How AI-Powered Data Annotation is Revolutionizing Precision Farming
Precision agriculture technologies, like drones and sensors, offer real-time data. When annotated, this data trains AI models to make precise decisions. This approach not only improves traditional farming but also leads to sustainable outcomes. It addresses challenges like climate change and food security.
For more context, this article highlights the importance of AI in farming as a whole.
Key Takeaways
- Precision agriculture technologies are transforming farming through AI, drones, and soil sensors.
- Data annotation is essential for accurate AI model training in agriculture, enabling meaningful data interpretation.
- Real-time insights from annotated data facilitate early disease detection and timely interventions.
- Yield prediction is significantly enhanced through machine learning models trained on annotated data.
- High-quality data annotation drives sustainable farming, operational efficiency, and profitability.
- AI-driven drones in agriculture optimize pesticide use, conserving the environment.

Understanding Crop Monitoring Annotation
In the realm of precision farming, accurate crop monitoring annotation is key to effective AI models. It involves labeling various agricultural data types, like drone and satellite imagery, sensor outputs, and field surveys. Detailed labeling enhances AI's understanding and interpretation of farm data, leading to smarter agricultural practices.
What is Crop Monitoring Annotation?
Crop monitoring annotation is a detailed process of tagging and identifying elements in agricultural data sets. This includes:
- Satellite and UAV (Unmanned Aerial Vehicle) imagery for extensive crop health analysis.
- Close-up plant images from UGVs (Unmanned Ground Vehicles) to detect diseases.
- Data from IoT sensors to measure environmental conditions.
The main goal is to create reliable datasets for AI models to understand and predict agricultural phenomena. These annotated datasets enable AI to manage tasks like ripeness detection, pest identification, and yield optimization.
Importance of Accurate Data in Farming
The accuracy of annotations is critical for AI tools in precision farming. High-precision data labeling ensures accurate tasks such as crop health analysis, yield prediction, and resource allocation. This leads to:
- Improved Decision-Making: Accurate data provides insights for informed decisions on planting, irrigating, and harvesting.
- Enhanced Productivity: Identifying issues early, like disease outbreaks or nutrient deficiencies, allows farmers to take timely actions, improving yields.
- Cost Efficiency: AI technologies reduce resource wastage, saving costs while maintaining crop quality.
An accurate crop health analysis, backed by robust agricultural imagery annotation, modernizes farming. It enables a sustainable and productive agricultural system. Detailed and precise data labeling is essential for AI's full agricultural impact.
Aspect | Traditional Methods | AI-Powered Methods |
---|---|---|
Data Collection | Manual, Time-intensive | Automated, Efficient with UAVs and Sensors |
Data Accuracy | Prone to Human Error | High Precision with Machine Learning Models |
Decision-Making | Based on Experience | Data-Driven Insight and Predictions |
Resource Allocation | Generalized Application | Targeted, Efficient Use of Resources |
The Role of AI in Agriculture
Artificial Intelligence (AI) is transforming the farming industry, setting new standards in efficiency and productivity. AI-driven agriculture solutions are changing how farming practices are managed. These technologies improve crop yields, reduce resource wastage, and streamline farm operations.
How AI is Transforming Farming Practices
The integration of agricultural AI advancements has significantly improved farm management. Machine learning algorithms analyze satellite images and weather data for market demand analysis, boosting productivity. AI also aids in risk management by forecasting and predictive analysis, reducing crop failure risk.
AI systems analyze fruits and vegetables, providing insights on quality, ripeness, size, defects, and diseases. These insights lead to quicker and more accurate decision-making, impacting profitability.
Benefits of AI-Powered Solutions
The benefits of AI-powered solutions in agriculture are significant. AI algorithms enhance crop yields and efficiency. For example, AI facilitates seed cross-breeding for better hybrid varieties that resist diseases and adapt to weather changes.
AI's role in soil health monitoring is also critical. It analyzes soil properties, estimates missing nutrients, adjusts fertilizer use, and recommends long-term soil health strategies. AI-powered hardware like sensors and image recognition tools track crop changes, ensuring timely interventions for crop maturity predictions.
Aspect | Traditional Methods | AI-Powered Solutions |
---|---|---|
Weed Control | High Herbicide Usage | Up to 90% Reduction in Herbicide Usage |
Disease Detection | Manual Inspection | High Accuracy with Machine Learning |
Resource Management | Estimates Based on Experience | Data-Driven Decisions and Forecasts |
Crop Yield | Variable | Enhanced and Predictable |
AI systems in agriculture automate processes like intelligent spraying for weed detection and pest control, reducing pesticide usage by up to 90%. These AI benefits contribute to environmental sustainability and increase farm profitability by lowering input costs.
In conclusion, the future of farming relies on AI-driven agriculture solutions. It's essential for us to embrace these technological advancements to lead in the agricultural sector.
Key Technologies in Crop Monitoring
In modern precision farming, technologies like satellite imagery, drones, and IoT sensors are changing how we collect and analyze data. These tools provide detailed insights into crop health and growth, making farming more efficient. By using AI and computer vision, farmers can now make better decisions with remote sensing data annotation.
Satellite Imagery and Drones
Satellite imagery and drones have transformed crop monitoring by providing high-resolution data for detailed observation. Remote sensing data annotation allows for precise monitoring of crop health and environmental conditions. This technology helps identify areas needing attention, such as pest or disease outbreaks, enabling timely interventions.
Drones, with their various sensors, capture aerial images for flexible field survey data annotation. AI-powered drones use polygon annotation to map crop areas accurately. By tracing crop outlines, drones help assess field conditions and support decision-making.
IoT Sensors and Data Collection
IoT sensors are key for real-time agricultural data collection. They monitor soil moisture, nutrient levels, and weather conditions. Combined with field survey data annotation, this data provides actionable insights. This detail optimizes resource management, ensuring efficient farming.
Utilizing agricultural sensors and remote sensing data annotation allows us to track and analyze agricultural parameters in real time. This approach supports precise farming practices and improves crop health and yield predictions.
Working with data annotation experts, like Keymakr, helps manage large datasets efficiently. This ensures accuracy and consistency for effective precision farming solutions. Outsourcing to specialists provides access to advanced technologies and expert knowledge, significantly improving AI's impact in agriculture.
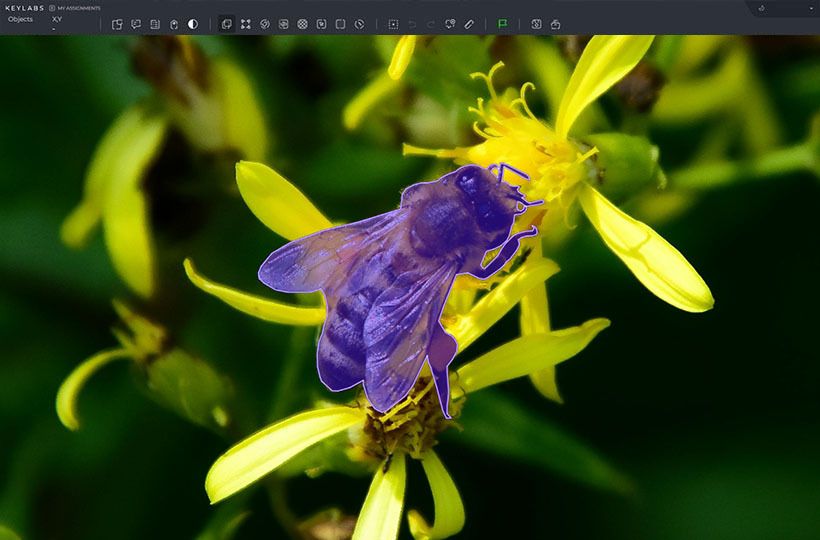
Advantages of AI-Powered Data Annotation
AI-powered data annotation brings significant benefits to precision farming, leading to enhanced farming efficiency enhancement and productivity gains. It is essential for accurate yield prediction, crop health assessment, and resource management.
Enhanced Crop Yield and Efficiency
Yield prediction data annotation allows farmers to forecast crop outputs accurately. This enables efficient resource planning, reducing waste and boosting profitability. By using precise data annotation, farmers can detect diseases early, ensuring timely intervention and better crop health.
Annotated data is vital for automated systems like drones and robots. These systems use AI to monitor crop growth and spot weeds, preventing crop loss. The integration of satellite imagery, drones, and IoT sensors with accurate data annotation further boosts farming efficiency.
Improved Decision-Making Processes
AI decision support in agriculture has revolutionized farming practices by providing detailed insights and analytics. Computer vision systems trained with annotated data offer visual crop condition insights, aiding in informed decision-making. These systems can spot patterns and anomalies beyond human detection.
Timely and accurate data enables farmers to strategize better, aligning operations with environmental conditions and market demands. This alignment leads to better crop management, resource allocation, and enhanced productivity and profitability.
Innovative Startups Making Waves
Innovative agricultural startups are leading the next technological wave in farming. These startups use AI and data annotation to tackle modern challenges like climate change and food security. They create scalable solutions that resonate within the farming community.
Startups excel in introducing novel technologies that combine AI with IoT devices, drones, and satellite imagery for crop monitoring. A notable success is the development of sophisticated pest detection systems. These systems enable farmers to act preemptively, reducing crop losses and supporting sustainable agriculture. Other ventures focus on precision irrigation systems powered by AI, ensuring water usage is optimized without compromising crop quality.
The impact of these startups is clear in their ability to provide tailored, data-driven insights that significantly enhance farming practices. Their agility and innovation distinguish them, continually pushing the boundaries of what's possible in modern agriculture.
Challenges in Crop Monitoring Annotation
In the rapidly evolving field of agricultural AI, several key challenges hinder the effective adoption and implementation of precision farming technologies. Ensuring data quality and annotation accuracy is critical for the reliability of AI models in agriculture. Technological barriers also impede the widespread acceptance of these advanced solutions by farmers.
Data Quality and Accuracy Issues
Precision farming heavily relies on AI models trained with accurately annotated data. Achieving high data annotation accuracy is a significant challenge. The variability in data types, from satellite imagery to drone-captured photos, demands specialized knowledge for precise annotation. Inaccurate annotations can lead to unreliable AI predictions, impacting crop health monitoring and yield optimization.
For instance, growth monitoring AI models require accurate annotations to detect changes in plant size and color. Ripeness detection systems depend on precisely annotated color variations, enabling farmers to harvest crops at their peak. Disease detection models need to identify minute details for early intervention. Platforms like Keymakr's Keylabs provide the expertise to curate high-quality training images, ensuring the data's reliability and effectiveness.
Technological Barriers in Adoption
While AI-powered solutions hold immense promise, technology adoption in farming faces several hurdles. High costs of advanced equipment and insufficient infrastructure make it challenging for many farmers to adopt these technologies. A noticeable digital literacy gap among the agricultural community further slows down the proliferation of such innovations.
Satellite imagery generates vast amounts of data daily, demanding significant computational resources for processing and annotation. Large satellite images, often exceeding 30MB, pose substantial memory and processing challenges, causing performance bottlenecks in many annotation tools. Geospatial data annotation with GeoTIFF images requires handling embedded metadata accurately, essential for applications like environmental monitoring and disaster management.
Innovations in technology are gradually addressing these barriers. For example, multi-spectral imaging in agriculture offers insights into plant health, moisture content, and stress factors, helping farmers make informed decisions. To realize the full benefit of these advancements, overcoming the existing challenges in agricultural AI is essential.
The Future of Precision Farming
The future of precision farming is set to see significant advancements in agricultural technology trends. The integration of AI with robotics and advanced sensor technology promises a transformative impact on farming practices. These developments will optimize resource allocation and enhance crop health assessment and management.
Trends to Watch in Agricultural Technology
Agricultural technology trends are moving towards more sophisticated data analysis tools and IoT connectivity. Modern sensors and drones are becoming essential for gathering real-time data on crop conditions. This enables more precise interventions. The rise in remote sensing capabilities and variable rate technology is leading to more accurate and efficient farming techniques.
- AI Integration: Enhanced predictive capabilities and automated systems are becoming standard.
- Sensor Advancements: Improved sensitivity and accuracy in environmental monitoring.
- Data Analysis Tools: Sophisticated software for deeper insights into crop health and yield optimization.
Predictions for AI and Crop Monitoring
AI predictions in agriculture highlight a future focused on automation and enhanced decision-making support. The integration of AI-driven platforms is expected to revolutionize farm management. This will lead to significant improvements in productivity and sustainability. For instance, automating labor-intensive tasks like weeding and harvesting through AI and robotics will significantly reduce operational costs.
- Increased Automation: AI-enabled machines to perform tasks traditionally done by human labor.
- Enhanced Predictive Modeling: AI systems providing predictive analytics for better crop yield and resource management.
- AI-driven Platforms: Complete farm management solutions that integrate predictive models and real-time data.
The future of precision farming looks promising with ongoing advancements in AI and technology. As the AgTech industry grows, data annotation will remain central to these innovations. Collaborative efforts between data scientists, agricultural experts, and skilled annotators will drive this evolution forward. Offerings like iMerit’s Crop & Weed Detection Data Annotation Technology are key to this progress.
Trend | Description | Impact |
---|---|---|
AI Integration | Automation and predictive analytics | Increased efficiency and productivity |
Advanced Sensors | Real-time environmental monitoring | Better resource management |
Data Analysis Tools | Sophisticated software solutions | Deeper insight and precise farming |
Best Practices for Implementing AI Solutions
The successful AI integration in farming demands a strategic approach tailored to agricultural needs. Understanding farm requirements and using the right AI tools are key. This allows farmers to fully exploit AI's benefits, increasing productivity and sustainability.
Steps to Integrate AI in Agricultural Practices
To effectively integrate AI in farming, follow these critical steps:
- Assess Farm Requirements: Identify the unique challenges and goals of the farm to select appropriate AI solutions.
- Select AI Tools: Choose AI technologies tailored for agriculture, such as satellite imagery, IoT sensors, and data analytics platforms.
- Customize Solutions: Adapt AI tools to address specific farming needs, ensuring a tailored fit for diverse agricultural environments.
- Implement Incrementally: Introduce AI solutions gradually to allow for adjustments and fine-tuning based on real-world results.
- Monitor and Evaluate: Continuously monitor AI performance and assess its impact on farm operations to make necessary improvements.
Training and Resources for Farmers
Effective farmer training in AI is essential to maximize AI's benefits in agriculture. Providing farmers with the right knowledge and skills ensures they can use AI tools effectively. This improves overall farm management. Here are some key resources:
- Workshops and Training Programs: Offer extensive training sessions on AI technologies, covering practical applications and troubleshooting techniques.
- Online Courses: Provide accessible online courses focusing on various aspects of AI implementation and its benefits for farming.
- Consultation Services: Engage AI experts to provide personalized guidance and support in integrating AI into specific agricultural practices.
- Resource Portals: Create online portals with a wealth of agricultural AI resources, including tutorials, case studies, and toolkits.
By utilizing these resources, farmers can overcome AI integration challenges. They can unlock new opportunities for improved crop yield, efficiency, and sustainable agriculture.
FAQ
How is AI-powered data annotation revolutionizing precision farming?
AI-powered data annotation is transforming precision farming by boosting the accuracy and efficiency of machine learning models. This advancement enables the prediction of crop yields and monitoring of crop health. It also optimizes resource use, essential for sustainable food production to meet global demands.
What is crop monitoring annotation?
Crop monitoring annotation involves labeling various agricultural data, including images from drones and satellites, sensor outputs, and field survey data. This process helps AI models understand and interpret farm-related data accurately. It leads to better crop health analysis and management.
Why is accurate data annotation important in farming?
Accurate data annotation is vital as it directly influences decision-making in farming. Properly annotated data ensures high precision in tasks like disease detection and yield prediction. It contributes to improved crop management and productivity.
How is AI transforming farming practices?
AI is transforming farming practices by incorporating advanced predictive analytics and machine learning technologies. This enables farmers to anticipate and address challenges swiftly. It leads to more efficient operations and better farm management.
What benefits do AI-powered solutions bring to agriculture?
AI-powered solutions in agriculture offer numerous benefits, including increased crop yields and reduced resource wastage. They enhance disease and pest control and improve overall farm management efficiency. These advantages lead to more sustainable and profitable farming operations.
How do satellite imagery and drones contribute to crop monitoring?
Satellite imagery and drones provide high-resolution data that, when annotated, aid in detailed monitoring of crop health and growth patterns. They also monitor environmental conditions affecting the farmland. This helps farmers make more informed decisions regarding crop management.
What role do IoT sensors play in agriculture?
IoT sensors collect real-time data from the field, such as soil moisture and nutrient levels. Annotated sensor data helps in precise tracking and analysis of these parameters. It enables farmers to manage resources more effectively and improve crop health.
How does AI-powered data annotation enhance crop yield and efficiency?
AI-powered data annotation enhances crop yield and operational efficiency by enabling accurate predictions and assessments. It relates to crop health, disease risk, and resource requirements. This ensures timely and precise interventions, boosting productivity and efficiency.
How does annotated data improve decision-making processes in farming?
Annotated data improves decision-making by providing accurate and detailed insights into various agricultural factors. It aids farmers in planning and executing farming operations more effectively. This leads to increased productivity and profitability.
Are there successful examples of AI implementation in large agricultural firms?
Yes, large agricultural firms have successfully implemented AI-powered data annotation systems. This has resulted in substantial improvements in crop yields, resource management, and overall farm efficiency. These success stories highlight the transformative power of AI in farming.
How are startups contributing to AI advancements in farming?
Startups are playing a key role in the agricultural sector by introducing innovative AI-driven solutions. They tackle modern farming challenges like climate change and food security. Their fresh approaches and technologies are driving the adoption of AI in agriculture.
What challenges exist in crop monitoring annotation?
Challenges in crop monitoring annotation include ensuring data quality and accuracy, which impacts the reliability of AI models. Variability in data types and the need for specialized knowledge for precise annotation are significant hurdles.
What are the technological barriers to adopting AI in agriculture?
Technological barriers to AI adoption in agriculture include high costs, lack of infrastructure, and digital literacy gaps among farmers. Overcoming these barriers requires investment in technology, training, and support systems. This will facilitate widespread use of AI solutions in farming.
What are the key trends in agricultural technology?
Key trends in agricultural technology include the integration of AI with robotics, advancements in sensor technology, and the development of sophisticated data analysis tools. These trends are driving the evolution of precision farming towards more automated and efficient practices.
What predictions are there for AI and crop monitoring in the future?
Predictions for AI and crop monitoring include increased automation, enhanced predictive capabilities, and greater use of AI-driven platforms for farm management and decision support. These advancements are expected to further revolutionize farming practices.
What are the best practices for implementing AI solutions in agriculture?
Best practices for implementing AI in agriculture involve understanding specific farm requirements, selecting appropriate AI tools, and customizing solutions to meet those needs. Providing training and accessible resources for farmers is essential to facilitate adoption and efficient use of AI technologies.
How important is continuous improvement and innovation in farming?
Continuous improvement and innovation are critical to addressing the evolving challenges of food production. By embracing modern technologies like AI and data annotation, farmers can enhance productivity, conserve resources, and ensure sustainable farming practices.
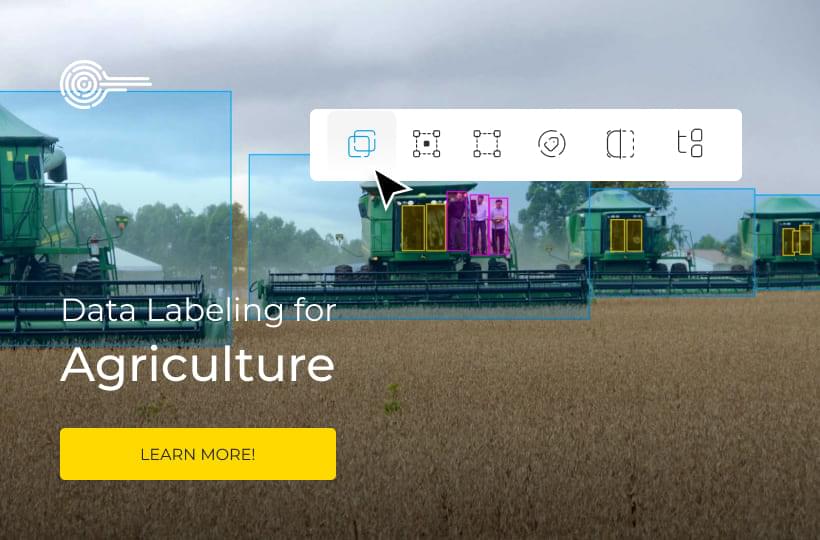