Retail & E-commerce Annotation: Enhancing Product Recognition and Personalization
E-commerce sales in the second quarter of 2024 reached 16 percent of total retail sales in the United States. These statistics from the U.S. Census Bureau emphasize the growing importance of digital retail. Companies now use AI for retail and e-commerce data to stay competitive.
Annotation in retail involves labeling images, text, and other forms of data to train machine learning models that can accurately recognize products, understand customer behavior, and enable intelligent automation. As product catalogs expand and user interactions increase, the need for accurate and scalable annotations becomes critical.
Key Takeaways
- E-commerce sales now represent over 16% of total retail sales.
- Data annotation is crucial for accurate product recognition.
- Retail AI drives personalized shopping experiences.
- Advanced annotation techniques improve product visibility.
- Understanding e-commerce data is essential for business growth.
- Tailored customer experiences are key to staying competitive.

Definition and Importance
Retail and e-commerce annotation refers to labeling data such as product images, descriptions, customer reviews, and user interactions to make them understandable to machine learning algorithms. This labeled data trains models that recognize products, categorize content, and personalize the shopping experience based on customer behavior. For example, an image of a shoe might be tagged with tags such as "sneakers", "red", and "leather", while a customer review might be tagged with a sentiment or intent. Without this foundation, intelligent automation in retail would lack the accuracy and relevance consumers now expect.
The importance of annotation in retail and e-commerce lies in its ability to make AI systems more responsive, accurate, and aligned with user expectations. With millions of products and an ever-increasing amount of data, companies rely on annotated datasets to simplify search, improve recommendations, and reduce friction in the customer journey. With high-quality annotations, features such as virtual trials, visual search, and chat-based assistants work reliably to create richer, more interactive experiences.
Benefits for Businesses
Annotation in retail and e-commerce provides businesses with a solid foundation for effective, revenue-generating AI use. One key benefit is improved product visibility through more intelligent search and filtering. When product images and descriptions are accurately labeled, search engines and recommendation systems become much more efficient, helping customers find what they're looking for with less effort. This not only improves the shopping experience but also increases conversion rates and reduces bounce rates.
Another essential benefit is personalization, which has become a central requirement of modern e-commerce. Annotated data on user behavior, such as clicks, purchases, or time spent on a page, allows AI systems to learn and predict individual preferences. This leads to more accurate product suggestions, customized promotions, and dynamic content that speaks directly to each customer. As a result, companies see higher customer satisfaction, repeat visits, and stronger brand loyalty.
In addition to customer engagement, annotation also drives internal efficiency and innovation. Tagged data helps automate product classification, inventory tracking, fraud detection, and customer service via chatbots. This reduces the workload of human teams and minimizes errors, allowing companies to scale operations smoothly.
The Role of Data Annotation in Retail
Data annotation enables AI to work effectively in a retail environment. Retail often involves tagging images with product categories, attributes, and styles and tagging textual data from reviews, queries, or customer service messages. These annotations allow machine learning models to "understand" what they are analyzing and make decisions based on patterns in the data. Whether recognizing a handbag in an image or interpreting the tone of a customer's response, annotation is the key to turning data into actionable insights.
Retail operations increasingly depend on automation to process vast amounts of product and customer data. Annotated data makes this automation possible by training AI systems to sort, categorize, and respond accurately. Similarly, annotated user experience data enables recommendation engines to predict what a customer will likely buy next.
As product lines evolve and consumer behavior changes, annotations must be updated and refined to reflect new trends and expectations. Retailers maintaining high-quality annotated datasets can adapt to market changes faster, introduce more innovative features, and continuously improve the customer experience.
Data-Driven Customer Insights
By tagging user actions, such as searches, clicks, purchases, product reviews, and browsing patterns, retailers can understand what customers are doing and why they are doing it. For example, annotated sentiment in reviews shows how people feel about specific products, while labeled navigation data shows which parts of a website or app are most appealing. The result is a more nuanced view of customer needs, behaviors, and expectations.
By analyzing annotated data, retailers can make better decisions at both the strategic and operational levels. Marketing campaigns become more targeted as companies know which messages resonate with a particular audience. Product development teams can track feedback trends and adjust features based on real user data. Even customer service can improve as annotated conversations help identify recurring issues and optimize support workflows.
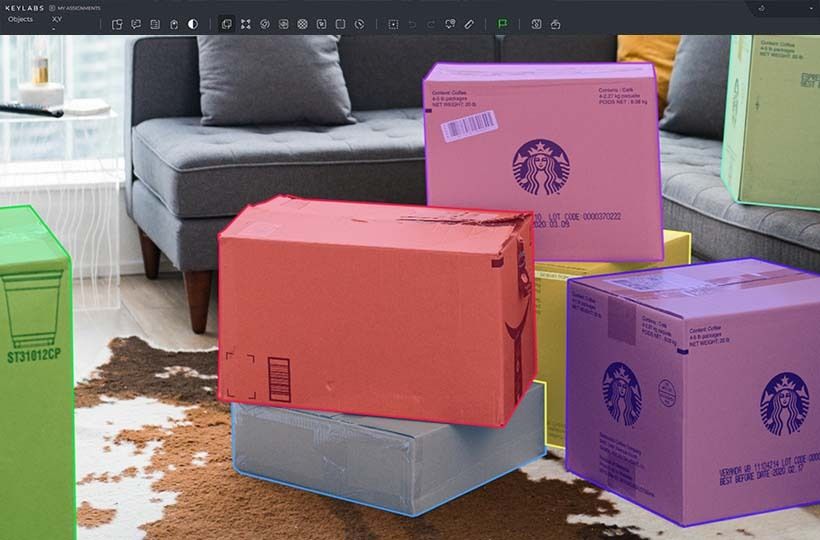
Challenges in Retail Data Annotation
- One of the most pressing challenges is the sheer volume and variety of retail data. Maintaining consistent labeled data sets is a massive undertaking with thousands of products, numerous variations, and constantly changing inventory. Minor inconsistencies in labeling, such as different definitions of color shades or styles, can lead to unreliable model output and customer-facing errors. As product catalogs evolve rapidly, keeping the annotation up to date becomes time-consuming and time-sensitive.
- Another major challenge is the complexity of the annotation tasks themselves. Annotating an image, for example, may require bounding boxes, segmentation, or attribute tagging, all of which require accuracy and subject matter expertise. Textual annotations add their layer of complexity, especially when interpreting sentiment, intent, or context in user reviews and queries, which are often subjective or nuanced. It can be difficult for annotators to maintain consistency across large datasets, and labeling errors can degrade model performance over time.
- Privacy and ethics issues add a layer of complexity, especially when annotating data about user behavior. Retailers must be guided by data protection and consent regulations, ensuring customer information is handled responsibly. In cases where annotation is outsourced, maintaining data security and training annotators to understand the product and brand context is also challenging. Overcoming these challenges requires a combination of human oversight, intelligent tools, and iterative feedback loops to ensure that annotations are supported in robust, scalable, and reliable retail AI systems.
Best Practices for Effective Annotation
One of the main best practices is to follow clear and consistent labeling guidelines that define precisely how each data type should be labeled. This ensures that all internal and external annotators follow the same standards, reducing ambiguity and increasing data reliability. Consistency between annotation tasks leads to cleaner training data, directly affecting the model's accuracy and performance.
Another essential practice is to combine automation with human supervision. Tools that pre-label data using basic algorithms or pre-trained models can significantly speed up annotation. Quality control mechanisms, such as random sampling, cross-checking, and consistency checks between annotators, should be built into the workflow to detect and correct errors in advance. This hybrid approach helps to maintain quality while scaling annotation efforts to meet growing data needs.
Quality Control Measures
One of the most effective quality control measures is to create a review cycle where a second set of eyes regularly checks annotations for accuracy and consistency. This may involve sampling a percentage of the tagged data for manual review or comparing annotations between multiple workers to identify discrepancies. These checks help identify common issues, such as misclassified products, mismatched tags, or missing labels, which can degrade model training if left unchecked.
Another essential metric is inter-annotator agreement, which measures how consistently different annotators label the same data. The high deal indicates that the instructions are clear and that the annotators understand the task, while the low agreement may reveal ambiguity or the need for additional training. This metric is handy for subjective tasks such as sentiment analysis or attribute classification, where interpretation can vary.
Automation also plays a vital role in maintaining quality at scale. Pre-annotation tools and anomaly detection algorithms can flag outliers or unusual patterns in the data, prompting human review before these issues affect the model. Tracking performance metrics such as accuracy, recall, and model feedback also helps close the loop between annotation quality and system performance.
Summary
Data annotation has become a cornerstone of innovation in retail and e-commerce, enabling AI systems to understand products, interpret customer behavior, and deliver personalized experiences. From enabling visual search to optimizing recommendation engines, accurate annotations turn raw data into actionable insights that drive customer satisfaction and operational efficiency. However, the effectiveness of these systems depends on the quality of the annotated data, which requires well-defined processes, skilled oversight, and continuous improvement. Retailers that invest in effective annotation practices improve their current AI capabilities and prepare for the future in a competitive and rapidly evolving digital environment. As customer expectations rise and product ecosystems expand, high-quality annotations will continue to be the foundation of smarter, more responsive retail.
FAQ
What is retail and e-commerce data annotation?
Retail and e-commerce data annotation involves labeling and categorizing data like images, text, and video. This process trains AI models for the retail sector.
How does data annotation improve product recognition in retail?
Data annotation boosts product recognition through advanced image labeling and text tagging. AI systems can then accurately identify and categorize products using visual data.
What are the benefits of data annotation for e-commerce businesses?
Data annotation offers numerous benefits for e-commerce businesses. It improves customer insights, optimizes inventory management, enhances forecasting, and enables personalized shopping experiences.
What are the main challenges in retail data annotation?
Key challenges in retail data annotation include data quality concerns and scalability issues. Privacy and regulatory compliance are also significant concerns.
What are some best practices for practical data annotation in retail?
Practical data annotation in retail involves maintaining consistency and implementing quality control measures.
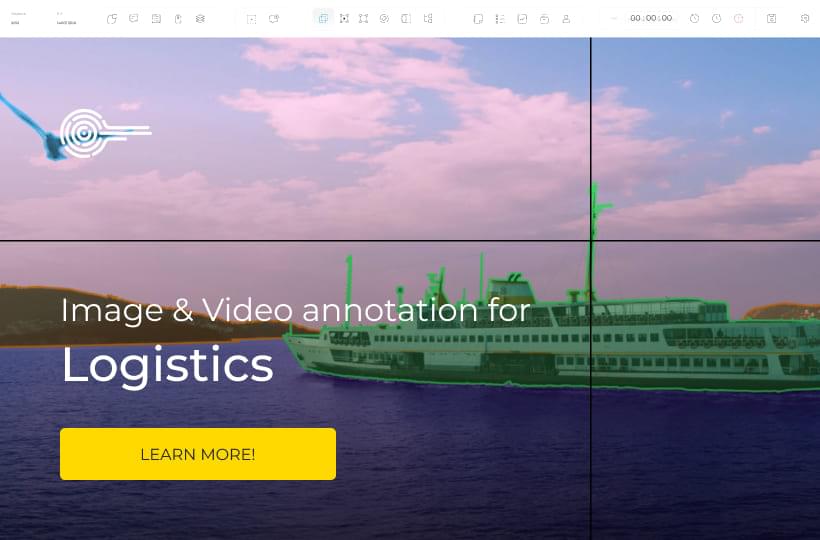