Improving Semantic Segmentation for Waste Management AI with Annotation Tools
Tackling plastic pollution is vital, because waste plastics threaten ecosystems and can be bad for our health. Therefore it is important that we maintain effective recycling and waste management practises. Conscientious waste management takes the pressure off the natural world, and keeps human workers safe from dangerous materials and chemicals.
Computer vision models are being used more and more by the waste management industry to make processing of potentially harmful waste safer and more efficient. However, this technology relies on semantic segmentation annotation.
This annotation method helps to train AI models so that they can recognise waste products and handle them correctly. Data annotation tools can help waste management AI companies achieve precise semantic segmentation in their datasets.
Firstly, this blog will define semantic segmentation. Secondly, we will show how semantic segmentation allows AI models to identify and categorise waste. Thirdly, we will look at the advances being made in the handling of hazardous waste. And finally, we will identify the key advantages offered by data annotation tools, like Keylabs.
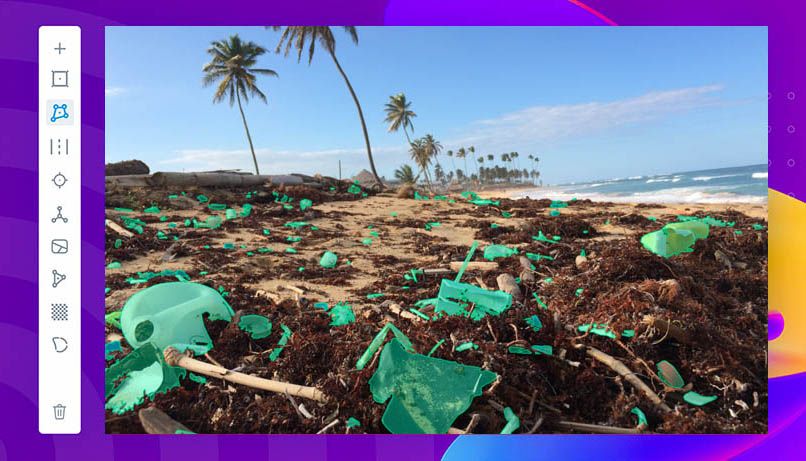
What is semantic segmentation
Semantic segmentation for image and video annotation means dividing images and frames into classes, at the pixel level. To do semantic segmentation human annotators outline target objects, people and animals using an annotation tool. Outlined “things” are then given a colour and a label.
Eventually, every pixel in the image or frame is attached to a labelled class. For waste management models, annotators outline and label relevant objects. For example, specific plastic types are located and separated from the background or other, non-target, objects. This creates a pixel mask that models can be trained to output when presented with new images.
Sorting waste objects
Semantic segmentation supports important applications in the waste management industry. Waste categorization AI systems allow sorting facilities to process waste faster and with less input from human operators. Computer vision models can be combined with robotic arms to autonomously sort waste into defined categories, recycling and landfill for example.
However, accurate semantic segmentation is essential for the technology to function consistently. Training datasets helps computer vision systems efficiently identify different waste types on a fast moving conveyor belt. Accurate training data greatly increases the reliability of the entire waste sorting process.
Handling medical and chemical waste
Sorting facilities need to be able to identify and process potentially dangerous waste. Medical and biohazard waste can be difficult to identify in large collections of other waste. As a result these materials can cause harm to the people we ask to sort and dispose of our waste products.
Therefore, AI is being deployed to protect human workers by quickly finding and removing hazardous waste. Computer vision models can work in combination with industrial robotics. Together these systems can locate dangerous objects, like syringes or biohazard bags, and process them without human workers needing to handle them.
Computer vision models in waste sorting facilities, working with robotic arms, are dramatically improving worker safety whilst ensuring the dangerous waste is still correctly managed.

Annotation tools for semantic segmentation
As has been shown, today’s most exciting waste management AI applications are the product dependent on quality semantic segmentation annotation. This means that in order to be successful AI innovators in this sector need a powerful annotation tool. Keylabs is built to make large dataset annotations more precise and easier to manage:
- Workforce analytics: Keylabs gives managers unique insight into the performance of individual annotators. This means that future annotation tasks can be given to those who are performing well, or who are particularly well suited.
- Video annotation: Annotating video is difficult because even short pieces of training footage contain thousands of individual video frames. Keylabs is designed by video annotation experts.
Multiple annotators can work on the same piece of video at the same time. Their work can then be seamlessly stitched back together, without compromising on quality.
Hot keys: Keylabs is designed to accelerate animation. Efficient hotkeys help to achieve this.