Bias In Data Annotation [Video Labeling For Facial Expressions]
Machines recognizing emotions is a little too futuristic for some. Just like humans, machines have biases. Facial and emotion recognition technology is picking up speed, but it has major biases. Most data for these programs comes from Western datasets. There’s a lack of ethnically diverse databases. Across different ethnic groups, we’re able to judge expressions accurately. An AI video labeling program doesn’t have enough reference data to make the same judgments.
It’s reminiscent of a scene from one of Arnold Schwarzenegger’s movies. A robot looks at a human, instantly reading their emotions and intents. That’s precisely what facial and emotional recognition software will be used for one day. But hold on. There's a positive side to this.

Crash Prevention
Feeling drowsy behind the wheel? Your face will alert your car and keep you safe. It’s not unreasonable to think that your car will adjust music, temperature, and even scents based on your expressions.
Personal Assistants
Alexa and Siri will be more useful than ever once they can read facial expressions. Personal assistants will use a built-in AI video labeling tool to monitor your satisfaction. How the information is used will be up to you. For example, you might use it to fine-tune your Spotify playlist. Your assistant might even be able to choose movies based on your current emotions.
Law Enforcement
Chinese law enforcement has already used AI emotion recognition technology to aid police interrogations and even monitor behavior in schools. The old-fashioned lie detector tests with leather chest straps and pulse monitors will soon make way for AI. Of course, this poses another deeper question about how this powerful technology will be used. Unfortunately, we’ve got to save that for a future blog post. It’s likely that developed nations will create policies and limitations for emotion recognition technology.
A Study Out Of Korea Highlights Labeling Tool Flaws [KVDERW]
The top emotion databases focus on Western facial expressions. This makes emotional recognition difficult within Korean populations.
A team of five researchers faced this problem head-on. The paper is called Korean Video Dataset for Emotion Recognition in The Wild, or KVDERW. The study focused on Eastern people, specifically Koreans. Initiatives like this are valuable, building a more global dataset of emotions.
They compiled a dataset of 1200 video clips to overcome their lack of data. These weren’t Tik Tok or Instagram reels. They needed high-quality footage. Six evaluators poured over the videos and made detailed annotations. They designated main faces, plus they included background and head pose information. In the end, they developed a semi-automatic labeling tool for ML. The tool can search for specific faces and make emotional predictions.
They’ll continue improving this emotion recognition tool while the rest of the world follows suit.
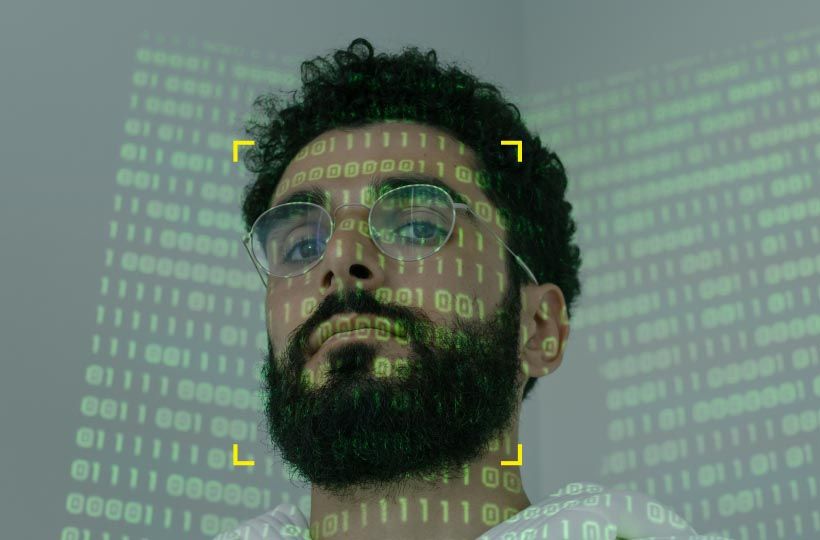
Bias Isn’t Exclusively A Human Trait Anymore
Let’s get back to the problem of bias. For humans, this means tendencies to lean one way or the other based on prejudices. As a result, we make incorrect and misguided choices. Machine bias is similar but distinctly different. In the world of AI, bias occurs when datasets are unbalanced or have information gaps.
There’s often a disconnect between the programs and the real world. The emotion recognition issues we mentioned earlier are a perfect example of this. We’ve unbalanced the data with more Western facial data than from other ethnic groups. So if a recognition program attempts to read the emotions of someone of Asian descent, it does it with a Western facial bias.
Sometimes we introduce bias to machines by excluding data. For example, let’s say an online business has a 3% base of Canadian customers. They decide it’s not worth annotating the data and discarding it. This is called exclusion bias. Prediction models won’t account for your Canadian customers, so creating strategies for them will be difficult.
Let’s go over the main examples of data bias, with an example of each one.
The Seven Main Types Of Data Biases
Sample Bias/Selection Bias
If we only train a labeling tool to annotate Mars rocks, we can’t expect it to perform well on Pluto. This is sample bias. It’s the reason why emotion recognition works best on Western faces and struggles with Eastern facial features. The solution is more diverse data.
Recall Bias
Recall Bias is a problem arising from inconsistent data labeling. Let’s say annotators are labeling apples. They categorize the apples as Blemished, Partially Blemished, and Unblemished. If too many Blemished apples are wrongly labeled Partially Blemished, the quality of the data is eroded. As a result, AI software might continually label apples incorrectly.
Observer Bias/Confirmation Bias
This is a very “human” bias. Observer bias happens when we expect to see results before conducting research. Our subjective thoughts can influence our research and testing. Machines don’t have subjective bias, but they might inherit it through human training.
Racial Bias
Racial bias is not traditionally a data bias, but it will influence the systems we create. This kind of bias is apparent in facial recognition software, whether conscious or subconscious. It’s also present in vocal recognition software, which favors the English language.
Association Bias
Here’s an example of association bias. Imagine telling your child that your dogs are both females. If they never saw other dogs, they might think only female dogs exist. Going further, maybe your child thinks male dogs live outside and females inside.
Machines extrapolate the data we feed them. An AI program forms beliefs based on the data it sees, even if there is more to the story.
Measurement Bias
Recall bias is very similar to measurement bias. This occurs when data is gathered incorrectly. Like building a house on a bad foundation, AI labeling tools won’t perform optimally with inconsistent data.
Exclusion Bias
Exclusion bias is omitting data that is thought to be unimportant. This can happen in specific cases or systematically over long periods.
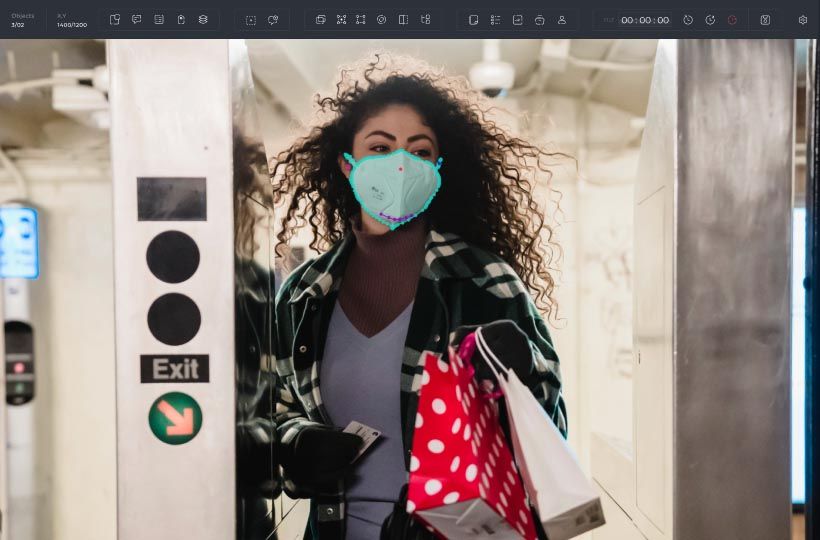