Expanding Horizons: Applications of Instance Segmentation
Instance segmentation is a groundbreaking technology in the field of computer vision, fueled by the advancements in deep learning, image processing, and artificial intelligence. It enables precise object identification and differentiation, revolutionizing the way we analyze and understand visual data. By accurately delineating individual objects of the same type, instance segmentation opens up a world of possibilities across various industries.
Whether it's autonomous vehicles navigating complex environments or medical professionals analyzing medical images, instance segmentation offers unparalleled solutions. Its ability to provide pixel-level accuracy and real-time tracking makes it an invaluable tool for visual recognition and object detection tasks.
In this article, we will explore the ins and outs of instance segmentation, delving into its inner workings, applications, and future potential. Join us on this journey to discover how instance segmentation is reshaping industries and paving the way for AI-powered image analysis.
Key Takeaways:
- Instance segmentation is a revolutionary technology in computer vision that allows for precise object identification and differentiation.
- It is powered by deep learning algorithms, image processing techniques, and artificial intelligence, enabling pixel-level accuracy and real-time tracking.
- Applications of instance segmentation span various industries, including autonomous vehicles, medical imaging, and more.
- This transformative technology has the potential to reshape processes and improve outcomes in diverse fields.
- As technological advancements continue, instance segmentation's capabilities are expected to expand, opening up new possibilities for AI-powered image analysis.

Instance Segmentation vs Semantic Segmentation
Instance segmentation and semantic segmentation are two powerful techniques used to analyze images in the field of computer vision. While they share the common goal of understanding and interpreting visual data, they differ in their approach and output.
Semantic segmentation is a process that involves classifying each pixel in an image into a specific category or class. It provides a pixel-wise label for different regions, enabling the identification and grouping of similar objects or elements. This technique is valuable in applications where understanding the overall scene or context is crucial, such as autonomous driving or scene understanding.
Instance segmentation, on the other hand, goes a step further by not only labeling pixels but also differentiating between individual objects of the same type. It provides precise delineation and accurate boundaries for each instance, allowing for a more detailed analysis of the image. This technique is particularly useful in applications where object differentiation is essential, such as object tracking, image editing, or robotics.
To better illustrate the differences, consider the example of segmenting a street scene with multiple cars. Semantic segmentation would label all the cars with the same class, providing a single category for all the pixels corresponding to cars. In contrast, instance segmentation would distinguish between each car separately, assigning a unique label for each instance and precisely delineating their boundaries.
Instance segmentation's ability to differentiate between objects at a pixel level sets it apart from semantic segmentation in terms of accuracy and specificity. It allows for more precise object delineation, enabling advanced applications that require detailed object recognition, pixel-level accuracy, and precise analytical insights.
"Instance segmentation provides not only pixel-level accuracy but also the ability to distinguish between individual objects of the same type, which is crucial in many practical scenarios."
Instance Segmentation vs Semantic Segmentation: A Comparison
Aspect | Instance Segmentation | Semantic Segmentation |
---|---|---|
Objective | Differentiate between individual objects, provide precise delineation and accurate boundaries. | Classify pixels into different categories, identify regions of similar objects. |
Output | Pixel-wise labeling with distinct labels for each object instance. | Pixel-wise labeling with categories assigned to regions. |
Applications | Object tracking, image editing, robotics. | Autonomous driving, scene understanding, image segmentation. |
Benefit | Precise object differentiation, pixel-level accuracy. | Contextual understanding, scene analysis. |
As shown in the comparison table, instance segmentation and semantic segmentation serve different purposes and are employed in diverse applications. While semantic segmentation offers a higher-level understanding of the scene, instance segmentation provides the detailed object-level analysis required for many real-world scenarios.
How Does Instance Segmentation Work?
Instance segmentation, a fundamental technique in computer vision, operates through a series of steps that involve feature extraction, region proposal, object classification, and mask prediction. By leveraging deep learning models, such as Convolutional Neural Networks (CNNs), instance segmentation achieves pixel-level accuracy and detailed object recognition.
First, the process starts with feature extraction, where the deep learning model analyzes the image and captures essential visual patterns and characteristics. These features serve as the foundation for identifying and distinguishing objects within the image.
Next, the model proposes potential regions in the image that may contain objects. This region proposal step helps segment the image into meaningful regions of interest, eliminating non-relevant areas and focusing on object boundaries.
After region proposal, the model performs object classification, where each proposed region is classified into specific object categories. By trained models, the algorithm is capable of distinguishing between different object types and assigning accurate labels to each region.
Finally, the instance segmentation model predicts precise masks for each object, outlining their boundaries and separating them from the background. These masks provide a detailed representation of the object's shape and size, enabling precise identification and delineation.
By combining the stages of feature extraction, region proposal, object classification, and mask prediction, instance segmentation achieves pixel-level accuracy and a granular understanding of object boundaries. This level of detail allows for various applications, from autonomous driving systems that can accurately detect and classify different objects on the road to medical imaging where tumors can be analyzed in detail for personalized treatment plans.
"Instance segmentation operates through a series of steps that involve feature extraction, region proposal, object classification, and mask prediction."
Applications of Instance Segmentation
Instance segmentation, with its ability to accurately identify and delineate individual objects, finds diverse applications across multiple sectors. Let's explore some of the key industries where instance segmentation is making a significant impact:
1. Healthcare
In the healthcare industry, instance segmentation is revolutionizing precision diagnostics and treatment. By accurately identifying and analyzing tumor cells, instance segmentation enables personalized therapies for cancer patients. It plays a crucial role in detecting irregularities, assisting doctors in the early identification and monitoring of diseases. With its pixel-level accuracy, instance segmentation aids in precise localization and analysis of medical images, leading to improved patient outcomes.
2. Autonomous Driving
In the automotive industry, instance segmentation plays a vital role in enhancing the capabilities of autonomous driving systems. By accurately detecting and classifying objects on the road, such as vehicles, pedestrians, and traffic signs, instance segmentation ensures the safety and reliability of autonomous vehicles. Real-time tracking and precise delineation of objects enable autonomous vehicles to make informed decisions, improving overall road safety.
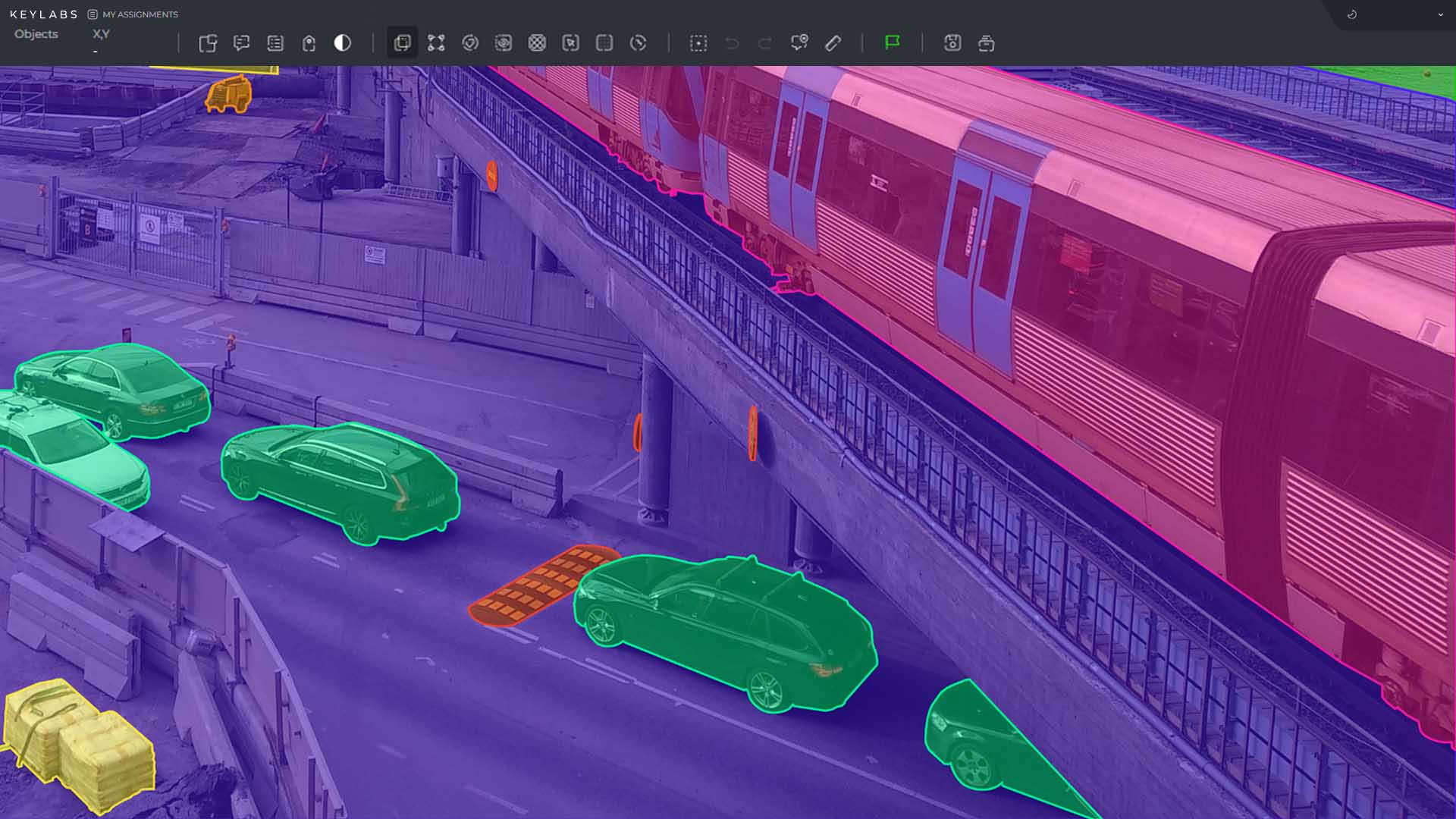
3. Retail
Instance segmentation brings significant benefits to the retail sector by improving customer experiences and optimizing inventory management. By accurately tracking and identifying items, instance segmentation enables efficient stock management and inventory optimization, reducing wastage and enhancing cost-effectiveness. Moreover, it facilitates personalized customer recommendations and targeted advertising, enhancing customer satisfaction and boosting sales.
4. Agriculture
In agriculture, instance segmentation enables precision farming practices. By identifying and managing weeds among crops, instance segmentation allows farmers to selectively target and eliminate weeds, reducing the need for herbicides and promoting sustainable farming. It helps optimize resource allocation by providing insights into crop health, enabling farmers to take corrective measures in a timely manner, and maximizing crop yields.
These are just a few examples of the wide-ranging applications of instance segmentation. Its ability to precisely delineate and differentiate objects has the potential to revolutionize processes, improve outcomes, and drive innovation in various industries.
Industry | Applications |
---|---|
Healthcare | Precision diagnostics, personalized therapies |
Autonomous Driving | Object detection, road safety |
Retail | Inventory management, customer experience |
Agriculture | Precision farming, weed management |
Future Trajectory and Potential
The future trajectory of instance segmentation holds immense promise, fueled by ongoing technological advancements and the ever-expanding range of industry applications. As computational power and machine learning algorithms continue to improve, instance segmentation is poised to become even more accurate, efficient, and versatile, revolutionizing the field of AI-powered image analysis.
One of the key factors driving the future potential of instance segmentation is the constant evolution of technological advancements. As hardware capabilities improve, with the emergence of more powerful processors and GPUs, the computational power required for instance segmentation algorithms increases, enabling faster processing and more complex analyses.
Furthermore, the development of more sophisticated machine learning algorithms and neural network architectures enhances the performance and capabilities of instance segmentation models. The ability to detect and segment objects with pixel-level accuracy has significant implications across numerous industries where precise object identification and delineation are crucial.
The potential applications of instance segmentation extend far beyond the current industries it serves. For example, in environmental monitoring, instance segmentation can be utilized to accurately identify and track specific features, such as wildlife or vegetation, enabling effective conservation efforts. In urban planning, instance segmentation can play a pivotal role in analyzing public spaces, identifying potential hazards, and optimizing infrastructure design for enhanced safety and livability.
The transformative impact of instance segmentation is not limited to a specific domain. Its ability to analyze images and videos in real-time with pixel-level accuracy opens up a world of possibilities across various fields. From healthcare and manufacturing to agriculture and transportation, the applications of instance segmentation are vast and diverse.
Potential Industry Applications of Instance Segmentation
Industry | Applications |
---|---|
Healthcare | - Tumor segmentation for personalized therapies - Organ and anomaly detection in medical imaging |
Manufacturing | - Quality control and defect detection in production processes - Object detection and tracking in robotic systems |
Agriculture | - Weed detection and crop management for precision farming - Yield estimation and disease identification |
Transportation | - Object detection and tracking for autonomous vehicles - Traffic flow analysis and congestion management |
The versatility and potential of instance segmentation make it a driving force behind innovative solutions in the rapidly evolving landscape of AI-powered image analysis. As its accuracy, speed, and applications continue to expand, instance segmentation is set to shape the future of industries, enabling enhanced decision-making, automation, and efficiency.
Conclusion
Instance segmentation applications have had a transformative impact on various industries, revolutionizing the field of AI-powered image analysis. The precision and accuracy offered by instance segmentation have opened up new possibilities and driven innovation across sectors such as healthcare, autonomous driving, retail, and agriculture.
In healthcare, instance segmentation has fostered personalized therapies by accurately identifying and analyzing tumor cells, leading to more precise diagnostics and treatment. In the automotive industry, it has significantly enhanced the capabilities of autonomous driving systems, enabling the detection and classification of objects on the road in real-time, ensuring safety and reliability.
Instance segmentation has also made its mark in the retail sector, where it optimizes inventory management and improves customer experiences. By accurately tracking items and optimizing stock levels, it streamlines operations and enhances overall efficiency. Additionally, in agriculture, instance segmentation supports precision farming practices by identifying and managing weeds among crops. This targeted approach increases crop yields and reduces the use of harmful herbicides.
As technological advancements continue to evolve, instance segmentation's transformative impact is expected to grow. Its broad range of applications, from healthcare to autonomous driving, and its ability to provide precise object differentiation and delineation make it an invaluable tool in shaping the future of AI-powered image analysis. With its transformative potential and ongoing innovations, instance segmentation is set to play a significant role in driving further advancements in the field.
FAQ
What is instance segmentation?
Instance segmentation is a revolutionary advancement in computer vision that allows for the precise delineation of objects in images.
How does instance segmentation differ from semantic segmentation?
Semantic segmentation classifies each pixel in an image into a category, while instance segmentation not only labels the pixels but also distinguishes between individual objects of the same type.
What are the steps involved in instance segmentation?
Instance segmentation involves feature extraction, region proposal, object classification, and mask prediction to achieve pixel-level accuracy and detailed object recognition.
What are some applications of instance segmentation?
Instance segmentation has applications in healthcare, autonomous driving, retail, and agriculture, among others.
What is the future trajectory of instance segmentation?
As technological advancements continue to evolve, instance segmentation is expected to become even more accurate and efficient, with potential applications in various fields.
What is the impact of instance segmentation on AI-powered image analysis?
Instance segmentation drives innovation and provides transformative capabilities in AI-powered image analysis, improving outcomes and processes across industries.
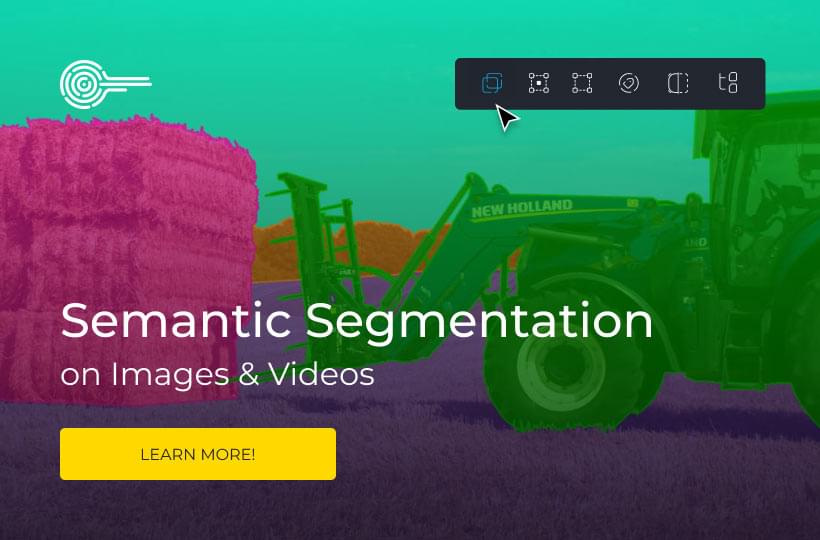