Precision of Data Labeling Tool Annotation: How to Choose the Right Level
Precision is critical to the performance of your machine-learning algorithms. That precision and performance matter most in the development of your AI model. You should not compromise at all on precision. Doing so sacrifices the quality of your final product, and your customers will notice that.
You can always leave all of your data labeling, data creation, and data collection to our team of experts for the highest level of precision. With that said, it would still benefit you to understand why having the highest level precision is so important. Also, you should still know how to choose the best data labeling tools and services to suit your needs.
Precision really does matter a lot in your data and data annotation. Sometimes being a little less precise can still actually be acceptable, though. For example, if you draw cuboids around objects to label things like cars, trucks, buses, and people, going a bit larger can be okay.
That is because AI for autonomous vehicles and drones uses these for things like object detection and collision avoidance. So long as the cuboid is a bit larger than the object, it is more likely to avoid collision with that object successfully. The object will also appear to take up a larger space in the AI's computer vision.
Think of cuboids a little bit like the "hit boxes” used in video games, only you want to avoid all the hit boxes this time. Typically, in developing video games, you want very accurate hit boxes so players can hit them, and it doesn't feel like the game is unfair.
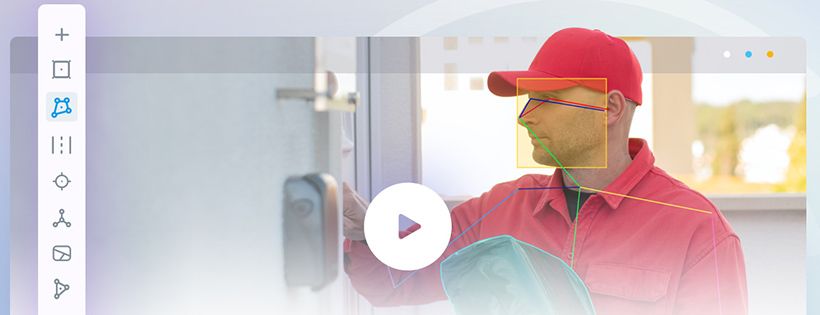
Still, even in this example, more precise cuboids are better. That is because AI accidental collisions are more likely if they are too small. On the other hand, if they are too large, that can throw off other necessary calculations like calculating the distance between objects.
Also, if you happen to be developing a video game, you would want the bounding boxes or cuboids used for hitboxes to be as accurate as possible. That way, the game is fair and more fun. This precision also matters when making an augmented reality or VR game. Otherwise, players blinded to the real world around them are likely to stumble and knock into something.
If you are developing a self-driving car instead, you would expect the cuboids and bounding boxes to be at least as accurate as those used in video games. It just would not seem right otherwise, would it? That is because the self-driving car has much higher stakes than any game.
In fact, image annotation tools for computer vision always need the highest level of precision. That is true, no matter your AI application of that computer vision. Therefore, the best image annotation tool is also always the most precise.
These kinds of accuracy and precision matter and affect the accuracy and precision of your machine-learning algorithms. That is all crucial to the quality of your final AI product. It is also vital in improving the quality of your dataset. So you want to turn up the volume of your signal. You want to have a lot of high-quality, valuable data.
Improving the quality of your data creates a type of virtuous cycle in your machine learning algorithms. That's because it also reduces the noise and the inaccurate or useless data that can cause expensive problems. Those problems can also spiral and trap your project in development for a long time.
Key Takeaways
- You need the highest level of precision possible.
- Precision in your dataset and labeling affects the precision of your AI.
- Precision data labeling tools improve the quality of your dataset.
- Improving the quality of your dataset creates a virtuous development cycle.
- Sacrificing Accuracy and Precision can be a very expensive mistake.
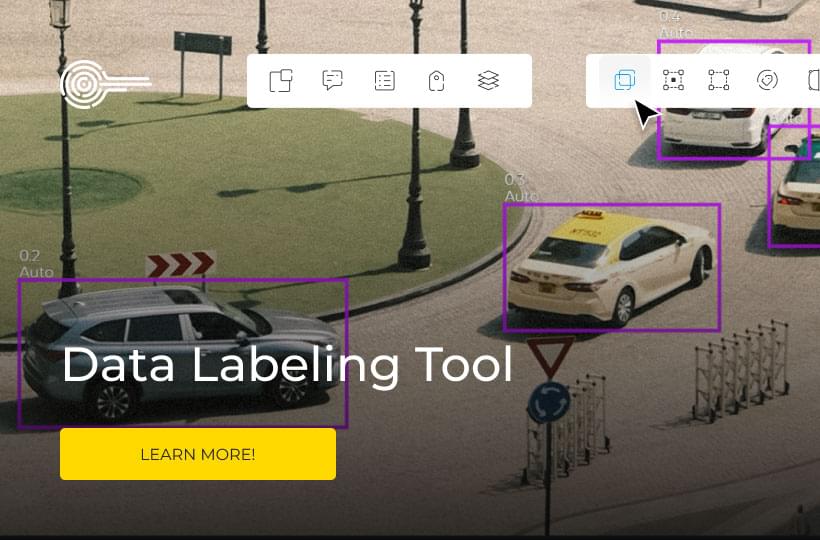
Creating Virtuous Cycles in the Pursuit of Precision
These kinds of accuracy and precision matter and affect the accuracy and precision of your machine-learning algorithms. That is all crucial to the quality of your final AI product. It is also essential in improving the quality of your dataset. So you want to turn up the volume of your signal. You want to have a lot of high-quality, valuable data.
Improving the quality of your data creates a type of virtuous cycle in your machine learning algorithms. That's because it also reduces the noise and the inaccurate or useless data that can cause expensive problems. Those problems can also spiral and trap your project in development for a long time.
A semantic segmentation labeling tool can increase precision a lot. That is because it is used to label every single pixel and classify it. It adds important context as well. This metadata adds more precise data to your dataset. That also increases the signal and decreases the noise. Using the right tool and the best labeling tool increases the precision possible for your AI to achieve.
There are many data labeling tools to choose from, depending on the tasks your AI needs to be trained on. You select these tools based on what you need your AI to learn. What are the features that you need it to have? What is it supposed to do? What do you need to achieve some breakthrough? What gives your AI a competitive advantage over any other?
It is questions like these That also determine the data that you add to your dataset. These questions also help decide the level of precision you need. Although, in reality, you will always want the highest level of accuracy possible. The pursuit of precision is the pursuit of perfection, productivity, and value.