Real-Time Object Recognition: Transforming Industries
Every second, advanced object recognition software analyzes loads of images and videos in many areas. We often overlook how big these operations are. These applications are not just new tech terms. They have pushed many fields to new heights of efficiency and safety.
Self-driving cars, for example, rely on this technology to see and make smart, safe choices. In healthcare, it helps spot early signs of diseases like Parkinson's. Retail stores use it to improve shopping by understanding customer behavior.
This technology is essential for public safety, with smart cameras now a must-have. They are used in everything from facial recognition for security to monitoring farms. Real-time object recognition is not just a new trend. It's changing everything about how we do things.
Key Takeaways
- Real-time object recognition is key for safe self-driving cars by helping them understand their surroundings.
- In healthcare, AI-powered recognition helps watch over patients and spot diseases early, potentially saving lives.
- Retail uses it to watch how shoppers behave and improve where products are placed, boosting sales.
- Security has gotten stronger with it, making public places safer with careful watching.
- It's making farming smarter, leading to more food and less waste.
- Deep learning and neural networks are the core of these smart recognition systems, doing complex tasks in many fields.

The Emergence of Real-Time Object Recognition Technology
The world of computer vision applications is growing fast. It might reach a market value of USD 48.6 billion by 2022. This jump shows how quickly industries are embracing ai-powered object recognition systems.
object detection algorithms
In busy city streets and factory settings, different areas are seeing the benefit of real-time image processing solutions. Companies like IBM play a big role. They create advanced software that makes it easier to use these technologies and uses less computing power.
- Industries like automotive and energy use object detection algorithms to work smarter.
- In traffic monitoring, algorithms track moving objects to improve surveillance.
- Deep learning with CNN and YOLO boosts real-time object detection systems to nearly 97% accuracy.
A vast array of computer vision applications is changing technology today. They need huge databases and affect everything from production lines to health care. Free databases like MS COCO, PASCAL VOC, and Kitti help improve object detection algorithms.
Now, studies focus on real-time systems using the SSD algorithm. They can tell the difference between things that move and those that don't. At its core, this research is about tracking and recognizing moving objects. It's a key part of future ai-powered object recognition systems.
Breaking Down Real-Time Visual Object Detection
Exploring the world of real-time visual object detection reveals the complex relationship between ai-powered object recognition systems and their real-world uses. This area is huge, impacting many computer vision applications that change how we engage with technology.
Understanding the Basics
The basics of real-time visual object detection revolve around special object detection algorithms. These algorithms are designed to quickly identify and find objects in images. Thanks to advanced object recognition software, these systems can process images like humans do, but much faster.
Advancements in Detection Algorithms
Recent years have seen big leaps in object detection algorithms. Techniques like YOLOv7 have improved speed and accuracy, showing the power of ai-powered object recognition systems. This progress shows how far we've come in making tech that can think and analyze like humans.
Application in Video Surveillance
In video surveillance, real-time object tracking technology shows its true value. With it, security systems can watch non-stop, offering strong safety measures. Real-time visual object detection acts quickly in emergencies, showing AI's vital role in our safety every day.
Revolutionizing Manufacturing with AI-Powered Object Recognition
Manufacturing is changing big time, thanks to computer vision applications. These tech advancements are pushing the industry towards smart factories. Now, real-time object recognition is key, helping factories watch, monitor, and manage production with great accuracy.
The YOLOv5 model is a prime example of this tech at work. It combines AI and deep learning to make manufacturing smoother. It has improved its precision a lot, from 0.349 to 0.622. This means better quality control, jumping from 0.552 accuracy to 0.865.
Think about what AI deep learning means for making semiconductors. Spotting the tiniest detail can prevent big mistakes. Real-time object recognition checks carrier tapes very closely. This ensures chips are packed safely for transport, showing the power of computer vision applications.
This tech magic isn't just for the factory floor. It helps with health and safety too, spotting dangers early. It's reshaping fields like finance and security every day. For example, factories use computer vision to better quality checks. This cuts losses and boosts production.
Real-time object recognition applications are building tomorrow's efficient, mistake-free factories. It's all about using AI to improve production. Now, factories can grow, run better, and save resources easily.
"The evolution of manufacturing now hinges on the backbone of computer vision technology, where smart shelves in retail and predictive maintenance in renewable energy projects join the ranks of industries reaping the benefits of object recognition and AI."
As tech grows, computer vision in modern industries has lots of uses. It's changing retail, healthcare, cars, and even farming. These changes are shaping how we live, work, and see technology's future.
Using Keylabs is a great way to help further computer vision applications by creating clean and actionable datasets for training.
Advanced Object Recognition Software in Autonomous Vehicles
The global market for AI in vehicles will reach USD 6.6 billion by 2025. This growth is due to ai-powered object recognition systems. These systems are key for the navigation and safety of self-driving cars. The industry grows at a rate of 36.15% each year, thanks to advanced object recognition software.
The Role of Object Recognition in Navigation
Vehicles use two main methods to detect objects: two-stage and single-stage. Two-stage methods, like RCNN and Faster R-CNN, are precise. On the other hand, single-stage systems such as YOLO and SSD are fast. This makes them good for real-time object tracking technology. Hybrid models combine these methods. They offer both accuracy and speed, enhancing navigation for self-driving vehicles.
Ensuring Safety with Real-Time Detection
Real-time visual object detection is crucial for the safety of autonomous vehicles. The urban landscape is full of surprises. So, cars need advanced software that reacts accurately and quickly. The latest AI models are even more accurate than YOLOv5 and YOLOv7. They keep up with the fast pace needed for safe navigation. Tests show these technologies are key to vehicle safety.
Pedestrian safety gets a boost from new technologies like the multiresolution adaptive fusion strategy. This strategy allows vehicles to detect obstacles with 96.1% accuracy. It uses databases like KITTI and operates at 202 frames per second. This impressive ability is vital. It lets cars move safely and autonomously in a constantly changing world.
Real-Time Object Recognition Applications in Healthcare
The use of AI-powered object recognition systems in healthcare is changing how we diagnose diseases. These advanced computer vision applications help in spotting important details in medical images. This leads to new ways to diagnose diseases and monitor patient health.
For medical image processing, technologies like Faster R–CNN, DETR, ViT, Swin Transformer, and CoTr are improving object detection. Researchers are moving from old models to Transformer models. They do this for better analysis of large data sets.
Spotting tiny lesions in unclear imagery is tough. Optimized models are needed. Hierarchical transformer models with a new mask mechanism are improving accuracy in finding small issues.
- The YOLOv3 algorithm and DeblurGANv2-InceptionResNet show promise for analyzing blurred images in diagnostics, with a 52.3% precision rate.
- Quick processing times are essential for analyzing healthcare data fast. Thanks to MIMO-UNet+ and HINet, real-time patient monitoring is more effective.
- Enhancing accuracy even with motion-blurred images shows the strength of these tools in real healthcare settings.
The developments in AI-powered object recognition systems are making healthcare better. They are improving personalized care with innovative computer vision applications.
Object Detection Algorithms: From Theory to Practice
Object detection algorithms used to be mainly for research and testing. Now, they're vital for many industries. They improve things like self-driving cars and medical tests. This shows how machine learning and computer vision applications have moved from ideas to real-world use.
YOLO and SSD are types of algorithms that work fast for real-time use. They handle images quickly in one step, which is great for jobs needing quick object detection.
Faster R-CNN is another method that's really good at spotting small and hidden objects. Even though they use a lot of resources, they're a big achievement in AI. They blend complicated algorithms with learning that's focused on results.
There's been big progress in object detection algorithms. New versions of YOLO have gotten faster and more accurate. This growth meets the higher needs of computer vision applications that want quick and correct results.
YOLO-NAS uses Neural Architecture Search to make better speed and accuracy. It's about big improvements in precision, raising the bar for real-time detection systems.
Improvements like the Darknet structure and techniques like Non-Maximum Suppression aim for more accuracy and efficiency. These upgrades help make object detection systems more reliable and able to handle different situations, from traffic analysis to robots working in changing places.
computer vision applications
These systems are now used for many tasks, from analyzing still images to spotting moving objects in videos. They show just how versatile and needed object detection algorithms are in today's industries.
How AI-Powered Object Recognition Systems Are Changing Security
Security is evolving thanks to AI-powered object recognition systems. These systems improve surveillance and access control. With real-time visual object detection, they're changing security management. They also boost our ability to detect threats quickly.
Facial Recognition and Access Control
Facial recognition is revolutionizing access control. This real-time object tracking technology identifies people fast and accurately. It offers a smooth and secure way into protected places. Places like companies and government buildings are now safer. This is because of these smart, AI-powered object recognition systems.
Anomaly Detection and Threat Management
Advanced object recognition software leads in keeping places safe. It spots unusual activity and threats efficiently. Because of this, security teams can understand situations better. With real-time visual object detection, spotting and managing threats has improved. This shows how crucial AI is for proactive security measures.
Technology | Application | Benefits |
---|---|---|
Real-Time Visual Object Detection | Surveillance Cameras | Minimizes response time to security incidents |
Facial Recognition | Access Control Systems | Streamlines secure entries with precise identity verification |
Anomaly Detection | Perimeter Security | Enhances perimeter defense with advanced alert systems |
Object Tracking | Mobile Patrols | Provides real-time location tracking of assets and personnel |
AI-powered object recognition systems are key in today's security. They help secure transactions and prevent bad actions. By using these systems, organizations protect their things better. They also create new standards for the security world.
Exploring Computer Vision Applications in Agriculture
Agriculture is changing thanks to computer vision applications. Real-time image processing solutions are more than just ideas. They're real tools already improving our farming methods. They help farmers and agribusinesses make smarter decisions, improve crops, and make food production better and more eco-friendly.
Consider the CIFAR-10 dataset, which has 60,000 color pictures of everyday items. Though first made for testing algorithms, now it helps create image processing solutions. These can spot plant diseases or lack of nutrients accurately. The huge ImageNet dataset, with over 14 million images in 21,841 categories, is also crucial. It helps in training models for detailed examination of crops.
The benefits of these tech tools are clear. For example, algorithms trained on the Microsoft COCO dataset can check crop health in ways we never imagined before. The Open Image dataset improves these models further. It supports deep learning that's changing how we look at and handle crop growth.
The impact of these tech advances is huge. In 2021, computer vision applications in the healthcare scene, which is similar to agriculture, were worth more than $3 billion. In farming, these technologies are also making a big difference. Some systems can now identify pests and weeds with up to 95% accuracy.
Looking ahead, computer vision applications are key to the future of farming. They help with everything from a single farmer's choices to worldwide farming policies. Thanks to rich datasets and strong models, we're getting better at monitoring crops. We can spot tiny changes in plant health, predict harvests, and cut down on waste. This is how we're moving towards a greener future in agriculture.
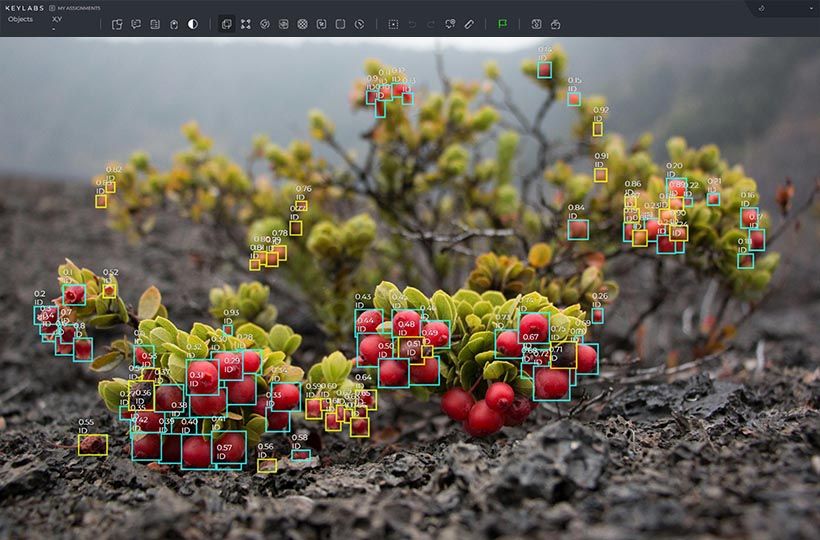
Real-Time Image Processing Solutions in Smart City Infrastructure
Smart cities are changing thanks to real-time object tracking technology and advanced object recognition software. These technologies are creating more efficient urban areas. They're changing traffic management and environmental monitoring for the better.
Deep Vision has processed 10 million images for the Global Emancipation Network (GEN). This has greatly improved their response times. Their tech analyzes images and videos frame-by-frame using an API on Amazon Web Services (AWS). This shows how powerful real-time image processing can be.
Traffic Management Systems
Computer vision tech improves urban mobility in traffic management. Deep learning algorithms help make traffic flow smoother and reduce jams. They provide real-time parking info and control traffic signals instantly.
These applications lower fuel use and cut greenhouse gas emissions. Also, they reduce commuter stress. Recurrent neural networks (RNNs) are key in predicting traffic flow, making transportation better.
Urban Planning and Environmental Monitoring
Computer vision applications and real-time image processing solutions are key in urban planning and environmental monitoring. They help public sectors understand demographics and traffic better. This reduces the time needed for analyzing data.
In environmental monitoring, vision cameras with special sensors track air quality in real time. Drones locate survivors more efficiently after disasters. This tech is making a huge difference.
Yet, we must be careful with these advances. The world's city population is growing, increasing the risk of data breaches in computer vision systems. Such breaches can lead to cyberattacks. Also, there's a problem with algorithm bias and lack of regulations. We need to use these technologies wisely to protect data and ensure fairness.
Understanding these tracking and processing technologies helps us make better choices for future smart cities. As technology advances, we must focus on ethics, data safety, and fairness. This will help make sure smart cities benefit everyone equally.
Edge Computing: Enhancing Real-Time Object Recognition
Edge computing is changing the game in real-time object recognition applications. It moves data processing closer to where it's needed, speeding up and improving real-time visual object detection. This change makes operations smoother and helps with quick, reliable decision-making right where it counts.
The Role of Edge AI in Object Detection
Edge AI takes computing power to the edge of the network. This makes real-time image processing solutions faster and more accessible. Now, edge devices can identify patterns, process images, and detect objects all on their own. This boosts how well and quickly they operate, without needing central servers.
Reducing Latency and Improving Efficiency
Edge computing sets new standards for speed and efficiency in real-time object recognition applications. It cuts down the time needed to process and understand video data. As industries demand faster real-time visual object detection, edge computing shows it's pushing performance to new heights.
Performance Metric | Value Achieved | Application/Model |
---|---|---|
Processing Speed (FPS) | 97.47 | YOLOv8s with Adaptive Frame Skipping |
Correct Count Rate | 82.57% | Surveillance/Industrial Monitoring |
F-score | 0.86 | Anomaly Detection/Autonomous Driving |
Summary
Emerging technologies are shaping our future, especially in real-time object recognition. These applications greatly boost efficiency and help in making better decisions. With an amazing accuracy rate of 97%, new systems are changing how industries work. They use deep learning and the SSD algorithm to achieve great accuracy and speed, making them key in modern industries.
Computer vision has seen big improvements thanks to AI and object recognition systems. Techniques like CNN and YOLO are now used beyond just research. They're helping with quality control and even in critical medical procedures. These advancements play a big role in security, like in watching over traffic, showing how vital computer vision is for our safety.
Understanding the role of these technologies in different fields is critical. Adopting AI-powered vision systems is changing the game. It's leading to better productivity, safety, and innovation. As we embrace these systems, we're entering a new era. This era will change how we view industry and technology forever.
FAQ
What are real-time object recognition applications?
Real-time object recognition applications detect and classify objects in images or videos instantly. They're used in many fields like video surveillance, self-driving cars, medical diagnostics, and store analytics. These tasks rely on advanced algorithms to analyze visuals as they occur.
How is real-time visual object detection influencing computer vision applications?
It's making computer vision much better by letting systems quickly identify and follow objects. This is key for AI apps which need to react to their surroundings. It helps in areas such as autonomous driving, security, and retail by processing visual information fast.
What advancements in object detection algorithms have been made recently?
The big progress in object detection algorithms comes from deep learning and AI. Techniques like convolutional neural networks, YOLO, and R-CNNs have greatly improved how these algorithms work. Now, they're faster and more accurate in identifying a wide range of objects.
How is AI-powered object recognition transforming manufacturing?
AI in manufacturing is a game-changer. It's used for monitoring and gives instant analytics, boosting productivity and safety. For example, it can spot defects, manage stock, oversee quality, and aid maintenance. This leads to better operations and more output.
What role does advanced object recognition software play in the development of autonomous vehicles?
Crucial for self-driving cars, advanced object recognition helps them see and understand the world. By identifying objects around, such as other cars and pedestrians, it helps vehicles decide where to go safely. This tech is essential for their operation and safety.
How are real-time object recognition applications utilized in healthcare?
In healthcare, these applications are vital. They help diagnose illnesses, watch patient health, and handle logistics. By recognizing medical conditions and tracking movements, they help patients get better faster and make care more efficient.
What is the impact of real-time object tracking technology on retail?
It's changing retail by offering insights into how customers act, improving stock control, and reducing theft. This tech boosts the shopping experience with tailored suggestions and better stock management. It improves customer service and protects the store's goods.
How is edge computing enhancing real-time object recognition?
Edge computing makes object recognition faster by processing data right where it's collected. This cuts delays and makes the systems more effective. With edge AI, recognition happens quicker, perfect for apps needing immediate responses, reducing the need for cloud computing's delays.
In what ways are AI-powered object recognition systems altering the security landscape?
They're revolutionizing security with better surveillance, like facial recognition and threat detection. These systems identify risks faster and more accurately. By managing security proactively, they greatly improve how secure an area is.
How do computer vision applications contribute to agricultural advancements?
Computer vision is a big help in farming by supporting precision agriculture. It watches over crops, fights pests, and studies growth. This leads to more crops, less wasted resources, and better farm operations. Agriculture becomes more efficient and sustainable with these tools.
What is the significance of real-time image processing solutions in smart city infrastructure?
Key for smart cities, real-time image processing aids in managing traffic, planning developments, and tracking environmental changes. It allows cities to respond immediately to various situations, improving traffic flow and the environment. This tech is vital for modern, responsive urban areas.
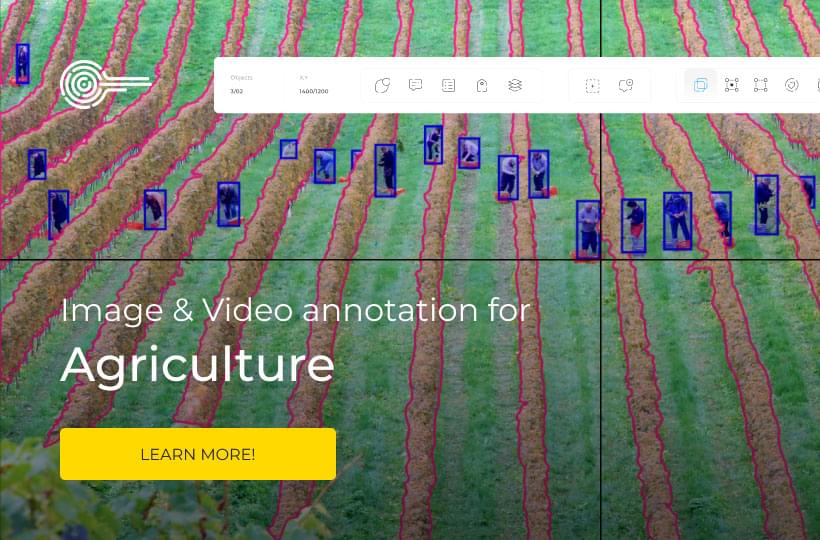