Data Privacy and the Ethical Use of AI
Data runs much of the world around us. Everything from social media platforms to lane-correcting vehicles needs constant sources of data to work properly. Data is undoubtedly going to be the major commodity of the twenty-first century. But that transition happened extremely quickly. The things we take for granted today would have been almost unthinkable even thirty years ago. The speed with which these things were invented comes with growing pains, mostly centering around two things: data privacy and ethics.
When people discuss the ethics of artificial intelligence algorithms, it often becomes a very emotional subject. People do not want their personal information to be given to technologies they do not understand. In addition, these platforms can have unclear security protocols and terms of use. AI is especially opaque when it comes to how data might be used. More than that, it’s often unclear what the timescale for potential problems will be. Addressing these problems is frequently the job of these tech companies, government regulators, and well-informed users.

What do data privacy and AI ethics look like?
There are a couple of different ways that people have chosen to address potential issues around the uses of data. Often, the first mover is governments who want to protect constituents from potential issues. These governments frequently impose restrictions on companies that embed them into user terms. These companies then communicate these terms to their users, who make decisions based on them. At each stage, important conversations must be had about what is necessary.
Government regulations around data privacy have lagged behind the introduction of data into major tech companies. The most significant policy ever implemented by a government is widely considered to be GDPR, implemented by the European Union. It established clear guidelines on how data could be used and how those guidelines must be communicated to users. Although this applies only to a small region of the world (the EU), it has become fairly standard across the internet.
The application of data privacy to artificial intelligence centers around the datasets used to train algorithms. The data annotation process requires sample datasets to be labeled with specific information. The labels that are put on user information mean that the companies are at liberty to impose their perspectives on these algorithms. Questions of ethics arise when it isn’t clear that these judgments are working in users' best interests. In addition, the complexity of these technologies creates fears about the ethical decisions being made.
Tools to implement data privacy and AI ethics in technology.
The data privacy industry has become a major sector in recent years. As was stated above, customers and users cannot trust tools that do not make clear their intentions to protect user data. Believing that these companies will act in users’ best interest will often not be enough. Some of the most significant ways this has been addressed include the following:
- Increased transparency
- Data consent
- Cybersecurity
- AI bias detection
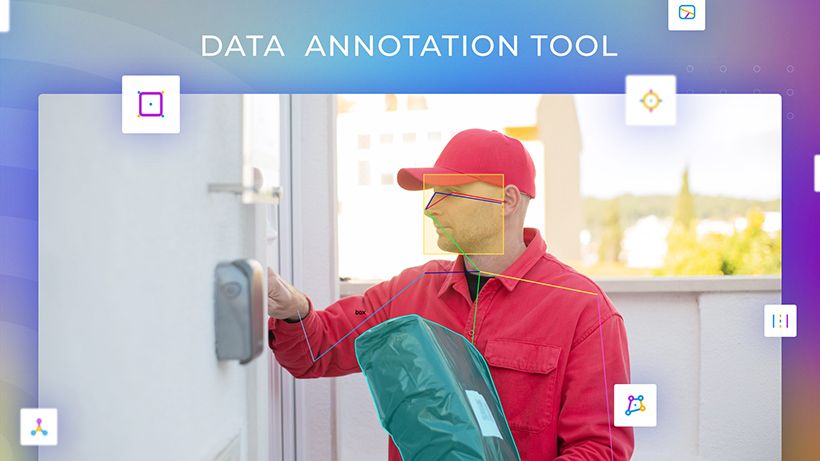
Transparency refers to a comprehensive suite of tools that make clear how and when user data is being used. This can come in many forms. The most basic is notices that inform users when their data is being collected. Many of the biggest tech companies have embedded this directly into their products. For example, the collection of cookies on certain websites is frequently used by AI systems to make recommendations. However, in the early days of the internet, it was difficult to know when these were being collected. Transparency measures prevent this from happening.
Tied closely to the idea of transparency is the idea of data consent. This was one of the major contributions of GDPR to the internet. Data consent doesn’t just allow people to choose when they want to send their data. Proper data consent also must give users the ability to opt out of data collection altogether. Removing the coercive ability of websites to force users to give up data in order to use their website was a major part of the law. Often, websites will allow users to choose what uses of their data they are comfortable with.
Cybersecurity is also a rapidly growing area in the world of data privacy. Data leaks have proven to be extremely destructive to these companies. They erode user trust and harm the industry as a whole. Countless companies today use tools like AI to ensure that the data they are collecting does not get to the wrong people. Trustworthy cybersecurity processes can make all the difference for these companies.
Lastly, AI bias detection can improve the fairness of AI systems. Humans are prone to impose their own biases on the systems they create. These are man-made tools, after all. AI detection is one strategy that can be used to prevent these automated systems from making unjust predictions or recommendations. Although not foolproof, they can improve their trust in the ethical soundness of the tools they are using.
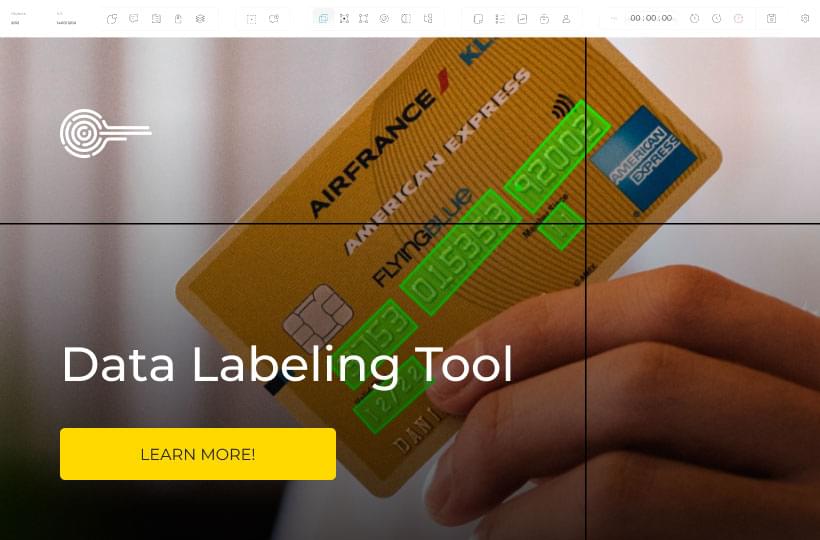
What does this mean for you?
Fears around the immorality of tech companies are often overstated. However, instances where data has leaked or destructive decisions have been made justify people feeling that way. Companies adhering to regulations or self-regulating from the start can meaningfully engage with these fears. This dialogue is at the center of current thinking about data privacy in tech. It’s fundamentally a question of trust. Without some way to codify that trust, people will continue to be fearful of these technologies.
At the end of the day, a completely trustworthy technology can’t exist. Maintaining a constant and honest dialogue with users is the only way to gain users' trust. Mistakes must be acknowledged and addressed quickly. Data privacy benefits both consumers and companies. Data annotation processes need to engage with each of these layers from the start. Ultimately, the success of your algorithms will be defined by the trust users have in the decisions they are making.