Model-Based Labeling Tools
The role of model-based labeling tools in AI development is changing how data is annotated, reducing time and effort for scalable AI applications. We will explore the landscape of model-based labeling tools. How are these tools changing data preparation and their functions? This will give you an idea of how these tools improve your AI development workflows. And help you choose the right model-based labeling solution for your needs.
Quick Take
- Model-driven labeling tools can reduce annotation time.
- These tools minimize human error, ensuring annotation accuracy.
- Integration enables seamless collaboration and data access.
- Intelligent tooling accelerates annotation for complex tasks across industries.

What are model-driven labeling tools?
AI model-driven labeling tools use pre-trained machine learning models to annotate data automatically. They speed up creating AI training sets and reduce the need for manual annotation.
Benefits of tools in data annotation
- Save time. AI model-driven annotation reduces the time spent manually labeling data.
- Improved accuracy. Pre-trained ML models provide accurate data annotation.
- Reduced costs. Automating data annotation minimizes the need for manual labor.
- Rapid iteration. ML teams train and test AI models, which speeds up the development process.
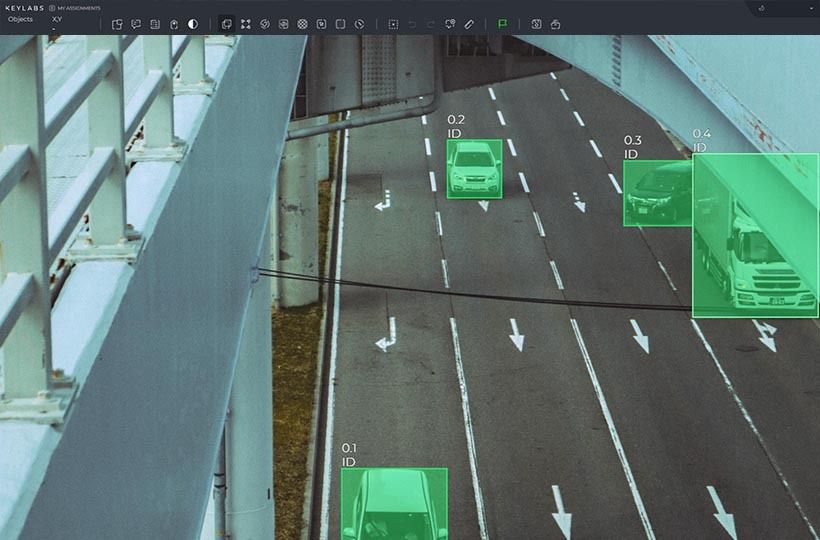
How AI Model Labeling Tools Work
- Pre-training an AI model that has basic knowledge of the data structure. This allows it to work with text, images, video, or audio.
- Automatic annotation allows you to analyze input data and predict data labels. Two approaches are used for this: pseudo-labeling or clustering.
- Validation of annotations by a human on automatically annotated data. This increases the accuracy of labeling and reduces noise in the data.
- Feedback and retraining increase the accuracy of subsequent iterations and adapt to the tasks of AI models.
- User interaction and experience allow annotators to check and adjust the AI-generated labels.
The Keylabs platform has a user-friendly interface and ML-enabled tools that simplify the data labeling process and ensure high accuracy.
Comparing Performance Metrics
This section provides a detailed tool comparison, evaluating key performance metrics such as accuracy, throughput, and automation rate.
Challenges and Limitations
Large data sets require computing power that not all organizations have. This leads to performance issues, slows the annotation process, and reduces project time.
Data privacy is a concern for model-driven labeling. Compliance with regulations, such as GDPR and HIPAA, is key. Tools must have safeguards to protect data privacy. This impacts trust, especially in healthcare, where data privacy is exceptionally high.
User resistance hinders the adoption of new labeling tools. Poor training and changes in workflows make data annotation difficult. Some employees may fear change, leading to reluctance to implement new technologies. To overcome this problem, a clear explanation of why the change is needed and a comprehensive training program are required.
By avoiding these problems early, enterprises can unlock the full potential of AI model-driven labeling tools.
Future Trends in Model-Assisted Labeling
AI-driven automation is transforming the model-based labeling process. It reduces manual annotation and speeds up the work of processing complex datasets. The development of automation and integration of AI has increased speed and accuracy. Therefore, AI-based annotation tools are essential for managing large-scale labeling tasks.
The tools now include intelligent predictions and real-time quality checks by integrating predictive analytics into AI-based modeling. This increases accuracy and minimizes manual annotation. This is required in medicine, and autonomous vehicles, where accurate annotation of medical images and road signs is essential for patient safety and care.
The complexity of AI projects requires collaboration features in AI-based labeling tools. Cloud-based annotation tools allow teams to process large datasets with high quality.
FAQ
What are model-driven labeling tools?
AI model-driven labeling tools use machine learning or artificial intelligence (AI) algorithms to automatically or semi-automatically annotate data.
How do AI model-driven labeling tools improve the data annotation process?
AI model-driven labeling tools automate or semi-automate the annotation process, reducing manual labor. They improve the accuracy of data annotation through iterative learning and feedback-based error correction.
What are the main features to look for in model-driven labeling tools?
Automation, a user-friendly and intuitive interface, scalability for large data sets, and reliable support.
How do these tools compare in performance?
Performance is measured by speed, accuracy, and user satisfaction.
Are there any challenges or limitations in using model-driven labeling tools?
Challenges include technical hurdles, data privacy concerns, and user resistance.
What does the future hold for AI-powered labeling tools?
Automation, predictive analytics, and collaboration capabilities are expected to expand.
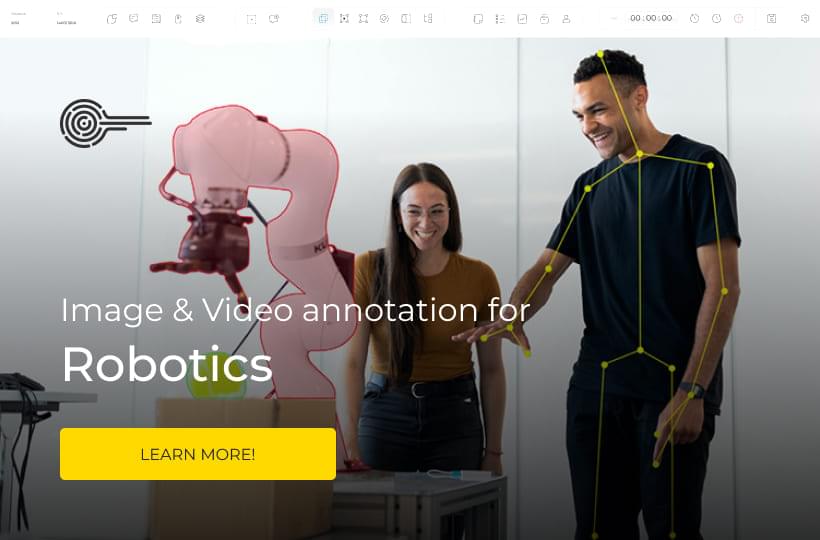