Citizen Scientists for AI: How You Can Contribute to AI Image Recognition Development
Ordinary people can make a significant mark in AI development. As it turns out, many citizen science tasks are designed with that in mind. They tap into skills that most individuals already have. Regular folks push forward the frontiers of AI image recognition. This phenomenon, known as citizen science, involves millions around the world. It marries the excitement of the public with the rigors of scientific research. Together, they gather and analyze data for research projects.
One shining example is Penguin Watch, a collaboration that lets everyday people help monitor penguin populations in Antarctica. This work doesn't just connect the public with science; it also forges strong links with technology giants. For instance, partnerships between Ernst & Young and Microsoft, in initiatives like Snapshots at Sea, show the power of working together. Surprisingly, despite these opportunities, only a small portion of U.S. adults take part in such projects. This low participation hints at a vast potential for more people to join in. If more individuals get involved, it can spark continued interest in various scientific fields.
Key Takeaways
- Most citizen science tasks are well-structured and require general skills.
- Public participation has a significant impact on ecological monitoring and comprehension.
- Collaborations between citizens and AI can enhance technological outcomes.
- Projects like Penguin Watch and Snapshots at Sea exemplify successful citizen science contributions.
- Increasing participation can amplify the benefits to various scientific disciplines.
- Opportunities for involvement exist across many areas of AI development.

Introduction to Citizen Science and AI Image Recognition
Citizen science is a powerful initiative that allows the public to partake in scientific research. It significantly impacts fields like ecological monitoring. Here, volunteer contributions in data collection and analysis are vital. For instance, eBird collects vast amounts of bird sightings, improving data quality and developing species models by filtering irrelevant information.
Overview of Citizen Science
Since its debut, citizen science has bridged a gap between the public and scientists, promoting a better understanding of scientific work. By incorporating AI, the contributions of citizen scientists have increased in efficiency. This is done by AI algorithms that eliminate errors in image analysis, reducing the manual processing of data. New systems even learn from data on their own, bypassing the need for human-made training data, improving the verification of data exceptionally.
Importance of AI in Image Recognition
AI, with its image recognition technology, has transformed how data is managed in citizen science. It has expedited data processing and accuracy in projects, such as iNaturalist. Here, quick responses on submitted photos enhance biodiversity knowledge and scientific interest. AI is also integral in reducing errors in ecological monitoring, such as mistaking objects for animals. This allows more accurate identification, enriching scientific endeavors.
The field holds great potential for growth, particularly with the development of new AI tools and increased funding. This could lead to the widespread use of AI in citizen science, furthering research and conservation efforts. The table below briefly illustrates AI's impact on citizen science research.
Database | Total Articles | Articles on AI & Citizen Science |
---|---|---|
ACM DL | 92 | 50 |
IEEE Xplore | 8 | 6 |
The Role of Citizen Scientists in AI Development
The field of artificial intelligence (AI) is greatly impacted by the contributions of citizen scientists. Their efforts extend far beyond simple data collection. They are essential in improving AI's abilities through the analysis and validation of substantial datasets. This teamwork is especially critical in areas like wildlife preservation and ecological monitoring. The combination of public participation and AI knowledge significantly enhances our ability to evaluate and protect global ecosystems.
Take, for example, the success of the "Dark Energy Explorers" app, where citizens provided over 2 million classifications. These classifications were instrumental in teaching AI systems to recognize authentic galaxies in space. Similarly, the "Building Detective for Disaster Preparedness" project witnessed the contribution of over 20,000 labeled images in a short span by more than 1,000 volunteers. This rapid collective effort showcases the immense impact of citizen involvement in advancing AI technologies.
The Galaxy Zoo project, beginning in 2007, exemplifies this synergy. It engaged citizens in labeling astronomical data. This data, labeled by the public in 2007, was essential for training neural networks to classify millions of galaxy types nearly a decade later, in 2019. Such projects illustrate the power of collective intelligence in AI advancement.
Acknowledging the role of citizen scientists in AI also means recognizing their efforts to mitigate bias in datasets. Skewed data, as found in examples of AI detecting biased hate speech, can be corrected by a diverse group of citizen contributors. Their involvement introduces a variety of perspectives into AI development, promoting systems that are more fair and inclusive.
Furthermore, citizen involvement in platforms like iNaturalist, aiding with species identification, highlights their vital role in AI-based ecological studies. For instance, iNaturalist provided a set of 5,000 images in 2017 to train AI, thanks to its community's contributions. This demonstrates the significance of public participation in the creation of AI tools for monitoring the natural world.
Citizen scientists' support is also crucial in analyzing big data for research, as seen in Ben Goldstein's study on species reporting. His work at UC Berkeley, utilizing Jetstream analysis, showcases the fundamental role citizen scientists play in AI and big data research. Their contributions are key to gaining deep ecological insights and formulating relevant solutions.
Project | Type of Contribution | Data Volume |
---|---|---|
Dark Energy Explorers | Galaxy Classification | Almost 2 million classifications |
Building Detective for Disaster Preparedness | Image Annotation | 20,000 images |
Galaxy Zoo | Galaxy Data Labeling | Hundreds of millions of galaxies |
iNaturalist | Species Identification | 5,000 photographs |
Sentiment Detection | Tweet Labeling | Large-scale tweet datasets |
How You Can Get Started as a Citizen Scientist
Deciding to join citizen science ventures unveils both rewards and far-reaching effects. Your involvement in AI research projects will contribute to scientific growth. It will also shape the direction of technologies that significantly affect our lives.
Finding Projects to Join
The journey starts with finding the right project. Platforms like Zooniverse and iNaturalist host a wide array of projects. They range from ecological studies to AI research. These platforms will guide you to initiatives that pique your interest.
Understanding Project Requirements
Know the project's needs before immersing yourself. Each initiative will outline specific requirements. This could include data demands, expertise levels, and how often you should contribute. Understanding these details ensures your participation is not only helpful but also hits the project's targets.
Consider the 2019 "Building Detective for Disaster Preparedness" project. It drew over a thousand willing hands to swiftly annotate images. In the AI domain, projects require meticulously labeled datasets. They use vast repositories like ImageNet or iNaturalist's extensive collection of photos. These datasets are crucial for training computer-vision models.
Project | Year | Participants | Dataset Size | Impact |
---|---|---|---|---|
Building Detective for Disaster Preparedness | 2019 | 1,000+ | 20,000 images | High |
iNaturalist | 2017 | Ongoing | 5,000 photographs (initial dataset) | Continuing |
To excel in citizen science, commitment and insight into project principles are key. By selecting fitting projects and closely reviewing their requirements, your contribution to AI research will be significant. This approach strengthens the link between science and its public supporters.
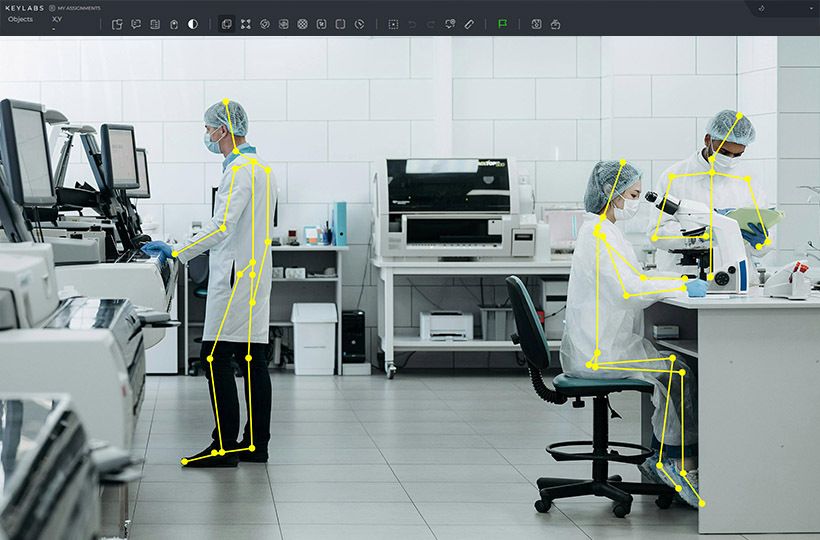
Examples of Successful Citizen Science Projects
Citizen science projects are playing a crucial role in uniting public passion with scientific progress, especially in environmental action through technology. These influential citizen science initiatives bring people together and use technology effectively in addressing environmental issues.
Penguin Watch
With a reach across the globe, Penguin Watch stands out. It calls on thousands of volunteers to examine photos of penguins in distant places like Antarctica. This 'crowd identification' process gathers important data. It helps scientists understand how climate change affects penguin populations.
Snapshots at Sea
Thanks to the support from Ernst & Young and Microsoft, Snapshots at Sea shines. This initiative relies on teams annotating ocean photos to support marine life conservation. The blend of corporate resources and cutting-edge AI underlines the power of partnerships in environmental endeavors.
Here is a comparison of these projects:
Project Name | Focus Area | Volunteers Involved | Data Collected/Annotations |
---|---|---|---|
Penguin Watch | Penguin Population Monitoring | Thousands | Millions of images analyzed |
Snapshots at Sea | Marine Conservation | Thousands | Thousands of oceanic images annotated |
These projects truly stand as models for influential citizen science initiatives. They underscore the vital role of collective data annotation in leveraging technology for the good of the environment.
Contributing to AI Development
Participation in AI research is key for upgrading AI mechanisms through joint work. Citizen scientists are vital in this by collecting and labeling data, improving machine learning models. The field's growth is seen in more private investments and research articles on artificial intelligence.
Data Collection and Annotation
In the realm of AI, data gathering is fundamental, and citizen scientists make a big impact by amassing large datasets required for teaching machine learning models effectively. The Koster Seafloor Observatory project is a prime example, showcasing the strength of public involvement. Moreover, these individuals validate data through their annotations, ensuring its dependability.
Key Metrics | Impact |
---|---|
Private sector investment growth | 30x increase from eight years prior to 2021 |
AI research publications | Doubled in the last decade |
Total potential economic impact by 2030 | $15.7 trillion |
AI impact on productivity | $6.6 trillion |
AI impact on consumption-side effects | $9.1 trillion |
Improving Machine Learning Models
By aiding in data annotation and refinement, citizen scientists bolster machine learning advancements. This approach tackles large datasets and spurs AI system development via a blend of human and AI tasks.
These efforts are crucial, seen in the uptick of computer science PhD students focusing on AI and the growth in AI-specific resources. Researchers are crafting new methods for AI to self-improve, highlighting its transformation power. The AI Impact Index, with a top impact of 5, illustrates AI's profound influence.
The Significance of Machine Learning Models in AI
Machine learning models stand at the core of AI, particularly in the field of image recognition. By mastering these models, you can greatly enhance your input into AI image processing projects. This exploration will delve into the essentials and real-world uses of these models in amping up image recognition capabilities.
Basics of Machine Learning Models
Grasping machine learning is key to understanding its role in AI. AI is the broad use of computers to mimic human thinking. However, machine learning is a specialized field within AI. It allows systems to identify patterns, make decisions, and improve over time by gathering data and experience. In this context, deep learning uses complex neural networks to uncover complicated patterns, enabling automated predictions.
Application in Image Recognition
Machine learning finds a crucial application in AI image processing. It combines natural language processing and computer vision to let AI handle tasks automatically, improve decision-making, and interact with customers through tools like chatbots. Notable applications include iNaturalist and Snapshot Serengeti, which use machine learning for tasks like wildlife identification.
Industry | Machine Learning Application |
---|---|
Banking | Detecting fraud, preventing cybersecurity threats, user identity authentication |
Healthcare | Improving patient outcomes, clinical decision support, predictive analytics |
Retail | Optimizing inventories, recommendation engines, enhanced customer experiences |
Manufacturing | Predictive maintenance, operational efficiency |
Education | Personalized learning, automated grading, student performance prediction |
Using machine learning and AI image processing strategically is transforming multiple sectors. With deep knowledge in machine learning, you become a valuable team member in enhancing image recognition tech. This is crucial for boosting scientific projects such as ecology studies.
Understanding Neural Network Architectures
Comprehending various neural network specifications is vital in deep learning applications. They stand as key elements in advancing AI, developing quickly beyond simple tasks. These networks now power visual search engines, recommendation systems, chatbots, and medical diagnostics, tackling sophisticated challenges.
At their core, these systems feature interconnected "neurons." These mimic the brain's activity. A neural network is usually composed of an input, hidden, and output layer. The hidden layers, vital for extracting features and processing data, prepare information. Finally, the output layer offers predictions based on these insights.
Types of Neural Networks
- Convolutional Neural Networks (CNNs): They excel in image analysis and language processing by detecting patterns. Notable models include AlexNet and VGG, showcasing their power.
- Recurrent Neural Networks (RNNs): Designed for sequence data, RNNs find use in understanding natural language and analyzing chronological information. LSTMs, a variation, boost their memory, further refining their abilities.
- Generative Adversarial Networks (GANs): By pitting a generator against a discriminator, GANs enhance video prediction and image creation. They exemplify the innovation in neural network design and application.
- Echo State Networks (ESNs): Offering a simpler and efficient approach, ESNs use sparsely connected layers. Their unique design, which trains only the output layer, manages complex data effectively.
- Transformer Neural Networks: Known for their prowess with unstructured data, transformers are key in natural language tasks. They've marked a milestone in handling vast datasets, elevating performance and scalability.
These varied neural network architectures play a crucial role in diverse AI projects, such as those aiming at image recognition and labeling. Projects like Galaxy Zoo and the Human Atlas rely on these complex models. As we delve deeper into AI, understanding these structures fosters better engagement and contribution to the field.
Data Preprocessing Techniques for AI
Data preprocessing is essential to ready datasets for AI learning. It involves data transformation and encoding for machine comprehension. Optimizing data this way ensures AI models work as intended. Let's explore key methods in detail.
Data cleaning is the first step, focusing on issues like missing values and outliers. It enhances dataset quality for AI model training by addressing noisy or incomplete data. This is critical for accurate predictions and model stability during the learning phase.
Data integration combines information from various sources into one, enhancing data quality. In fields like medical image detection, this step is vital. It creates a strong dataset for improved AI learning outcomes.
Transformation modifies data values or structures, preparing it for deep analysis. Generalization and normalization make data analysis-ready. This step is crucial for AI to understand and derive insights effectively from complex datasets.
Data reduction aims to keep essential data while making the dataset more manageable. It uses methods like data compression and attribute selection. Such processes ensure AI models train more efficiently without losing critical information.
Feature scaling standardizes independent variables for algorithm optimization. Scaling variations include Min-Max Scaler and Robust Scaler, used for consistent feature measurement. It’s essential for algorithms relying on feature distances to perform effectively.
Data quality assessment verifies dataset accuracy and completeness. It's a major part of data preprocessing, consuming about 80% of a practitioner’s time. This underscores its importance in the AI development process.
Preprocessing Step | Description | Importance |
---|---|---|
Data Cleaning | Filling missing values, smoothing noisy data, removing outliers | Improves data quality, prevents false predictions |
Data Integration | Merging data from multiple sources | Essential for comprehensive datasets, boosts AI training efficiency |
Data Transformation | Normalizing, generalizing data | Enables proper data analysis, improves image data preparation |
Data Reduction | Reducing dataset size while retaining key information | Enhances model performance, speeds up training |
Feature Scaling | Standardizing range of features | Ensures uniform measurement, crucial for distance-based algorithms |
Knowing and applying these techniques is crucial for effective AI model training. They help ensure AI systems work accurately and efficiently, leading to better image recognition and other advanced capabilities. Keylabs offers a wide range of tools for streamlining data labeling tasks.
AI Ethics and Safety in Citizen Science
The surge in AI research highlighted both its game-changing potential and the ethical challenges it poses. Citizen science began incorporating AI around 2000. With this integration, ensuring ethical participation and safety has become essential. For instance, projects like iNaturalist employ AI to identify species from images uploaded by users. Examining and addressing these critical aspects is of utmost importance.
Ensuring Ethical Participation
To ensure ethical AI contribution, adherence to established guidelines is crucial. This includes protecting the integrity and privacy of participants. Joining forces, researchers and citizen scientists must maintain transparency in data collection and usage. It’s important to secure informed consent and inform contributors about data handling. Platforms like iNaturalist, which relies heavily on user data, face a particular ethical challenge - maintaining data integrity and credibility. This underscores the platform's continuous commitment to ethical standards.
Safety Considerations
Engagement in citizen science mandates a broader view of safety, encompassing digital privacy and AI operation integrity. It's vital to implement strict safety procedures to prevent data abuse and assure the trustworthiness of AI models. Notably, the rapid increase in AI publications necessitates a parallel focus on rigorous safety measures. Such an approach protects contributors, bolsters the credibility of AI in citizen science, and ensures its beneficial use.
FAQ
What is citizen science?
Citizen science engages the public in significant scientific research, aiding in ecological monitoring and data analysis. It fosters contributions to diverse scientific inquiries.
How do I get started as a citizen scientist?
To begin, select projects aligning with your interests and understand the needed involvement. Various online platforms offer detailed guidance for engaging in impactful citizen science endeavors.
Why is AI important in image recognition?
AI leverages complex algorithms to revolutionize image recognition through machine learning models and neural networks. This innovation accelerates data processing and refines analysis accuracy.
How can citizen scientists contribute to AI development?
Citizen scientists play a critical role in AI advancement by collecting and annotating data. They refine machine learning models and assist in validating AI systems, enhancing their accuracy and reliability.
What are some examples of successful citizen science projects?
Examples include Penguin Watch and Snapshots at Sea. These projects highlight the profound effect of collective effort on ecological monitoring and data analysis in scientific research.
What are machine learning models, and why are they important?
These models are pivotal for AI, enabling systems to comprehend and learn from data. They are essential for tasks like image recognition, aiding in the precise identification and classification of objects.
What types of neural networks are used in AI projects?
Convolutional neural networks (CNNs) and generative adversarial networks (GANs) are common in citizen science. CNNs excel in image recognition while GANs offer strong capabilities in data synthesis.
What are data preprocessing techniques, and why are they important?
Data preprocessing includes cleaning and format adjustments for training AI models. It's crucial for ensuring the efficacy and precision of AI systems.
Why is AI ethics and safety important in citizen science?
Maintaining ethical standards and safety ensures the credibility of AI research in citizen science. Abiding by ethical principles and guarding privacy aligns contributions with societal values.
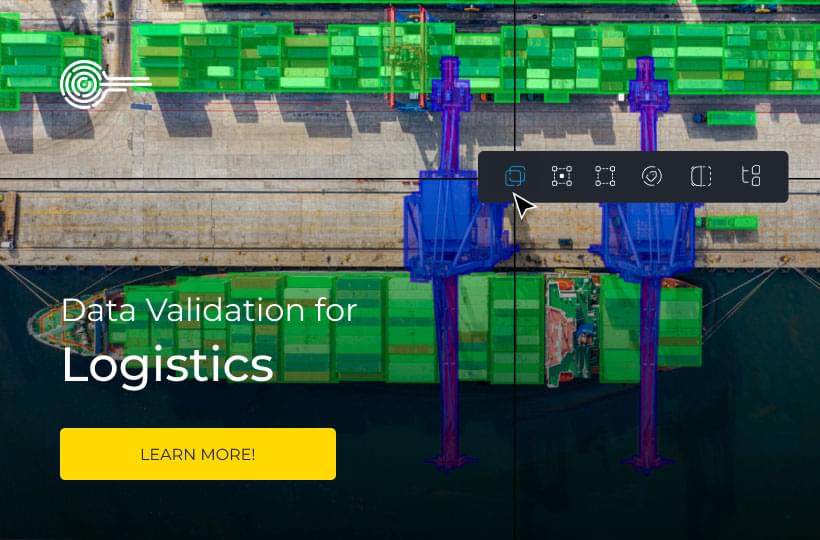