Exploring Applications of SemanticSegmentation
Semantic segmentation is a powerful technique in the field of computer vision that involves dividing an image into meaningful segments or regions. Its applications span across various industries and have transformative effects on machine learning technologies. By accurately labeling each pixel in an image with a specific class, semantic segmentation enables advanced image analysis, object recognition, and scene understanding. In this article, we will explore the different applications and use cases of semantic segmentation, highlighting its role in machine learning advancements.
Key Takeaways:
- Semantic segmentation divides an image into meaningful segments or regions.
- It plays a crucial role in autonomous driving, medical image analysis, remote sensing, augmented reality, and fashion industries.
- It enables object recognition, scene understanding, and advanced image analysis.
- Semantic segmentation improves the accuracy and reliability of machine learning technologies.
- As technology evolves, semantic segmentation will continue to drive innovation in various industries.

Semantic Segmentation in Autonomous Driving
Semantic segmentation plays a crucial role in enabling autonomous vehicles to perceive and understand their surroundings. By segmenting the pixels in an image into different classes such as road, vehicles, pedestrians, and obstacles, semantic segmentation helps self-driving cars navigate safely on the road. It allows the vehicle to detect lane boundaries, identify traffic signs and signals, and make real-time decisions based on the segmented information. With semantic segmentation, autonomous driving systems can achieve higher levels of accuracy and reliability, leading to safer and more efficient transportation.
One of the key challenges in autonomous driving is the ability to accurately detect and understand the road environment. Semantic segmentation provides a solution to this challenge by dividing the image into meaningful segments, allowing the autonomous vehicle to differentiate between different objects and road attributes. For example, by segmenting the image, the self-driving car can identify the road surface, the location of other vehicles, and the presence of pedestrians or obstacles.
The benefits of semantic segmentation in autonomous driving are numerous. It enables the vehicle to accurately and effectively perceive its surroundings, making informed decisions in real-time. By understanding the road environment through semantic segmentation, self-driving cars can navigate complex traffic scenarios, adapt to changing road conditions, and ensure the safety of passengers and other road users.
Autonomous driving systems rely on semantic segmentation for various critical tasks:
- Detecting and tracking lane boundaries
- Recognizing traffic signs and signals
- Differentiating between vehicles, pedestrians, and obstacles
- Identifying road markings and infrastructure
By accurately segmenting the scene, semantic segmentation provides precise information that enables the autonomous vehicle to make informed decisions. It serves as the foundation for advanced perception algorithms, artificial intelligence systems, and machine learning models used in self-driving cars.
In conclusion, semantic segmentation in autonomous driving plays a fundamental role in enabling self-driving cars to perceive and navigate the road environment. Through the accurate segmentation of the visual input, semantic segmentation empowers autonomous vehicles to make real-time decisions and ensures the safety and efficiency of transportation systems.
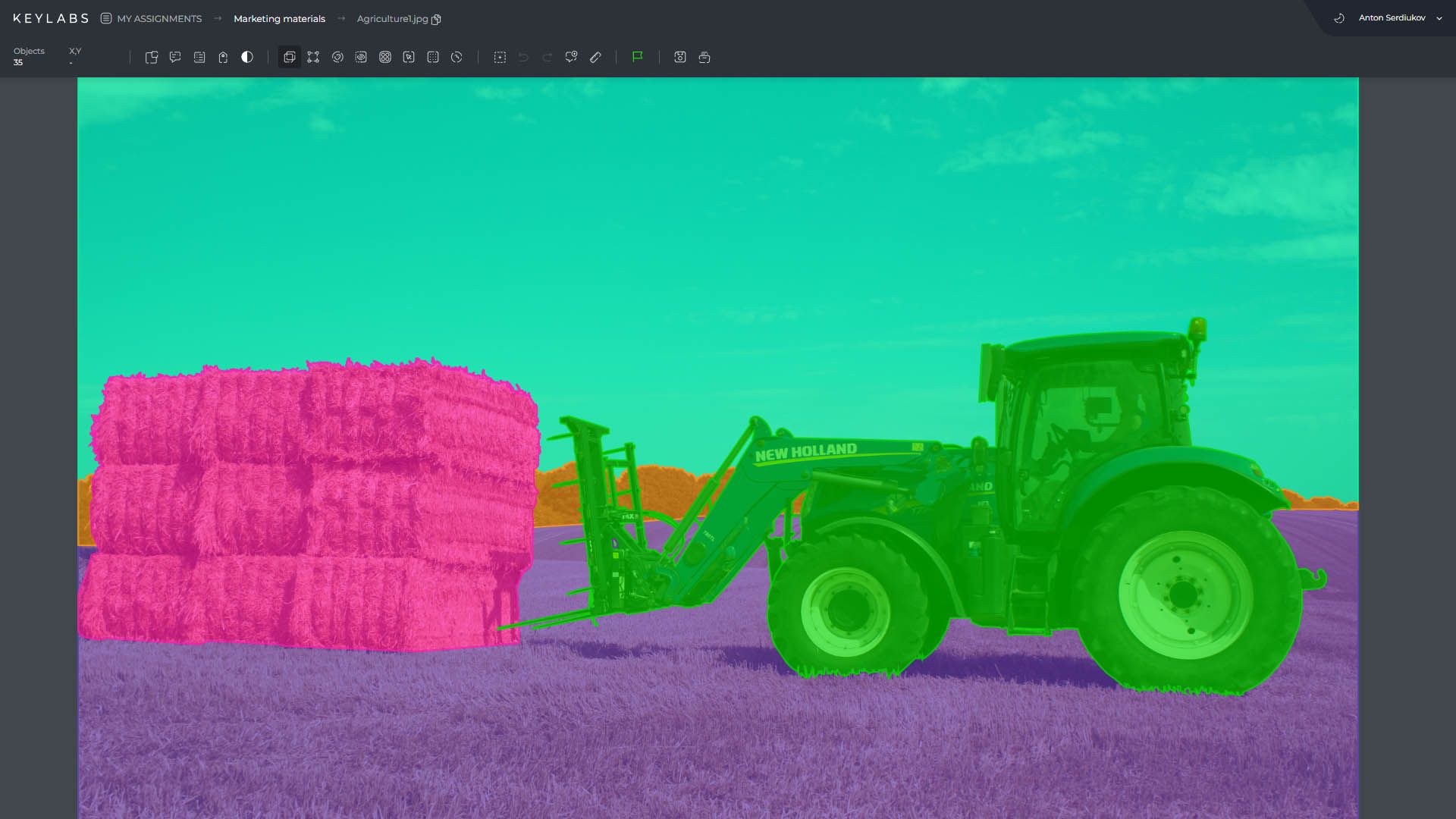
Semantic Segmentation in Medical Image Analysis
Semantic segmentation has revolutionized medical image analysis by enabling accurate and precise detection of abnormalities and diseases. By segmenting medical images, such as MRI scans or X-rays, into different anatomical structures or regions of interest, semantic segmentation assists in the diagnosis and treatment of various medical conditions. It allows for the identification of tumors, detection of lesions, and mapping of organs and tissues. This technology has greatly improved the efficiency and accuracy of medical imaging, leading to better patient care and outcomes.
One of the key applications of semantic segmentation in medical imaging is disease detection. By accurately segmenting images, medical professionals can identify diseased areas and anomalies with greater precision. This enables early detection and diagnosis of conditions such as cancer, cardiovascular diseases, and neurological disorders. Semantic segmentation provides a valuable tool for clinicians to analyze medical images and make informed decisions regarding treatment plans.
Tumor segmentation is another important area where semantic segmentation plays a crucial role. By segmenting medical images, radiologists can accurately identify and delineate tumor boundaries. This information is essential for planning surgeries, radiation therapy, and monitoring tumor growth over time. Semantic segmentation techniques provide valuable insights into the size, location, and characteristics of tumors, aiding in effective treatment strategies.
In addition to disease detection and tumor segmentation, semantic segmentation is also used in medical research and drug development. It helps researchers analyze large datasets of medical images to gain insights into disease progression, treatment response, and drug efficacy. Semantic segmentation algorithms can be trained to identify specific biomarkers or abnormalities, aiding in the discovery of new diagnostic tools and therapeutic targets.
Table: Applications of Semantic Segmentation in Medical Image Analysis
Application | Benefits |
---|---|
Disease detection | Early diagnosis, precise localization of abnormalities |
Tumor segmentation | Accurate tumor boundary delineation, treatment planning |
Medical research | Data analysis, biomarker identification, drug development |
Overall, semantic segmentation has revolutionized medical image analysis, offering new possibilities for disease detection, tumor segmentation, and medical research. Its use in healthcare has significantly improved the accuracy and efficiency of diagnosing and treating various conditions. As technology continues to advance, semantic segmentation is expected to further enhance medical imaging capabilities, enabling even more precise and personalized patient care.
Semantic Segmentation in Remote Sensing and Geospatial Applications
Semantic segmentation has revolutionized the field of remote sensing and geospatial analysis. By utilizing advanced algorithms, this technique enables the accurate segmentation of satellite or aerial images, providing valuable insights for various applications such as land cover classification, urban planning, agriculture, and environmental monitoring.
One of the key applications of semantic segmentation in remote sensing is land cover classification. By dividing an image into different classes based on land-use types, such as forests, water bodies, and urban areas, semantic segmentation allows for detailed and accurate mapping of land cover. This information is vital for urban planning, environmental management, and assessing changes in the landscape over time.
Another significant application of semantic segmentation in remote sensing is monitoring vegetation. By segmenting satellite imagery, scientists and researchers can analyze the health and distribution of vegetation in a specific area. This data is crucial for ecological studies, agriculture, and assessing the impact of climate change on plant life.
Semantic segmentation also plays a crucial role in assessing environmental changes. By segmenting aerial or satellite images, it becomes possible to identify and analyze changes in land cover, water bodies, and other environmental features over time. This information is invaluable for monitoring deforestation, urban expansion, and other environmental phenomena.
Overall, semantic segmentation in remote sensing and geospatial applications offers a powerful tool for analyzing and understanding large-scale geographical data. By providing detailed and accurate segmentation of images, it facilitates effective decision-making in various fields, ensuring sustainable land management, environmental conservation, and resource optimization.
Applications of Semantic Segmentation in Remote Sensing
Application | Description |
---|---|
Land Cover Classification | Accurately classifying land-use types, such as forests, water bodies, and urban areas, for urban planning and environmental management. |
Vegetation Monitoring | Assessing the health and distribution of vegetation in a specific area for ecological studies and agriculture. |
Environmental Change Detection | Identifying and analyzing changes in land cover, water bodies, and other environmental features over time for monitoring and conservation purposes. |
Semantic Segmentation in Augmented Reality and Virtual Reality
Semantic segmentation plays a vital role in creating immersive augmented reality (AR) and virtual reality (VR) experiences. By accurately segmenting the objects in a real-world scene, semantic segmentation enables virtual object placement, occlusion handling, and realistic interaction between real and virtual elements. It enhances the visual quality and realism of AR/VR applications by providing accurate depth information and object recognition capabilities. Semantic segmentation is fundamental in creating seamless and interactive virtual environments that merge with the real world.
Applications | Benefits |
---|---|
Virtual Object Placement | Allows virtual objects to be convincingly integrated into the real world. |
Occlusion Handling | Enables virtual objects to be properly occluded by real objects, enhancing realism. |
Realistic Interaction | Facilitates natural interaction between real and virtual elements. |
Accurate Depth Information | Provides precise depth perception, improving the overall visual quality. |
Object Recognition | Enables AR/VR systems to identify and interact with real-world objects. |
By leveraging semantic segmentation, AR/VR applications can create compelling and immersive experiences that blur the line between the physical and virtual realms. Users can interact with virtual objects in a lifelike manner, making AR/VR more engaging and realistic. Whether it's placing virtual furniture in a real room or overlaying virtual information onto a real-world scene, semantic segmentation enhances the visual quality and authenticity of these experiences.
"Semantic segmentation in AR/VR empowers developers and users alike to unleash the full potential of mixed reality technologies. With accurate object placement and occlusion handling, virtual elements seamlessly blend into the real world, enabling transformative experiences across various industries."
Advancements in Object Recognition
One of the key benefits of semantic segmentation in AR/VR is the ability to recognize and interact with real-world objects. By segmenting the scene and identifying objects, AR/VR systems can provide contextual and relevant information. For example, a healthcare AR app can recognize medical instruments and overlay information about their usage, enhancing surgical training or medical procedures. Similarly, a retail AR app can recognize products and display pricing or customer reviews, enabling virtual shopping experiences.
With continued advancements in semantic segmentation algorithms and hardware capabilities, the accuracy and efficiency of object recognition in AR/VR will continue to improve. This opens up new possibilities for interactive and personalized experiences in fields such as gaming, education, healthcare, and more.
Semantic Segmentation in Fashion and E-commerce
Semantic segmentation is a powerful tool that has found extensive applications in the fashion industry and e-commerce platforms. By leveraging semantic segmentation in fashion, companies can enhance clothing recognition and recommendation systems, thereby improving the overall shopping experience for users.
Through semantic segmentation, clothing items in images can be accurately segmented, enabling a detailed analysis of different garment attributes, including color, pattern, and style. This technology plays a crucial role in providing personalized fashion recommendations, virtual try-on experiences, and efficient product categorization.
With the help of semantic segmentation in fashion, e-commerce platforms can offer users a tailored shopping experience, ensuring that they find the products that best suit their preferences and needs. By accurately understanding and categorizing clothing items based on their segmented attributes, fashion recommendation systems can suggest relevant and stylish options to users, enhancing their decision-making process.
In addition, semantic segmentation in fashion enables virtual try-on experiences, allowing users to digitally visualize how clothing items will look on them without physically trying them on. By accurately segmenting and overlaying virtual objects onto real scenes, users can gain a realistic preview of how the garments will fit and suit their personal style.
Benefits of Semantic Segmentation in Fashion and E-commerce
The utilization of semantic segmentation in fashion and e-commerce brings several benefits to both businesses and consumers:
- Enhanced clothing recognition: Semantic segmentation allows for accurate and detailed analysis of various garment attributes, improving clothing recognition and identification within images.
- Personalized fashion recommendations: By segmenting clothing items and understanding their attributes, fashion recommendation systems can suggest personalized and relevant options to users, leading to more satisfactory shopping experiences.
- Virtual try-on experiences: Semantic segmentation enables virtual try-on experiences, allowing users to visualize how garments will look on them without physically trying them on, thereby reducing the uncertainty of online shopping.
- Efficient product categorization: With semantic segmentation, e-commerce platforms can efficiently categorize clothing items based on their characteristics, facilitating easy navigation and search for users.
By leveraging semantic segmentation in fashion and e-commerce, businesses can provide a more engaging and interactive shopping experience, enabling users to make informed decisions and find the perfect clothing items that match their preferences and style.
Conclusion
Semantic segmentation, an advanced technique in computer vision, has revolutionized various industries by transforming how machines perceive and analyze visual data. From autonomous driving to medical imaging, remote sensing to fashion, the applications of semantic segmentation have reshaped the capabilities of AI systems.
By accurately dividing images into meaningful regions, semantic segmentation enables advanced analysis, object recognition, and scene understanding. It plays a crucial role in autonomous driving by helping self-driving cars navigate safely, detect lane boundaries, and make real-time decisions on the road. In medical imaging, semantic segmentation aids in the detection of diseases and mapping of anatomical structures, improving diagnostic accuracy.
With the continuous advancement of technology, semantic segmentation is poised to become even more significant in machine learning and computer vision. Its implementation will drive further innovation and enhance the capabilities of AI systems across industries. From augmented reality and virtual reality experiences to fashion and e-commerce platforms, semantic segmentation enhances object recognition and recommendations, improving user experiences and decision-making processes.
In conclusion, semantic segmentation is a powerful technique with diverse applications that have transformed industries such as autonomous driving, medical imaging, remote sensing, fashion, and more. Its development and implementation will continue to propel advancements in machine learning and computer vision, enabling machines to perceive and understand visual data with greater precision and accuracy.
FAQ
What is semantic segmentation?
Semantic segmentation is a technique in computer vision that involves dividing an image into meaningful segments or regions and labeling each pixel with a specific class, allowing for advanced image analysis, object recognition, and scene understanding.
What are the applications of semantic segmentation?
Semantic segmentation has applications in autonomous driving, medical image analysis, remote sensing and geospatial analysis, augmented reality and virtual reality, and fashion and e-commerce industries.
How does semantic segmentation benefit autonomous driving?
Semantic segmentation helps self-driving cars perceive and understand their surroundings by segmenting the pixels in an image into different classes such as road, vehicles, pedestrians, and obstacles. It enables lane detection, object recognition, and real-time decision-making for safer and more efficient transportation.
How does semantic segmentation contribute to medical image analysis?
Semantic segmentation assists in the accurate detection of abnormalities and diseases in medical images by segmenting them into different anatomical structures or regions of interest. It improves the diagnosis, treatment, and patient care by identifying tumors, detecting lesions, and mapping organs and tissues.
What is the role of semantic segmentation in remote sensing and geospatial analysis?
Semantic segmentation in remote sensing allows for the detailed analysis of large-scale geographical data by segmenting satellite or aerial images. It aids in land cover classification, mapping urban areas, monitoring vegetation, and assessing environmental changes, providing valuable information for urban planning, agriculture, and environmental management.
How does semantic segmentation enhance augmented reality and virtual reality experiences?
Semantic segmentation accurately segments objects in real-world scenes, enabling realistic virtual object placement, occlusion handling, and interaction between real and virtual elements. It improves the visual quality, depth perception, and object recognition capabilities in augmented reality and virtual reality applications, creating immersive and interactive virtual environments.
How does semantic segmentation benefit the fashion and e-commerce industry?
Semantic segmentation in the fashion industry and e-commerce platforms enhances clothing recognition and recommendation systems by accurately segmenting clothing items in images. It allows for the analysis of attributes like color, pattern, and style, leading to personalized fashion recommendations, virtual try-on experiences, and efficient product categorization.
Conclusion
Semantic segmentation is a powerful technique with numerous applications that have transformed various industries. From autonomous driving to medical imaging, remote sensing to fashion, semantic segmentation has revolutionized how machines perceive and understand visual data. By accurately segmenting images into meaningful regions, semantic segmentation enables advanced analysis, object recognition, and scene understanding. As technology continues to evolve, semantic segmentation is expected to play an even more significant role in machine learning advancements and computer vision applications. Its continued development and implementation will drive further innovation and improve the capabilities of AI systems across industries.
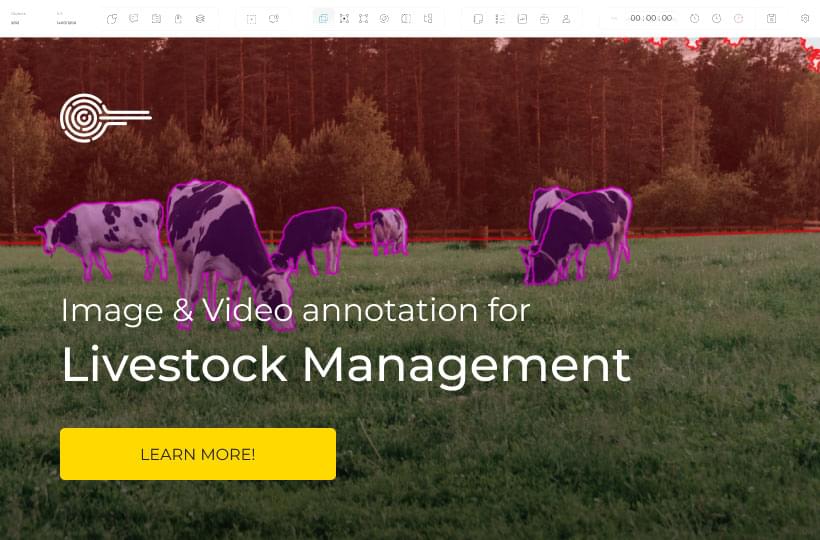