How To Measure the Effectiveness of A Data Annotation Project and Machine Learning Data Labeling Tools
Every successful data annotation team needs to understand the goals of the data annotation project. What is it exactly that they need to achieve? What is the best way to measure that achievement? Datasets are pretty large, so-called "Big data" that can take a long time, even with excellent efficiency. So, how to make sure that time and effort are not wasted?
There are a few things to do to make sure that your data annotation meets or even exceeds the expectations that you set. It also helps to know what is possible and what you can reasonably expect. It is also important to be able to provide relevant feedback. Finally, good management skills enable the success of your data annotation project and your team.

There should always be some human involvement in processing and testing data. That way, performance can be better assessed, the process can be improved, or problems can be corrected. There are some standard metrics that you can use to track performance. However, sometimes these metrics don't fully capture the precision and accuracy of the data annotation. This can still take human insights.
One way you might measure the effectiveness of your data annotation project is to break it up into batches. Then iterate your model and data training as you add each batch of annotated data. The first iterations may not give you the final results you are looking for, but you should be able to see that it is useful for training your model. If it is not effective, that may show you need to use a large enough dataset on each iteration.
The data a model analyzes can change from time to time and on different iterations. That is one reason why keeping track of the relevant metrics is essential. This does not just show where improvements may be made. Such measurements can also reveal data training needs and labeling gaps. This can also help make sure that you have enough diverse data to draw on to make predictions and draw outcomes.
The precision and accuracy of the data labeling are also vital for the efficacy of your data annotation project. Even the best data labeling tools take skill and experience to be effective. It is also important to remember that manual data labeling is time-consuming. Even when a custom AI labeling tool is used to automate the process later in a very large project, it still takes time. That means it is a good idea not to rush the process, and taking less time is not a sign that the data annotation project is effective.
Helpful Metrics and Ways of Measuring the Effectiveness of Labeling Tools
- The number of total labels.
- The number of Data types and categories.
- User reports on individual data annotator performance.
- The ratio of things like bounding boxes that actually denote objects. In a perfect world, this will be 1
- The ratio of objects that are correctly labeled by things like bounding boxes. Again you are looking for a 1 here.
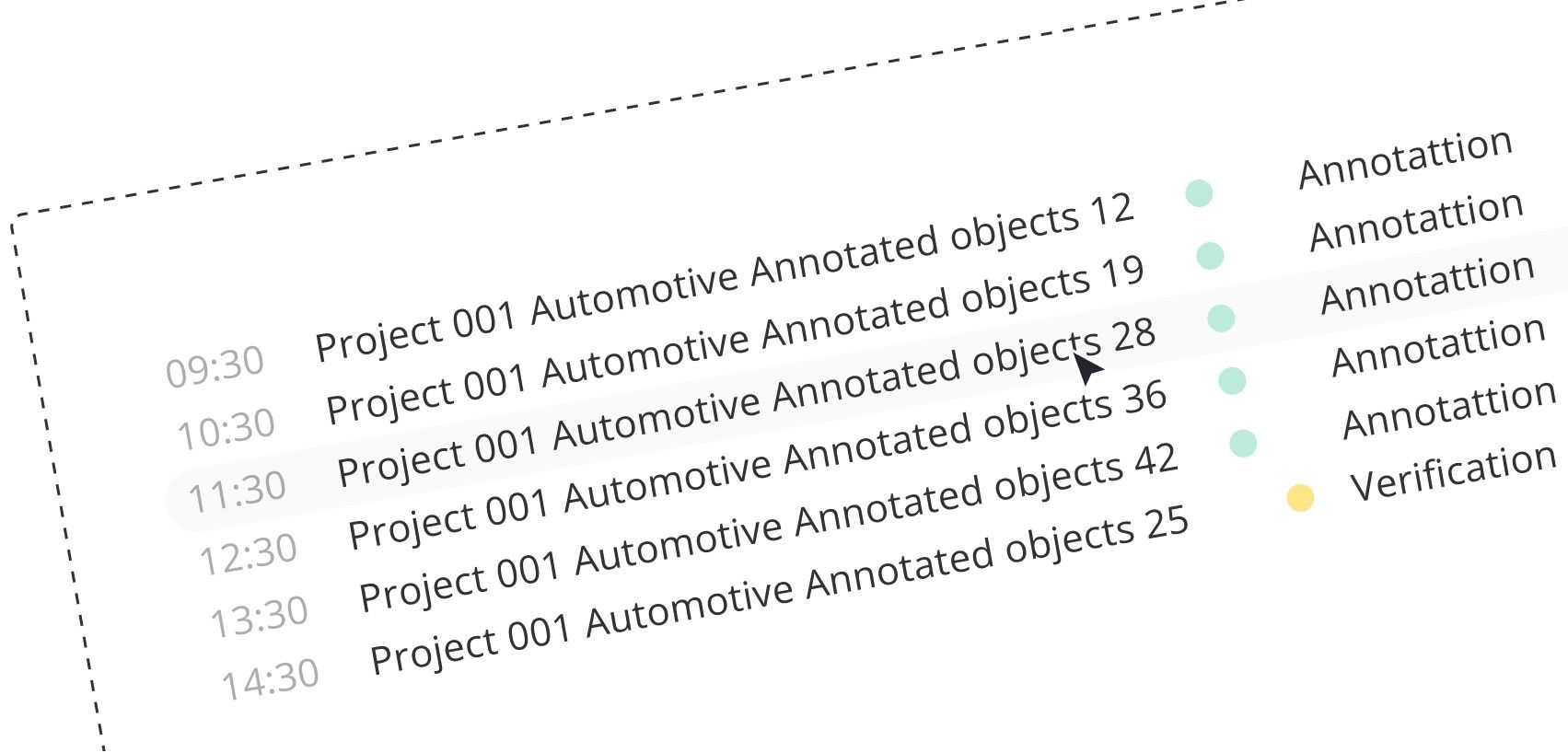
How to Make You Sure You Have the Best Data Labeling Tools
You can always choose to trust us to provide all the data you need and the best data labeling tools and outsource data labeling services. Still, it is important for you to see that for yourself and understand how to measure it. You should know that calculating the true precision using ratios and the real recall of a dataset is hard. That can be as expensive as the data and data annotation. A manual critical review of the entire dataset and labeling is just impractical.
Instead, it is better to pull a random sample that is valid for statistics for review. You can then use probability theory to draw conclusions. That is much more efficient and costs less to do. If you do this correctly, you can calculate a high degree of certainty about your conclusions. The larger the sample and the more review done, the greater the certainty.
You can add a property of consensus. For example, if you have a number of data annotators to label the same image in the same way, then it is probably precise and correct. It may seem redundant, however, to make a difference in knowing you have good precision and recall.
The best labeling tools for machine learning are like other tools, right for the job. For example, bounding boxes are pretty cheap and standard fare, so you should definitely use them. However, bounding boxes may not be the right tool for a job. When you need information on the three dimensions of an object, you actually need cuboids.
Quality assurance, benchmarks and metrics are all well and good. They also will not be so helpful if you are not using the right tool for what you need to do. Some tools provide better or more information than other labeling tools. It is a good idea to compare like to like. For example, if you want the best labeling tool for semantic segmentation, you should compare semantic segmentation tools to find the best one.
Finally, the best labeling tool is one you don't have to use yourself. It is best to leave data annotations to the experts. That way, you can be sure they are using the right tool for the job, and everything is done correctly. You can save a lot of time and money and prevent a lot of problems that way.
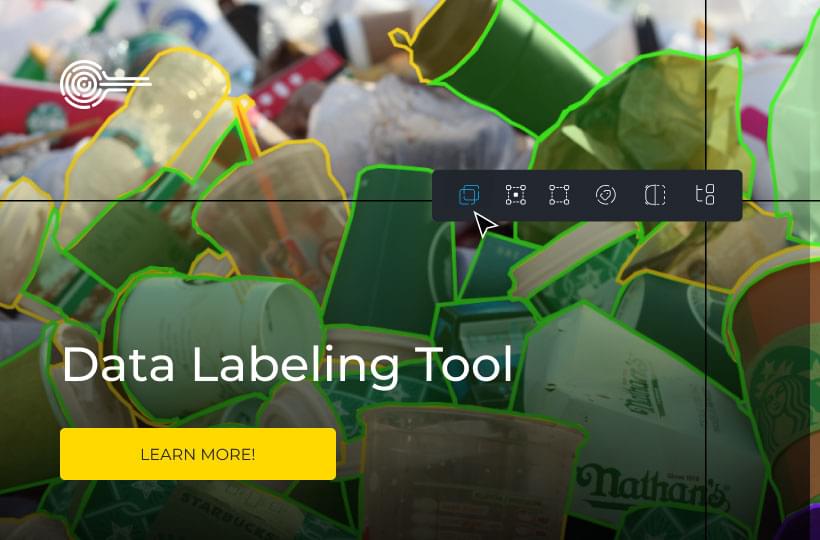