Boost Your Data Labeling Accuracy with These Pro Tips
In today's tech world, the precision of machine learning models greatly depends on data labeling. Automated data labeling is suggested for huge databases, especially with a minimum of 1,250 objects. But aiming for at least 5,000 objects is best for strong model performance. This highlights the critical need for large, quality datasets to train advanced algorithms. Here, the aim to enhance data labeling accuracy is crucial.
Key Takeaways
- Automated data labeling is critical for creating substantial and precise datasets necessary for training AI models efficiently.
- Understanding the impact of data labeling on AI performance is essential for businesses leveraging machine learning technologies.
- Adopting a hybrid approach to data labeling can streamline processes while balancing the accuracy and efficiency needed in AI development.
- With the rise of automated data labeling, the ability to discern and implement best practices has become an invaluable skill in the tech industry.

Understanding the Pillars of Data Labeling Accuracy
In machine learning and AI, accurate data labeling is crucial for good models. It's important to follow data annotation best practices. This ensures the process is done right.
Essential Components of Accurate Data Annotation
Success in data annotation comes from following good practices. Clear annotation guidelines are key. They help annotators make consistent, accurate data marks. Guidelines reduce confusion and errors, making data more reliable.
Impact of Data Selection and Diversity on Label Quality
Choosing the right data selection is about picking diverse, real-world datasets. Diversity impact on label quality is huge. It helps AI understand different situations better. Diverse data leads to stronger, more versatile AI models.
Establishing Clear Annotation Guidelines
AI needs clear rules to understand human input correctly. Setting clear annotation guidelines defines the path to good data labeling. With detailed instructions, businesses improve their data's accuracy. Good guidelines are vital for accurate machine understanding.
Element | Description | Impact |
---|---|---|
Data Selection | Process of curating diverse and relevant datasets | Crucial for model's ability to generalize |
Annotation Guidelines | In-depth instructions for labeling data | Ensures consistency and reduces annotator subjectivity |
Diversity in Data | Integration of varied data to mirror real-world scenarios | Improves label quality and model resilience in diverse situations |
The combination of data annotation best practices, careful data selection, impact of diversity, and clear annotation guidelines boosts AI model effectiveness. Recognizing and using these elements improves data labeling quality and function.
Strategies for Better Data Annotation
Data annotation is key for AI accuracy and machine learning precision. Organizations need strategies for better data annotation for high-quality datasets. This involves annotator expertise, iterative training, and quality control in annotation workflows.
Let's explore how to improve data annotation.
Enhancing Annotator Expertise for Improved Precision
To excel in data annotation, we must invest in annotator expertise. Well-trained annotators understand data complexities better. Thus, specialized training programs are essential.
Training should focus on real-world scenarios to enhance skills. This leads to better data quality.
Iterative Training and Refinement Techniques
Iterative training enhances data annotation continuously. It involves regular feedback to refine the annotation process. This way, businesses can improve their data labeling.
Businesses adjust to project changes better through iterative training. Annotators become more skilled, and categorization improves.
Incorporating Quality Control in Annotation Workflows
Quality control in annotation workflows ensures annotation integrity. Through rigorous checks and reviews, businesses catch errors early. Also, statistical methods help measure annotator performance accurately.
This leads to reliable annotated datasets.
Strategy | Key Benefits | Implementation Example |
---|---|---|
Enhancing Annotator Expertise | Increase in accuracy and consistency of annotations | Specialized training modules tailored to project specifics |
Iterative Training | Continuous learning and improvement of annotation techniques | Weekly review sessions incorporating feedback |
Quality Control Measures | Higher data integrity and reliability of annotated datasets | Regular audits and cross-validation checks within annotation teams |
By adopting these strategies, organizations can improve their data annotation significantly. It helps build smarter computer vision systems that predict and analyze better. This keeps organizations innovative and efficient.
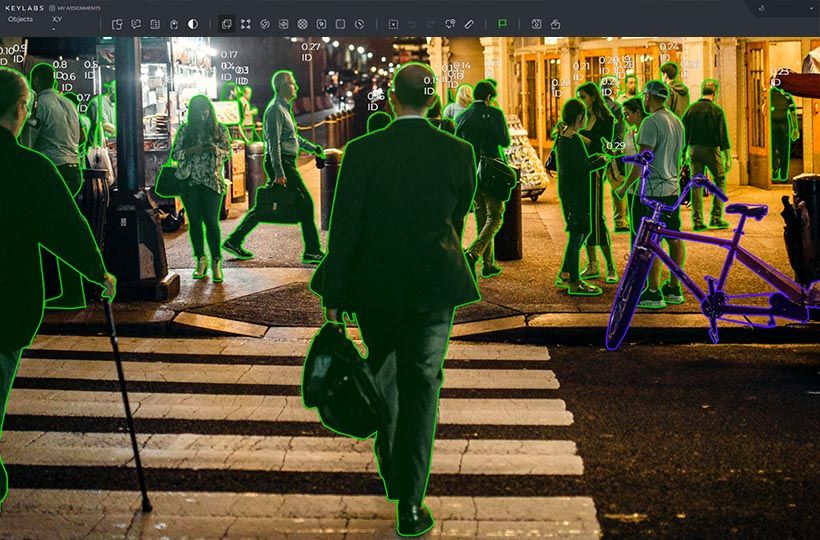
Tools and Technologies for Data Labeling Enhancement
The use of tools for data labeling is key in today’s AI and machine learning fields. High-quality training datasets are essential for models to work right. Advanced data labeling software such as Keylabs leads the way, balancing speed and accuracy.
Labeled data is crucial for supervised learning, teaching algorithms how to predict. Unsupervised learning, on the other hand, finds patterns in unlabeled data. Choosing the right data labeling tool is very important. Meanwhile, NLP (Natural Language Processing) grows, blending tech and linguistics to understand human language.
Advanced Software for Accurate Data Labeling
Tools range from manual annotation to semi-automated systems such as those provided on our platform. These advanced data labeling software help achieve accuracy. These tools are designed for diverse data, from databases to multimedia.
The Role of AI in Automating Data Annotation
AI plays a big role in making data annotation faster and cheaper. Specialized tools like Keylabs' AI labelling use both AI and human input to improve efficiency.
Choosing the Right Tools for Your Data Labeling Needs
Finding the balance between automated and manual tools is crucial. The right choice depends on the project's needs. Data scientists need a variety of tools for the best results.
There are many tools and technologies for data labeling today. Choosing wisely is key to unlocking technology's potential.
Innovative Techniques Across Different Industries
Artificial intelligence is growing fast, and smart data labeling is key. Many industries use these methods to improve AI and machine learning. These methods help fields from healthcare to automotive create big improvements. They make things like precision medicine and safer self-driving cars possible.
In healthcare, data labeling helps spot diseases better and creates personal treatment plans. It's a big step towards medical discoveries. For self-driving cars, it's crucial for safe travel and knowing what's around. This ensures driver-assistance systems are reliable.
Customer service and tech also benefit from audio data labeling. It helps make virtual helpers that can translate languages in real-time. Text labeling boosts AI in understanding and communicating across different industries.
The innovative data labeling technique called human-in-the-loop uses human checks with automated speed. This mix helps make fewer mistakes and trains algorithms well. It's great for learning systems that need correct data from the start.
Choosing the right data labeling method is tricky. Companies must weigh time, cost, and accuracy. This decision influences their project's outcome greatly.
Industry | Data Type | Labeling Technique | Application |
---|---|---|---|
Healthcare | Medical images | Manual labeling, Human-in-the-loop | Disease diagnosis |
Automotive | Video, LiDAR | Semi-supervised learning, Synthetic labeling | Autonomous driving |
Tech | Audio | Crowdsourced labeling, Programmatic labeling | Virtual assistants |
Information Technology | Text | Human-in-the-loop, Automated labeling | Natural language processing |
Computer vision and natural language processing show these methods' wide impact. They fine-tune AI for tasks like image segmentation and emotion analysis. Quality data labeling is crucial for AI's success in various applications.
As industries blend AI into their operations, excellent data labeling remains essential. Setting best practices, encouraging teamwork, and focusing on data quality are vital. They guide companies through the complex world of data labeling, fueling an AI-powered future.
Data Labeling Accuracy Improvement Tips for NLP and Computer Vision
As AI gets more advanced, we need to label data more accurately. This is true for natural language processing (NLP) and computer vision. Good data preparation helps machine learning models work better. It also helps us make better decisions based on AI.
NLP helps machines understand human language. It needs data labeled correctly. Customizing labels for NLP is key. This helps models understand different ways people talk and specific terms used in industries.
Labeling is more than recognizing words. It involves finding and categorizing objects in language data. This helps understand the context of words and phrases.
Customizing Labels for Enhanced NLP Model Performance
To make NLP models better, we need to customize labels. This includes thinking about words with multiple meanings and how people show emotion when they talk. A mix of manual and automated labeling adds depth.
Using supervised learning and expert knowledge makes NLP data labeling more accurate.
Techniques for Precise Object Recognition in Image and Video Data
In computer vision, recognizing objects in images and videos is crucial. Success comes from manual labeling and machine learning. Machine learning models use transfer learning to get smarter. This makes them more efficient.
Addressing Challenges in Speech Recognition and Healthcare Data Labeling
Speech and healthcare data labeling have unique challenges. Speech recognition must deal with different ways people talk. Healthcare data labeling needs to be very accurate and keep patient information private. High accuracy is essential for good healthcare monitoring and voice interfaces.
Aspect | NLP | Computer Vision | Speech Recognition | Healthcare |
---|---|---|---|---|
Main Challenge | Linguistic Nuances | Object Identification | Audio Variability | Data Precision |
Key Technique | Entity Annotation | Automated Labeling | Accent Recognition | Manual Expertise |
Quality Control | Expert Review | Transfer Learning | Predicted vs. True Labels | Compliance Checks |
Data Sources | Text Corpora | Images/Videos | Speech Datasets | Medical Records |
To sum up, using tips to improve data labeling for NLP and computer vision is vital. Along with strategies for speech recognition and healthcare, it's key for AI success. By focusing on data labeling challenges in each area, we can make AI more relevant and accurate.
Data Labeling Best Practices for AI Training Data
Accurate data labeling is key for top-notch AI training data. It tells machines how to learn efficiently. As tech grows, sticking to the best data labeling practices is vital. It helps keep up with AI and machine learning progress.
In AI, labeled data is crucial for supervised learning. Unlabeled data is used in unsupervised learning. This difference is crucial for setting up effective machine learning systems. Making sure data is labeled right is super important. It ensures that quality checks work well, impacting machine learning success.
The area of computer vision is expected to grow from USD 25 billion in 2024 to 175,72 billion by 2023. Better data annotation adds more detail to data. This boosts machine learning in many fields, including computer vision tasks. Data labeling is important but takes a lot of time and money. Yet, correct data labeling can lead to big discoveries. It helps with predicting trends and making forecasts.
AI training data
Each method has its pros. Crowdsourcing is often cheaper and faster. But, it's important to note that mistakes can happen. This can lower data quality and how smart models are.
- Using data labeling best practices boosts data exploration,
- Improves how well search engines work,
- And makes online shopping suggestions more on point.
Today, new methods and active learning help labelers find key data easier. This makes the process better and more precise. Labeling data right takes clear guidelines, teaching the labelers, strict quality checks, and always getting better.
Data Labeling Approach | Benefits | Risks | Suitability |
---|---|---|---|
Internal Labeling | Direct control over process and quality | Resource intensity | Small, specialized datasets |
Synthetic Labeling | Generates large datasets quickly | May lack real-world complexity | Pre-training tasks |
Programmatic Labeling | High efficiency, use of scripts | Requires precise algorithms | Structured tasks, large volumes |
Outsourcing | Expertise, scalability | Less direct control | Time-sensitive, large projects |
Crowdsourcing | Cost-effective, speed | Variable quality, management complexity | Diverse, unstructured tasks |
In summary, sticking to data labeling best practices is crucial for great AI training data. The aim is a balance where machine learning models are accurate, efficient, and precise. This combination drives AI forward, unleashing its full, innovative power.
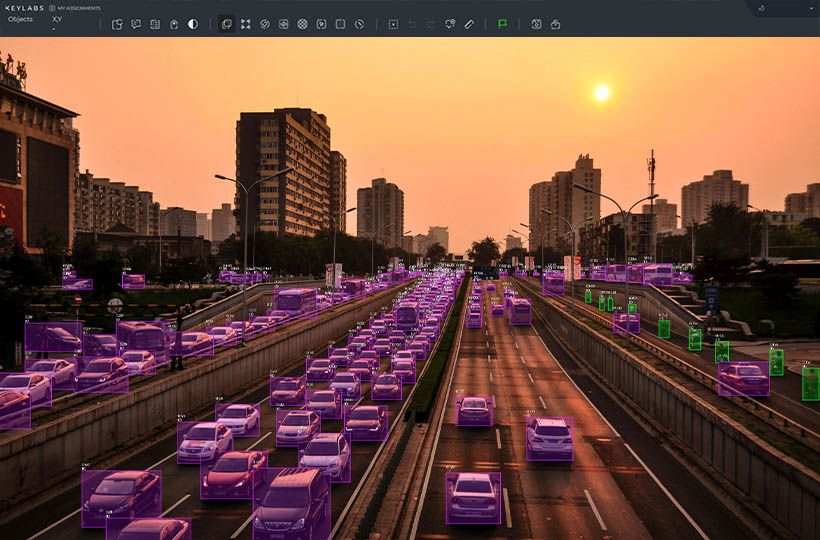
Increase Data Labeling Precision with Collaborative Efforts
To truly harness machine learning in healthcare, finance, and transportation, improving increase data labeling precision is key. The rise of deep learning shows the need for quality data. As datasets grow, one person often can't keep up.
Fostering Team Synergy in Annotating Large Datasets
Large datasets need a team effort. Team synergy in annotating large datasets speeds up and improves data labeling. Ensemble learning combines multiple models, making data more reliable.
This boosts AI accuracy. Advanced analytics help teams manage large data volumes well.
Role of Communication in Quality Annotation Practices
Good communication keeps everyone aligned, reducing errors. This also involves knowing data management and approach nuances.
Good communication helps label data correctly. This reduces AI bias risks and keeps data labeling accurate.
Collaborative data labeling and good communication boost increase data labeling precision. They're key as industries focus on data. This teamwork tackles current issues and fuels future AI success.
Role of Data Governance in Data Labeling Quality
The link between data governance and data labeling is vital for tech companies. These companies strive to offer top-notch data annotation services. Data governance in data labeling ensures the data's quality and reliability. It safeguards the decision-making that relies on machine learning.
Importance of Metadata in Tracking Data Lineage
Knowing the history of data is essential. Metadata for tracking data lineage helps follow the data's journey. It shows where the data came from, how it changed, and who handled it. This is key for good data labeling.
Ensuring Data Privacy and Compliance during Labeling
In today's digital age, following data privacy and compliance in labeling is a must. Laws like GDPR, HIPAA, and CCPA set the rules for handling data. To keep information safe and maintain trust, companies must focus on compliance during data labeling tasks.
Key Pillar | Considerations | Impact on Data Labeling |
---|---|---|
Accuracy and Consistency | Regular quality checks and annotator training | Enhances decision-making accuracy in AI applications |
Data Security | Adherence to GDPR, HIPAA, CCPA | Prevents unauthorized data access, ensuring privacy and compliance |
Scalability | Capability to handle evolving labeling demands | Future-proofs data labeling operations |
Automation | Employing advanced tools and software | Improves speed and quality of the data labeling process |
Customer Service | Quality assistance with a single point of contact | Enhances client experience and project management |
Data governance helps companies improve labeling quality and manage data well. As we move into the age of generative AI, cleaning and labeling data properly becomes crucial. These steps are the foundation for AI innovation and trust.
Measuring the Impact of Labeling Accuracy on Model Efficacy
The goal of measuring impact of labeling accuracy is crucial for AI success. It makes sure supervised learning is precise. It highlights growth in fields like computer vision and NLP.
Programmatic labeling uses scripts and cuts down on time. Unlike internal labeling, which needs lots of resources. Crowdsourcing offers a fast alternative, making the process open to all.
Quantitative Metrics for Assessing Annotation Accuracy
The need for quantitative metrics for annotation accuracy is rising. These tools offer detailed analytics. They play a key role in refining AI, helping fields like computer vision to improve.
Case Studies: Improved AI Performance through Enhanced Labeling
Case studies on improved AI performance show the value of better labeling. NLP benefits greatly from accurate labeling. It powers spam filters, translations, and virtual helpers effectively.
Continuous Improvement and Feedback Loops
Setting up continuous improvement and feedback loops makes labeling a dynamic process. It gets better with time. Insights from quantitative metrics, studies, and feedback boost labeling accuracy.
Labeling Approach | Time Efficiency | Resource Intensity | Suitability for Industries |
---|---|---|---|
Internal Labeling | Lower | Higher | Better for Larger Companies |
Programmatic Labeling | Higher | Lower | Suitable for Scalable Tasks |
Crowdsourced Labeling | Higher | Lower | Ideal for Wide Distribution |
Data Labeling Accuracy Improvement Tips
To improve machine learning and artificial intelligence, we must focus on data labeling accuracy. Making our labeled data more precise is essential for technological progress in areas like computer vision and natural language processing. This section offers tips and strategies to tackle common challenges and make the most of data labeling.
Pro Tips for Enhancing the Precision of Labeled Data
Getting better at data labeling starts with a smart approach to annotating data. For projects that rely on labeled data, using intuitive interfaces and checking labels carefully are key steps. Active learning, where models get better as they receive more data, also helps make annotations more precise. This process improves both the data labels and the model's accuracy over time.
Leveraging Domain Expertise for Accurate Annotations
Using the know-how of industry experts is critical for detailed data labeling. These experts understand the context and fine points that are hard to spot otherwise. Their insights are especially valuable in areas like medical imaging or autonomous vehicles. With their help, data labeling becomes more precise and aligns better with real-world needs.
Optimizing the Labeling Process with Effective Management Strategies
Effective management strategies are crucial for efficient data labeling. Since getting high-quality labeled data is tough and expensive, choosing the right labeling method is important. Organizations must pick a strategy that suits their needs and budget. Automating some parts of data labeling can save costs and boost efficiency, helping to scale operations while keeping labels accurate.
Conclusion
In the world of machine learning and artificial intelligence, accurate data labeling is key. We've seen that even the best algorithms rely on good training data. Since research by MIT's Computer Science and Artificial Intelligence Labs shows up to 3.4% of data can have errors, focusing on precision in labeling is crucial.
Seeing mistakes in projects like Google's Quickdraw shows the impact of poor labeling. Even a 10% error rate can greatly affect an AI's performance. Tools from Keylabs can greatly help lessen mistakes. We use software and diverse teams to improve data annotation quality.
Keeping a close eye on quality control is very important. It helps AI models learn better and ensures data governance is doing its job. With careful work and teamwork, you can make AI both powerful and fair. The drive to improve data labeling will shape the future of AI.
FAQ
What are the essential components of accurate data annotation?
The essentials of accurate data annotation are data selection and clear guidelines.
How does data selection and diversity impact label quality?
Choice and variety of data are key. They directly improve label quality and AI performance.
How can businesses establish clear annotation guidelines?
To ensure high-quality annotations, businesses should set precise guidelines. This gives annotators a clear idea of what's needed.
What strategies can businesses adopt for better data annotation?
Businesses can enhance data annotation by boosting annotator skills. They should also use iterative training and enforce quality control.
How can businesses enhance annotator expertise?
Comprehensive training programs are vital. They equip annotators with the skills for quality annotations.
How can businesses incorporate quality control in annotation workflows?
Introducing checks like spot-checking ensures data reliability. Monitoring annotations is crucial for quality control.
What tools and technologies can enhance data labeling accuracy?
Advanced software and AI automation greatly improve accuracy. User-friendly tools are particularly effective.
How does AI play a role in automating data annotation?
AI reduces human effort and increases efficiency. It plays a key role in automating the labeling process.
How can businesses choose the right tools for data labeling?
Choosing tools involves assessing complexity and scalability. Compatibility with current systems is also important.
What are some innovative data labeling techniques across different industries?
Innovative techniques vary by industry needs. For example, healthcare uses medical image segmentation, while autonomous systems focus on object identification.
What are some tips for improving data labeling accuracy in NLP and computer vision?
Customizing labels for NLP and precise object recognition in images boosts accuracy. These are key for NLP and computer vision.
What are the best practices for AI training data labeling?
Best practices include clear guidelines and thorough training for annotators. It's also key to implement quality control and iterative refinement.
How can collaborative efforts increase data labeling precision?
Team synergy and effective communication are crucial. They enhance precision in annotating large datasets.
What is the role of data governance in data labeling quality?
Data governance tracks data lineage and ensures privacy. It's vital for maintaining quality in the labeling process.
How can the impact of labeling accuracy on model efficacy be measured?
Quantitative metrics assess annotation accuracy. Case studies also show AI performance improvement through better labeling.
What are some pro tips for enhancing the precision of labeled data?
Leveraging domain expertise is essential for accurate annotations. Effective management strategies optimize the labeling process.
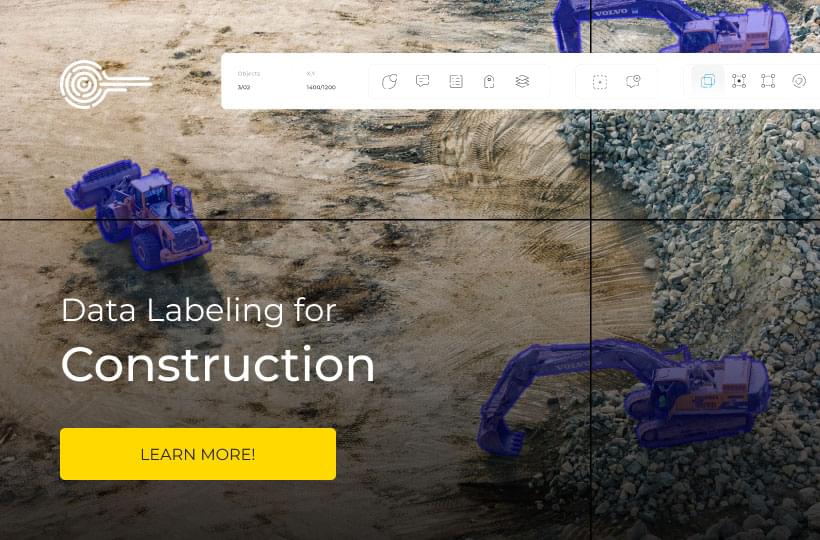