Overcoming Data Labeling Challenges: Expert Solutions
Did you know that data labeling mistakes can greatly reduce how well machine learning models work? Study shows that errors in data labeling might lower model performance by as much as 30%. This affects many uses, from self-driving cars to processing human language.
With technology growing fast, the need for well-labeled data has never been more important. Organizations now face big data labeling issues. These include making sure data is correct, dealing with how to mark data, and taking care of privacy worries. Yet, there are expert ways to solve these issues and improve machine learning results.
This article will look at the various sides of data labeling. We'll talk about the challenges that come up and offer practical solutions. We aim to cover the top ways to label data well, look at important tools and tech, and suggest ways to get better accuracy. We will also touch on common problems and give advice on picking data labeling partners.
Key Takeaways:
- Poor data labeling accuracy can lead to a significant reduction in machine learning model performance.
- Data labeling challenges include ensuring data accuracy, handling annotation difficulties, and addressing privacy concerns.
- Expert solutions, including best practices, tools and technologies, and human-in-the-loop approaches, can help overcome these challenges.
- Evaluating and choosing data labeling partners require careful consideration of expertise, data privacy measures, and quality assurance processes.
- Measuring the impact of data labeling solutions is essential for understanding their effectiveness and optimizing future labeling efforts.

Understanding Data Labeling Challenges
Data labeling is crucial for teaching machine learning models. We tag data with labels to make models accurate and reliable. But, this task has its hurdles. We’ll look at the main problems faced in data labeling.
Data Annotation Difficulties
Labeling data is complex and takes a lot of time. Experts must identify and label various data correctly. If annotations aren’t accurate or consistent, it hurts model performance. Challenges include understanding unclear data, managing different data types, and interpreting complex patterns accurately.
"The process of data annotation can be challenging due to the inherent subjectivity and variability in data interpretation. It requires annotators to possess domain knowledge and a deep understanding of the labeling guidelines to ensure accurate annotations."
Labeled Data Obstacles
Gathering a big, quality dataset for labeling is tough. It’s even harder for specific fields or tasks. The lack of labeled data slows down making accurate models. Also, there’s the issue of data bias. This means the data might not truly show the diversity of the target group, leading to uneven results.
"The scarcity of labeled data and the potential bias in the labeling process can introduce challenges in achieving reliable and unbiased machine learning models. It requires careful planning and evaluation to ensure that labeled data is representative of the desired target population."
It’s key to understand and tackle these problems for good data labeling. Knowing the issues with data annotation and labeled data helps. This lets organizations use the right strategies and tools to beat these challenges. Next, we’ll talk about how to get precise and dependable data labeling. This will help organizations build top-notch machine learning models.
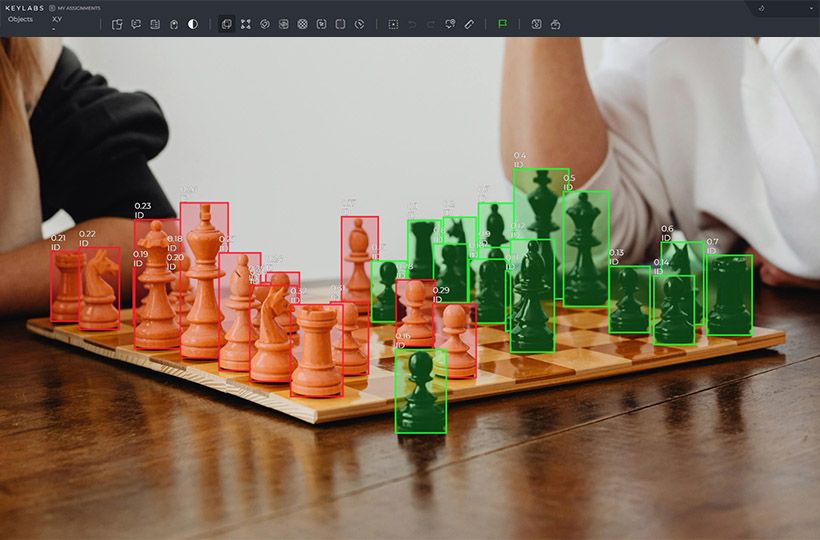
Best Practices for Effective Data Labeling
For good machine learning training data, following best practices is key. This helps data labelers make accurate and reliable models. Here are some main best practices:
1. Define Clear Labeling Guidelines
Clear and comprehensive labeling guidelines are crucial. They guide data labelers to be consistent. It's vital to explain how to label different data types, including tough cases.
2. Conduct Regular Labeling Quality Checks
It's key to check the data's quality regularly. Use quality checks to spot and fix any errors. This boosts training data quality.
3. Leverage Human-in-the-loop Labeling
This method uses both human brains and automated tools. Humans can correct or refine automation's work. It tackles hard cases better, making data more reliable.
4. Use Consensus Labeling for Ambiguous Data
Difficult data needs a special approach. Multiple people review tough cases and agree on the labels. This makes the labels more trustworthy.
5. Continuously Update and Train Labelers
As methods improve, labelers need to stay current. Giving regular training helps them keep up with new ways. This lets them adjust to new guidelines.
Best Practices for Effective Data Labeling |
---|
Define Clear Labeling Guidelines |
Conduct Regular Labeling Quality Checks |
Leverage Human-in-the-loop Labeling |
Use Consensus Labeling for Ambiguous Data |
Continuously Update and Train Labelers |
By using these practices, data labelers help build better machine learning models. Constantly improving data labeling steps up machine learning systems' performance.
Data Labeling Tools and Technologies
Choosing the right tools and technologies changes how we handle data labeling. Automation and machine learning have transformed it from a manual chore to a more streamlined process. Now, organizations have various tools to make their work faster and more accurate.
Automating repetitive tasks is a major plus of data labeling tools. This kind of automation speeds up annotation, making it less of a burden on people. It takes over simple tasks, like sorting images or analyzing feelings. This frees up human labelers to tackle more complex issues that need a personal touch.
Many data labeling tools are designed to be easy to use. They have helpful features like preset annotations, shortcuts, and clear guidelines. This helps ensure that every piece of data is labeled correctly and consistently. Plus, these tools let many people work on the same project at once, without getting in each other’s way.
These tools also come with features to keep the quality of work high. They use quality control checks, revisions, and validations. This means the data used for training machine learning models is as good as it can be.
Some tools even use machine learning to get better results. They might suggest labels or focus on specific areas that need work. This helps the tools get smarter over time, making the labeling even more precise.
When picking out data labeling tools, it’s important to think about the project's needs. Consider the task's complexity, how much data there is, and how much of the process should be automatic. The right tools are easy to use and can grow with your project.
Essentially, data labeling tools are key to better data handling. They automate the simple stuff, are user-friendly, maintain quality, and use machine learning to improve. This lets organizations label data more effectively and accurately.
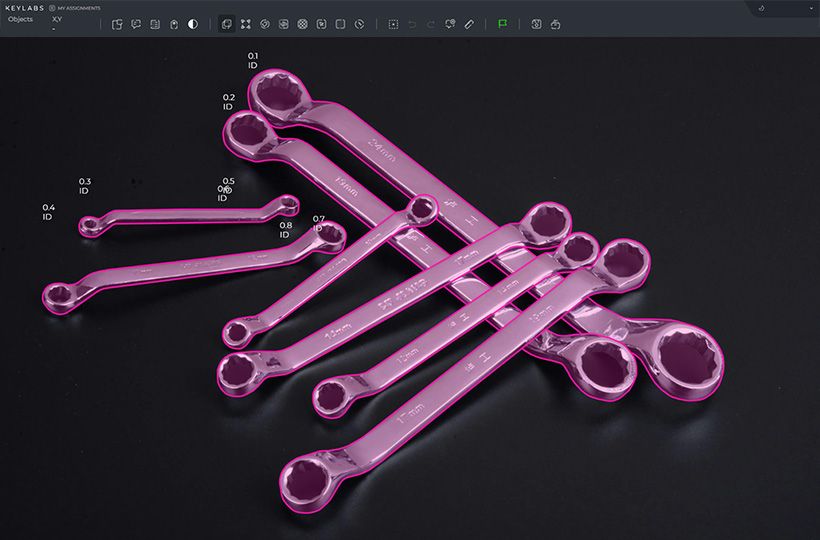
Improving Data Labeling Accuracy
Data labeling needs to be accurate. The success of machine learning models relies on good data. So, improving labeling accuracy is key for great results.
The human-in-the-loop data labeling method is very effective. It mixes human knowledge with automated methods. This way, we get the best of both worlds for high accuracy.
Human annotators bring their understanding and skills to the table. They are great at spotting patterns and making accurate marks. Sometimes, computers can't catch these details on their own.
Automated techniques, on the other hand, make data labeling faster. They're good for easy tasks. Human annotators check the work, ensuring it's all correct.
This mix improves accuracy a lot. With human-in-the-loop, even big datasets can be labeled well. This doesn't sacrifice the quality.
To do this right, humans and machines must work in harmony. This requires clear rules and ongoing training. Quality checks are also vital to keep everything on track.
Using human-and-machine teamwork boosts data labeling quality. This leads to better machine learning models. It's a smart move for accurate results.
Benefits of Human-in-the-Loop Data Labeling:
- Improved accuracy through human expertise
- Efficient handling of complex scenarios
- Scalability for large datasets
- Reduced manual effort through automation
- Consistent and reliable labeling
This example shows how it works:
A self-driving car company needs to spot traffic signs accurately. They use human-in-the-loop labeling. Humans check what the computer does first. This back-and-forth makes the labeling precise. So, the car reads signs well, making it safer on the roads.
By using this method, companies can trust their data more. This improves their tech projects a lot.
Addressing Common Data Labeling Challenges
Data labeling is key for making machine learning models that are reliable. But it comes with its challenges. Here, we'll look at these challenges and suggest ways to beat them.
Annotator Bias
Annotator bias is when personal views affect the data labeling. Providing clear instructions can help prevent this. This ensures annotators know exactly what to do.
Label Ambiguity
Label ambiguity happens when labels are unclear. This can make annotations inconsistent. A good way to avoid this is by chatting regularly with annotators. This clears up any confusion and keeps labeling consistent.
Scalability
Handling more data can be hard. Labeling lots of data by hand is slow. Using tools that label data automatically or with some human help can make this faster.
“Data labeling tools powered by AI and machine learning algorithms have the potential to revolutionize the data labeling process, providing efficient and accurate solutions.”
Data Quality Control
High-quality data is a must for good machine learning models. The trick is fixing errors in the data. Doing regular checks and having experienced annotators review the data helps keep it accurate.
Dataset Bias
Dataset bias means some data is overrepresented. This can make models less effective in the real world. To beat this, design training data to include a wide range of perspectives.
Common Data Labeling Challenges | Solutions |
---|---|
Annotator Bias | Provide clear guidelines and instructions to annotators |
Label Ambiguity | Establish a feedback loop and regular communication with annotators |
Scalability | Employ automated or semi-automated data labeling tools |
Data Quality Control | Implement regular audits and double-checking by experienced annotators |
Dataset Bias | Carefully design the training data to avoid bias and ensure representation |
The Role of Data Labelers in Data Labeling Process
Data labelers are key in creating accurate labeled data. They ensure machine learning models succeed with their attention to detail. Without them, we face major setbacks in developing effective solutions.
Data labeling challenges can pop up anytime. These range from choosing the right annotation methods to handling complex datasets. Experts in data labeling overcome these issues by precisely labeling data, following strict guidelines.
Ensuring data quality is a major task for data labelers. They review every piece of data carefully. This meticulous work helps train machine learning models better.
The Expertise of Data Labelers
Data labelers have knowledge in various fields. This allows them to label data correctly based on its context. Their understanding improves the dataset's relevance and usefulness.
"Data labelers act as the bridge between raw data and machine learning models. Their skill is crucial in turning unstructured data into useful datasets for accurate predictions."
Data labelers also enhance the labeling process with their feedback. Their input helps refine labeling methods and address new challenges efficiently. This teamwork leads to top-notch datasets.
Collaboration and Communication
Data labelers work with data scientists and managers to meet project needs. Clear communication and guidelines are essential for consistency and error reduction in labeled data.
This teamwork helps tackle complex tasks and clear up any confusion. This ensures the labeled data meets all requirements for model training success.
The Significance of Data Labelers
Data labelers' work in the labeling process is essential. They play a huge role in overcoming challenges and providing precise data. Their skills and adaptability are key to the success of machine learning models.
Ensuring Data Privacy and Security in Data Labeling
Data privacy and security are very important in data labeling. It's crucial to keep sensitive data safe while labeling. This helps maintain the system's trust. We'll look at the best ways and tools that make data privacy and security possible.
Best Practices for Data Privacy and Security
- Implement Access Controls: It's important to have strong access controls on data labeling platforms. This makes sure only allowed people can see and work with confidential data.
- Secure Data Transmission: Using encryption protocols is key when sending data to labelers or partners. Encryption keeps the data safe during transfer.
- Use Anonymization Techniques: Techniques like data masking protect people's privacy. They remove or change personal info before data is shared for labeling.
- Regularly Update Security Measures: Keeping up with new security steps is crucial. Updating and improving security can stop risks and keep threats away.
Data Security Technologies
There are many technologies to improve data security during labeling. Some of these are:
- Data Encryption: Encrypting data, whether stored or being sent, adds protection. It makes data unreadable if someone unauthorized gets to it.
- Tokenization: This replaces sensitive data with random symbols. It helps stop unauthorized viewing and lowers risk.
- Secure Storage: Keeping data in secure places like encrypted databases helps prevent unauthorized access.
"Data privacy and security are vital in data labeling. They ensure personal info is protected and trust is maintained in the system."
By following good practices and using the right technologies, companies can avoid data breaches. This protects the privacy and secrecy of labeled data.
Image:
Data Privacy and Security Best Practices | Data Security Technologies |
---|---|
Implement Access Controls | Data Encryption |
Secure Data Transmission | Tokenization |
Use Anonymization Techniques | Secure Storage |
Regularly Update Security Measures |
Evaluating and Choosing Data Labeling Partners
Finding the right data labeling partner is key to the success of your projects. It's essential to choose a reliable and experienced provider for high-quality and accurate data labels. Consider these important factors to make a good choice:
1. Expertise and Experience
Choose a partner with knowledge in your specific field. A provider that knows your industry can better understand your needs and offer accurate annotations. Asking for case studies or references helps judge their skill in similar projects.
2. Data Labeling Quality Control
Check the provider's quality control steps. A good partner ensures the accuracy and consistency of labeled data with strong quality measures. Look for quality-related certifications to confirm their commitment to excellence.
3. Scalability and Flexibility
Make sure the data labeling service can grow with your project. They should handle more data or new types as needed. The partner must adapt to your changing needs efficiently.
4. Security and Confidentiality
Your data's privacy and security are crucial. Ensure the provider has strict security measures to protect your data. Compliance with regulations like GDPR or ISO 27001 shows they treat data protection seriously.
5. Technology and Infrastructure
Examine the partner's tech and resources. Advanced tools and technology can make labeling faster and more accurate. Ask about their platforms and automation tools to understand their tech expertise.
6. Pricing and Cost-effectiveness
Pricing is important, but not the only factor. Seek a balance between cost and service quality. Compare cost estimates to the value each partner offers.
7. Communication and Collaboration
Good communication is essential for success. Check if the provider responds quickly and has a dedicated support team. A partner should work closely with you and value your feedback.
8. Customer Reviews and Reputation
Research the partner's reputation and customer feedback. Look at testimonials or reach out to their clients for insights. A well-regarded partner will have positive feedback and a strong industry presence.
Evaluating carefully helps you pick the right partner, overcoming challenges and securing reliable data for your projects.
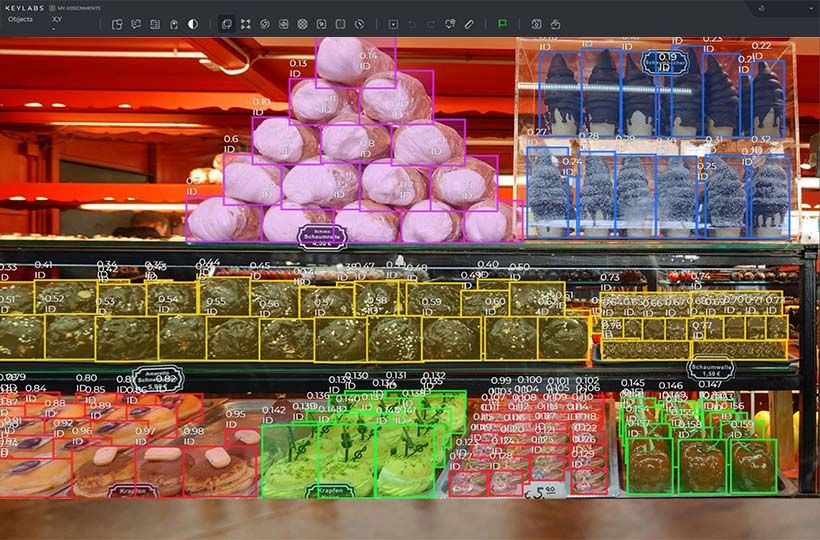
Measuring the Impact of Data Labeling Solutions
After putting data labeling solutions in place, we need to check if they work well. We should look at how they affect the performance of machine learning models. This means using the right methods and metrics to see how valuable data labeling has been.
One method for measuring the impact of data labeling solutions is through accuracy assessment. We can look at how machine learning models did before and after getting labeled data. By looking at how much better they perform, we can see how much data labeling helped.
Another important metric to consider is the efficiency of data labeling. Figure out how much time and resources we saved by using data labeling solutions instead of doing it by hand. If we saved a lot, it means the solution worked well and saved money too.
"Measuring the impact of data labeling solutions is essential in evaluating the return on investment for businesses," states IBM, a top source for data labeling tools. "It lets businesses know if their data labeling strategies work well and where they can get better."
Evaluating the quality of labeled data is another crucial aspect of measuring the impact of data labeling solutions. We need to check the labeled data carefully and compare it with the true or expert judgments. Making sure the labeled data is accurate and consistent is key for trustworthy machine learning models.
Feedback loops and iterative improvements are valuable measures in assessing the impact of data labeling solutions. We should listen to feedback from those training models, data scientists, and users. They can point out what's not working well or where we can make the labeled data even better. This way, we can keep making the data labeling process better over time.
It's very important to check how well our data labeling efforts are doing. This helps us understand their value and how they make our machine learning models better. It pushes us to make decisions based on data, improve our models, and enhance the accuracy and efficiency of our AI systems.
Conclusion
The journey through data labeling shows how crucial good data is for better machine learning models. Even though there are hurdles, experts have figured out ways to get past them and boost efficiency.
One main solution is using smart data labeling tools, as mentioned before. These tools automate work, making things faster and cutting down on mistakes. Still, picking tools that fit your project and protect data is key.
Skilled data labelers are vital for top-quality data. Their work, along with human-in-the-loop labeling, makes data more accurate and trustworthy.
Choosing the right data labeling partners is important for companies. The right partners understand the challenges and provide customized solutions. This leads to successful projects and goals being met.
Data labeling challenges are part of any machine learning project. But, the right approach can solve them. By tackling common problems, using best practices, and taking advantage of new tools, companies can label data well. This improves machine learning model performance.
FAQ
What are the common challenges faced in data labeling?
Data labeling involves challenges like annotation difficulties and label-related obstacles. These can affect machine learning models' accuracy and quality.
What are some best practices for effective data labeling?
For effective data labeling, use top-quality training data. Ensuring robust annotation guidelines is key. Clear communication and continuous improvement are also important.
What tools and technologies are available for data labeling?
There are many tools and technologies for data labeling. They include manual and automated options. These tools make the labeling process more efficient and accurate.
How can data labeling accuracy be improved?
Improving data labeling accuracy involves human-in-the-loop and automated techniques. Quality control and feedback loops also help increase accuracy.
How can common data labeling challenges be addressed?
To address data labeling challenges, improve annotation guidelines. Provide clear instructions and regular quality checks to avoid errors.
What is the role of data labelers in the data labeling process?
Data labelers are key in the data labeling process. Their expertise ensures data is accurately annotated. This is crucial for reliable machine learning data.
How can data privacy and security be ensured in the data labeling process?
Ensuring data privacy and security means using strong protection measures. This includes anonymization, secure storage, and strict access control. Following legal standards is also critical.
How should one evaluate and choose data labeling partners?
When choosing data labeling partners, consider their expertise and experience. Look at their scalability and quality processes. Evaluating privacy practices is key for successful partnerships.
How can the impact of data labeling solutions be measured?
The impact of data labeling solutions is seen in improved model accuracy and process efficiency. Savings in time and positive feedback also signal success.
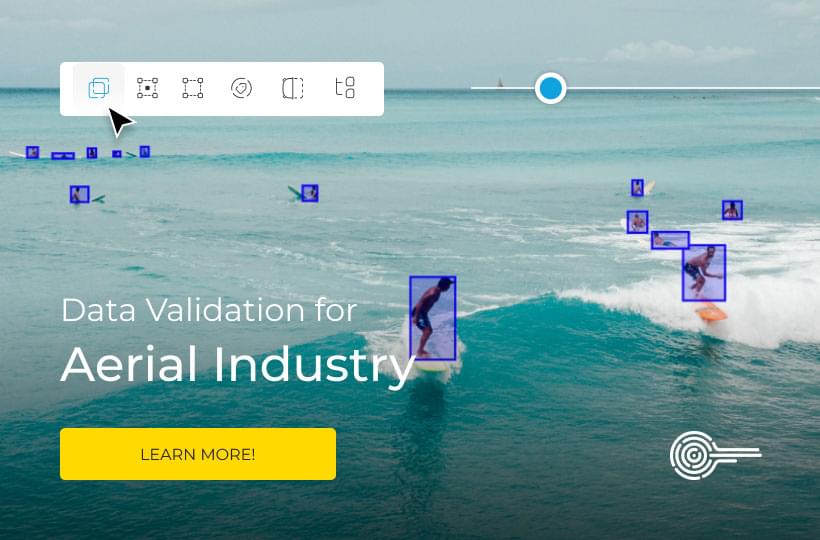