How Object Interpolation Speeds Up Video Annotation
Video annotation is a laborious process. Ten minutes of video footage can contain around 150,000 individual frames, all of which have to be accurately labeled by an annotator. Thousands of hours of pre-labeling and labeling work are required in order to precisely label the amount of video data. Computer vision based AI models need for effective object detection training.
As a result of these pressures there is increasing demand for video and image annotation tool features. They can speed up the labeling of video footage. Companies, like Keylabs, are meeting this need with automated annotation features, one of which is object interpolation.
TThis blog will define object interpolation for video annotation and show the extent to which it can accelerate labeling tools. It will also suggest how this feature can aid computer vision annotation tool development in a number of sectors.
What is Object Interpolation?
Object interpolation uses an algorithm to track objects through multiple frames in video sequence. This means that the annotator is freed from having to locate an object in every frame of a video. Using the Keylabs video annotation tool the process works as follows:
- The annotator localises an object in a starting key frame, for example a car, using a bounding box annotation.
- The annotator then moves the video forward a set amount of frames and places another bounding box around the car in another key frame.
- The algorithm then locates the same car in the interstitial frames. The bounding box tracks the object smoothly across these frames and can be labeled appropriately.
- The annotator checks the labeling to ensure the car is being tracked accurately. If the bounding box is off target at any point additional key frames can be added to steer the algorithm correctly.
Object interpolation capability has the capacity to greatly accelerate the annotation of video footage. Annotators are now only required to locate the object in a small amount of frames, and monitor the output of the active learning for mistakes.
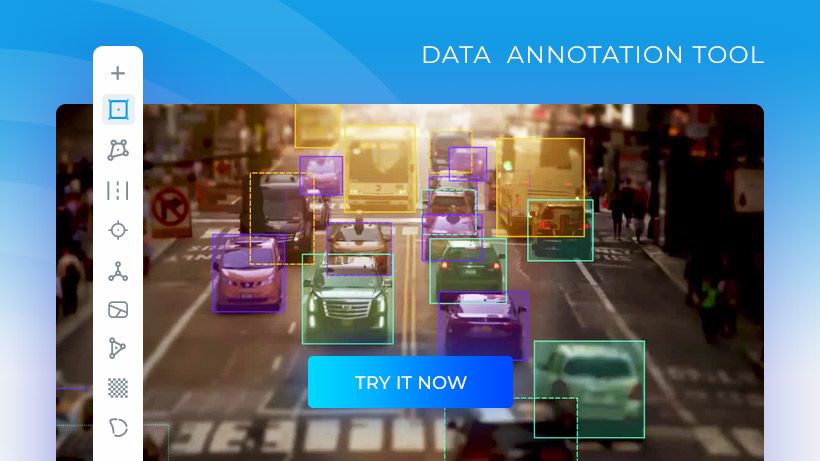
Usages and Use-Cases
Object interpolation features for AI project management tools and annotation tools have applications across a wide range of industries and use-cases:
- Autonomous vehicles: Self-driving vehicle development depends on large quantities of annotated video training data. For AI systems to operate on the road they need to be able identify the movement of different types of vehicles with one hundred percent accuracy.
Object interpolation is particularly vital for producing the data annotation this industry needs. The increased efficiency of this labeling feature is supercharging the production of quality training datasets and data labeling tools. - Retail: The retail sector is beginning to use computer vision AI-enabled cameras to track the movements of customers around their stores. This technology aims to improve customer experience and increase sales. Object interpolation can be employed by project teams working on data for these systems to follow customers from frame to frame in a fraction of the time.
- Aerial: Artificial Intelligence (AI)-empowered drones are being developed with the capacity to monitor traffic and report accidents, leading to clearer and safer roads. Long-distance video footage from drones contains large amounts of vehicles, all of which need to be tracked in each frame.
The timesaving nature of object interpolation will increase the efficiency of aerial traffic video annotation exponentially.
Annotation Tools with Integrated Object Interpolation
Keylabs is an annotation tool designed and built by annotation specialists. This state-of-the-art platform features the object interpolation and automatic labeling capabilities to streamline annotation processes.
