Best Object Recognition Software of 2024: An Expert Review
Pictures are often called a language of their own, and now, with computer vision, machines can also interpret them. Object recognition, a critical area of AI, allows computers to "see." It does so by recognizing and identifying objects in images and videos. The impact of this technology is significant. Experts predict that by 2024, the market for object recognition software will be valued at $10 billion.
This article delves deep into the cutting edge of object recognition. We'll talk about the latest in computer vision algorithms and their impact on different sectors. Discover the upsides and problems with utilizing object detection. This information is crucial for developers, data scientists, and professionals across many industries. Our expert review offers key insights into the premier object recognition software you can find.
Key Takeaways:
- Object recognition is a crucial field in artificial intelligence that allows computers to detect objects in visual images and videos.
- The global object recognition software market is projected to reach $10 billion by 2024.
- This article will provide an expert review of the top object detection tools and software, their applications, and the benefits and challenges associated with object detection.
- By leveraging the latest advancements in computer vision, businesses can harness the power of AI-powered image recognition and advanced detection solutions.
- Object recognition software plays a vital role in automating tasks, improving accuracy, and providing valuable insights across various industries.

What is Object Detection and How Has It Evolved?
Object detection identifies and spots objects in images or videos. Over two decades, it has become key in computer vision. Methods include both traditional techniques and cutting-edge deep learning.
This tech is vital for various tasks. It helps detect pedestrians, animals, and vehicles. It also works for people counting, recognizing faces, finding text, spotting poses, and reading number plates.
Types of Computer Vision Object Detection Methods
There are two main approaches to object detection in computer vision. The first uses older image processing techniques. The second relies on advanced deep learning networks.
- Traditional Image Processing Techniques:
- Edge detection finds areas in images with quick intensity changes. It locates objects using these edges.
- Template matching looks for similar patterns between a template and a target image. It helps identify objects.
- Feature-based methods find unique points in an image using algorithms like SIFT and SURF. They then match these points to known features.
- Modern Deep Learning Networks:
- CNNs are essential for object detection today. They automatically pick up and classify features in images.
- R-CNNs combine region proposals with CNNs for precise object location in images.
- Faster R-CNN outperforms R-CNNs in terms of both speed and accuracy.
Examples of Object Detection Applications
Object detection has many real-world uses across numerous fields:
1. Pedestrian Detection:
It’s crucial for autonomous vehicles in spotting people, ensuring their safety, and making smart urban navigation choices.
2. Animal Detection:
It’s essential for tracking wildlife movement, helping with conservation and wildlife management efforts.
3. Vehicle Detection:
Systems for traffic control and surveillance rely on it. They use it for traffic data collection and improving flow.
4. Face Detection:
It’s key in biometrics, surveillance, and facial recognition technology.
5. Text Detection:
This kind of detection is used to pull text from images and videos. It supports applications like license plate recognition, OCR, and document analysis.
6. Pose Detection:
It’s crucial for sports analysis, fitness apps, and virtual reality to track and understand human body movements and postures.
7. Number-Plate Recognition:
This technology helps in locating and reading license plates. It’s useful for vehicle ID and traffic regulation.
Application | Industry |
---|---|
Pedestrian Detection | Autonomous vehicles, urban planning |
Animal Detection | Wildlife conservation, research |
Vehicle Detection | Traffic management, surveillance |
Face Detection | Biometrics, surveillance |
Text Detection | OCR, document analysis |
Pose Detection | Fitness tracking, virtual reality |
Number-Plate Recognition | Law enforcement, toll systems |
The Most Popular Object Detection Algorithms Today
In recent years, object detection has seen huge strides, thanks to deep learning. Today's go-tos are YOLO, Detectron2, and EfficientDet. Notably, they excel in detecting and pinpointing objects in images or videos and easily slot into various object recognition applications. Thanks to deep learning and powerful GPUs, object detection has reached new heights.
Yolo is notable for its real-time and precise object detection. It breaks images into a grid and predicts boxes and objects in each cell. This quick process doesn't compromise accuracy, thanks to a unique detection approach.
Detectron2, birthed by Facebook AI Research, stands out for its design flexibility. It easily builds top-tier models for object detection. By using deep learning's latest, it's adept at finding objects without wasting resources.
EfficientDet, drawing from EfficientNet, merges efficiency with accuracy. It dynamically adjusts model properties to fit the task at hand. This adaptability has made it shine in object detection challenges, earning it a strong standing.
Advantages of Popular Object Detection Algorithms:
- High-speed object identification and localization
- Precision and accuracy in detecting objects
- Efficient and effective use of computational resources
- State-of-the-art deep learning architectures and techniques
- Flexibility and modularity for customization
popular object detection algorithms
Thanks to deep learning and advanced hardware, these algorithms are making waves in the real world. Their combination of speed, accuracy, and adaptability is fueling their ever-growing usage. This momentum is pushing the boundaries of computer vision further.
Algorithm | Description | Applications |
---|---|---|
YOLO | A real-time object detection algorithm that divides images into a grid to predict bounding boxes and class probabilities. | Autonomous driving, surveillance systems, robotics |
Detectron2 | A powerful object detection library developed by Facebook AI Research, providing a modular and flexible framework. | Image recognition, object tracking, instance segmentation |
EfficientDet | Efficient object detection algorithm that balances model size and computational requirements effectively. | Visual search, object counting, security systems |
Real-World Use Cases of Object Detection
Object detection is pivotal in countless industries. It spans from security and healthcare to agriculture, smart cities, and more. Its precision and flexibility make it vital for process optimization and safety enhancement. A prominent example is its use on production lines to detect people in real time.
The well-being of workers in manufacturing is critical. Real-time detection helps prevent accidents and boosts efficiency. Using these systems, companies can track workers, ensuring their safety and averting dangers.
Real-time person detection improves workflow efficiency and safeguards employees.
Cameras and sophisticated detection algorithms are employed in these settings. They identify individuals and issue alerts for safety breaches and potential accidents. This approach significantly improves workplace safety. Object tracking aids in more than just safety. It offers insights to optimize workflows, pinpoint inefficiencies, and reallocate resources. This data-driven strategy boosts productivity across the operation.
Additionally, such systems streamline regulatory compliance and promote risk-aware cultures. Investing in this technology asserts a company's focus on a secure workplace for its staff.
Benefits of Real-Time Person Detection in Manufacturing Production Lines | Challenges of Real-Time Person Detection in Manufacturing Production Lines |
---|---|
|
|
The Benefits and Challenges of Object Detection
Object detection is a crucial aspect of computer vision. It allows the pinpointing of objects within images or videos. This capability is key for automating tasks, enhancing precision, and offering insights that can transform business operations.
This technology excels in automating the counting, inspection, and check processes. With its accurate detection and localization abilities, tasks are executed more efficiently. This leads to time savings and a reduction in errors. These impacts streamline operations, boost productivity, and increase the output significantly.
Moreover, object detection bolsters accuracy. It does this by clearly identifying objects and removing incorrect detections. Such precision is essential in fields like security and surveillance. There, errors can compromise the reliability of detection and tracking systems.
"Object detection plays a crucial role in automating tasks, improving accuracy, and providing valuable insights for businesses."
By analyzing detected objects, companies can gain profound insights into their operations, customer behavior, and industry trends. These insights empower them to streamline processes, base decisions on data, and ultimately, boost business performance.
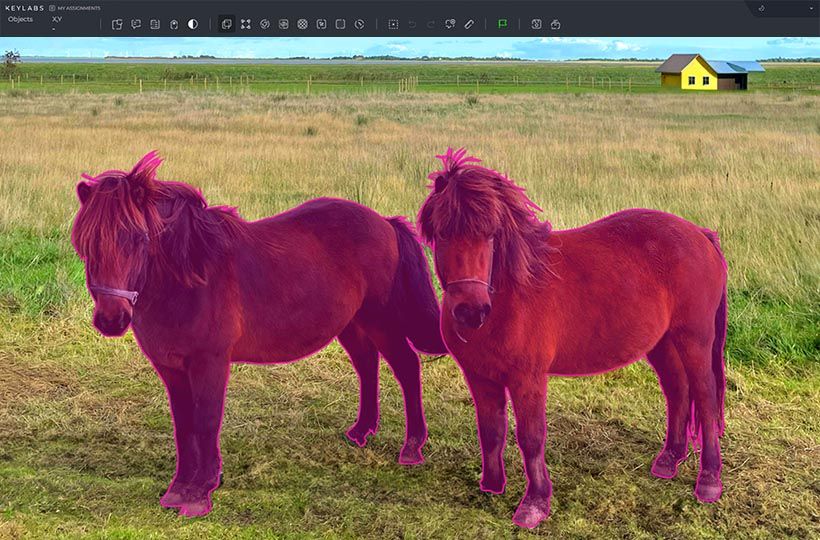
Yet, object detection also brings its own set of challenges. A primary hurdle is the significant computational burden it imposes. The sophisticated algorithms and networks require substantial processing capabilities. This inflates hardware needs and operational costs
Advantages of Object Detection | Disadvantages of Object Detection |
---|---|
|
|
The Difference Between Object Recognition and Object Detection
Object recognition and object detection differ greatly in computer vision. Both tasks aim to identify objects in images but do so in distinct ways. Object recognition finds what an object is, while object detection locates it precisely in an image.
Object Recognition: Image Classification without Localization
Known as image classification, object recognition discerns the object's class in an image. It's goal is not to pinpoint the object's location but to classify it based on visual traits.
Advancements in deep learning have vastly improved object recognition. These methods incorporate sophisticated neural networks. They're honed on vast datasets to spot patterns and accurately identify objects.
For instance, a model could recognize different dog breeds accurately within images. It would name the breed, like a Labrador, omitting exact positioning information.
Object Detection: Identifying Objects with Localization
Object detection surpasses recognition by also offering localization details. It pinpoints exactly where an object is in an image. Deep learning algorithms for object detection not only confirm an object's presence but also draw a boundary around it. This results in precise location information within an image.
Various approaches, such as R-CNN, YOLO, and SSD, are used for precise object identification. They enhance the algorithm’s ability to detect and position objects efficiently.
"Object detection is an essential computer vision task that combines object recognition with precise localization, enabling machines to not only identify objects but also understand their spatial context within images."
In an image with diverse elements like cars and traffic signs, an object detection model will identify each element clearly. It marks the objects with bounded boxes, offering detailed spatial data.
Deep Learning Advancements in Object Recognition and Object Detection
Deep learning has pushed both object recognition and detection forward. This technology employs neural networks with layers for extracting complex, meaningful features. It helps machines make precise predictions.
For image recognition, convolutional neural networks have set new standards. They excel in recognizing objects accurately by understanding high-level representations.
Object detection has also benefited from deep learning, notably through models like YOLO and SSD. These have enhanced both the speed and accuracy of object detection, making them practical for instant use scenarios.
An Illustrative Example:
Consider this illustration to grasp the distinction:
- An object recognition AI could spot a dog in an image accurately.
- An object detection AI would go further by identifying the dog and framing it in a bounding box.
Aspect | Object Recognition | Object Detection |
---|---|---|
Objective | Identify the class or category of an object in an image | Identify and locate objects within an image |
Localization Information | Does not provide localization information | Provides precise localization of objects with bounding boxes |
Common Techniques | Deep learning object recognition algorithms | Region-based CNN (R-CNN), You Only Look Once (YOLO), Single Shot MultiBox Detector (SSD) |
Use Case Example | Identifying dog breeds in images | Detecting cars, pedestrians, and traffic signs in self-driving car applications |
The Most Popular Computer Vision Tools in 2024
Computer vision has become a key area for data scientists and machine learning experts. In 2024, they rely on powerful tools like OpenCV, TensorFlow, CUDA, MATLAB, and Keras for their projects.
OpenCV is a top choice for image processing with its vast community support. It provides a wide array of functions and algorithms for tasks such as image manipulation, feature detection, and object recognition.
For those engaged in deep learning, TensorFlow shines as an open-source platform. It excels in training deep learning models for computer vision. Its adaptability and scalability stand out, attracting many for their projects.
CUDA, from NVIDIA, is vital for utilizing GPU power in image and video processing. It accelerates complex algorithms. This means quicker, more effective processing for computer vision tasks.
MATLAB’s reputation stems from its robust data analysis and prototyping features. It's favored for its support in image processing and computer vision algorithms. These offerings appeal to both researchers and scientists.
Keras, being Python-based, simplifies the building and deployment of deep learning models. It acts as an interface for TensorFlow, enhancing user experience. This is crucial for developing sophisticated computer vision applications.
Latest Technological Advances in Computer Vision
Computer vision has progressed significantly in recent years due to deep learning in object detection and tracking. Breakthroughs in these areas have transformed computer vision in sectors like healthcare, automotive, and manufacturing. This has enabled more precise applications across industries.
Advancements in deep learning have enhanced the accuracy and efficacy of computer vision systems. These improvements enable systems to not only detect objects but also to track their movement. This is achieved through the use of deep neural networks, which are pivotal for object recognition.
Edge AI is a central concept that pushes the field forward. By processing complex AI tasks on devices locally, it reduces delays and the need for large network data transfers. This approach, known as edge computing, sharpens the system's real-time decision-making abilities.
An integration of IoT and AIoT has further upgraded computer vision. It allows for the connection of various devices to the web. This connectivity enhances the analysis of real-time data for applications such as smart surveillance and quality control improvement.
These technological advances in computer vision have introduced new possibilities. They have driven significant changes and innovations in multiple sectors through deep learning, edge AI, and IoT/AIoT conjunctio
Advantages and Disadvantages of Object Detection Tools
In the realm of computer vision, object detection tools shine with unmatched flexibility. They can adapt to diverse tasks and custom needs. This makes them valuable for identifying items in images or videos, streamlining tasks, or offering insights that fuel business decisions. Their true worth is evident across various sectors.
These tools empower organizations to automate mundane but critical operations. Processes like counting, inspecting, and verifying items see a boost in efficiency and accuracy. By handling repetitive tasks, they lighten the workload for human workers, allowing focus on more intricate and strategic endeavors.
"Object detection tools provide valuable insights and help businesses make data-driven decisions. They can be used for market research, quality control, and inventory management, providing an accurate and efficient way to analyze visual data."
Yet, amidst their benefits, object detection tools pose some challenges. Notably, they require substantial computing power, leading to high operational costs. Operating these tools at scale means investing in powerful systems and maintaining sophisticated infrastructure.
For deep learning-based object detection models, achieving real-time results demands advanced GPUs and dedicated hardware. This prerequisite may put a strain on organizations with limited financial means. The hurdle of affordability could hinder widespread adoption within the business world.
Summary
Object recognition software is vital in today's computer vision technologies. It empowers systems to perceive and interact with their surroundings. Leading the way are advanced algorithms like YOLO, Detectron2, and EfficientDet. These are praised for their speed and accuracy in spotting objects. Their contributions are reshaping the landscape of object detection.
Nevertheless, there are hurdles to face, including the cost of utilizing such technology. The heavy computational requirements can strain budgets. Yet, with the aid of cutting-edge computer vision tools, these obstacles can be surmounted. This paves the way for organizations to fully embrace the benefits of AI-driven image analysis and sophisticated detection methodologies.
FAQ
What is object detection?
Object detection is the process of identifying and locating objects within images or videos.
How has object detection evolved?
Over the past two decades, object detection has grown significantly. It is now a key challenge in computer vision.
What are some examples of object detection applications?
Its applications are vast, from finding pedestrians and animals to detecting vehicles and text. These tasks show its broad utility.
What are the most popular object detection algorithms today?
Today, YOLO models, like the YOLO series, and other notable algorithms such as Detectron2 and EfficientDet, lead the field.
What are the real-world use cases of object detection?
Industries benefiting from object detection range from security to agriculture. There, it aids in tasks like tracking objects in real time.
What are the benefits of object detection?
Its advantages include task automation, enhanced accuracy, and insights that prove valuable for businesses.
What are the challenges of object detection?
The significant computational and processing power required pose challenges. These can lead to higher operating costs.
What is the difference between object recognition and object detection?
Object recognition determines the object's type without pinpointing its location. Object detection does this and provides the item's position as well.
What are the most popular computer vision tools in 2024?
In 2024, tools like OpenCV, Keras, TensorFlow, and others remain at the forefront of computer vision technology.
What are the latest technological advances in computer vision?
The field has seen recent progress in deep learning for object detection, edge AI, and the fusion of IoT with AIoT.
What are the advantages and disadvantages of object detection tools?
While these tools are flexible and streamline automation, they can be computationally intensive. Moreover, they require demanding processing resources.
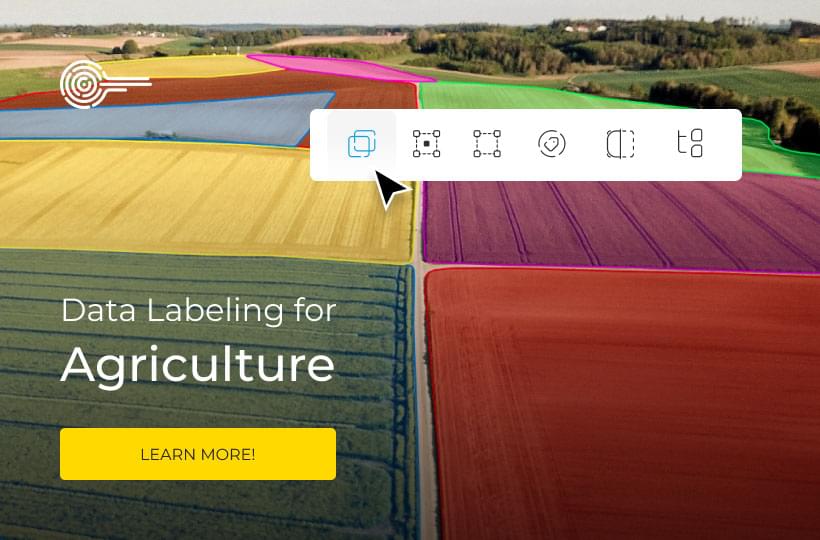