation. Advanced tasks like audio annotation, essential for language understanding, and video annotation, used for recognizing moving objects, show the wide application of this technology. Text annotation further refines AI models' language understanding by analyzing meaning and sentiment.
In fields like healthcare, where accurate data is vital, automatic annotation plays a key role. It combines AI training data with efficient tools, ensuring AI models mimic human behavior accurately. This boosts their performance in real-world tasks.
FAQ
What are the key benefits of automatic annotation for AI projects?
Automatic annotation boosts the efficiency of AI training data. It increases data accuracy and fosters the production of superior AI models. This leads to fast, consistent, and top-quality data labeling.
How is automatic annotation defined?
It is a technology called auto-labeling, leveraging advanced ML algorithms. These algorithms automatically generate data annotations. This reduces the need for extensive human involvement.
How does automatic annotation work?
Using sophisticated ML models, automatic annotation ensures consistent labeling. These models are integrated into annotation platforms. As a result, the data labeling process becomes much more efficient.
How does automatic annotation accelerate AI development?
By speeding up the data labeling process, automatic annotation helps AI projects meet deadlines. This acceleration allows for quicker iterative development. It enhances the agility of the entire project.
In what ways does automatic annotation improve consistency in data handling?
It reduces errors caused by humans. Also, it enforces consistent data processing. Consequently, automatic annotation ensures stable and reliable labeling. This is vital for the efficient training of AI models.
How does automatic annotation optimize resource efficiency?
Automatic annotation significantly reduces costs and saves labor. It cuts down the manual labeling effort. This efficiency allows companies to divert their resources to more critical areas. It improves the workflow significantly.
What scalability benefits does automatic annotation provide for AI projects?
It enables AI projects to effectively handle large amounts of data. This includes adapting to rising data demands. Thus, automatic annotation supports the scalability of projects for growing AI applications.
What is the role of human-in-the-loop AI in automatic annotation?
Even with reduced human involvement, human-in-the-loop AI plays a vital role. It integrates human expertise for quality assurance. This ensures the final annotated data meets high standards of accuracy and quality.
What are some notable application areas for automatic annotation?
Automatic annotation shines in Computer Vision and Natural Language Processing (NLP). It speeds up development and enhances AI solution accuracy. This drives innovation in these critical fields.
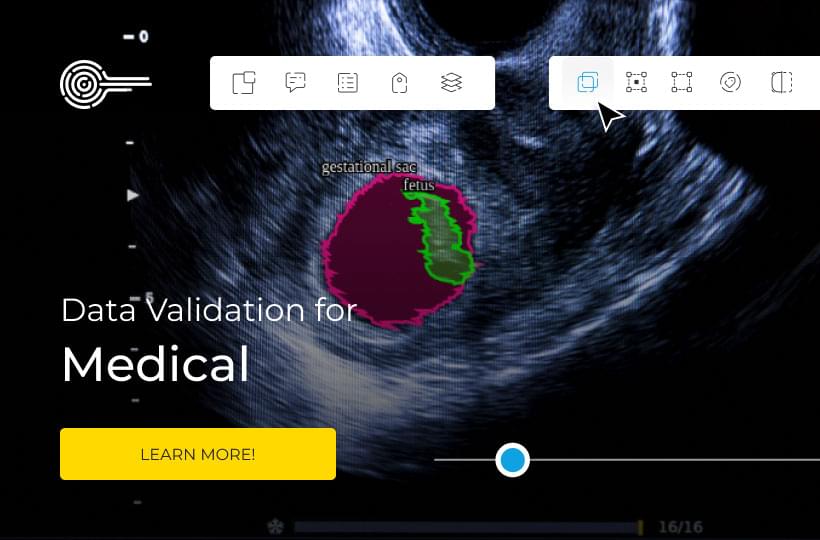