Revolutionizing Industries: Real-World Applications of AI Image Recognition
In an era where retail giants use technology to stay ahead, a staggering statistic propels the industry into the future: the global image recognition market in retail is expected to reach $38.9 billion by 2025. This explosive growth, identified by MarketsandMarkets, is driven by the urgent need for sophisticated inventory management systems. Fusing artificial intelligence (AI) with image recognition, businesses across diverse sectors are transforming their operations, capitalizing on AI image recognition use cases to enhance user experiences and refine decision-making.
Where customer convenience dictates market trends, image recognition technology becomes a game-changer, as 59% of consumers lean towards purchases augmented by reality, suggests Deloitte's research. This integration of AI is not just altering how AI is changing industries but is defining the future of consumer interaction.
Key Takeaways
- The retail image recognition market's substantial financial projection emphasizes the growth of AI in inventory management.
- Increased consumer spending due to augmented reality showcases the direct impact of image recognition on the retail experience.
- Significant advancements in healthcare indicate a promising trajectory for AI in medical image analysis and diagnosis.
- Quality control in manufacturing via AI can dramatically reduce errors and costs, evidencing the pragmatic applications in various industries.
- Image recognition is a crucial component in various sectors, from healthcare, where it promises to revolutionize patient care, to traffic management systems that could save lives by reducing congestion and accidents.

Embracing the Future: The Rise of AI Image Recognition in Tech
The fusion of artificial intelligence (AI) with computer vision is spearheading technological revolutions, by facilitating perceptual capabilities in machines that mirror human sight but with superior accuracy and efficiency. This powerful synergy, prominently featuring AI image recognition use cases, underscores a transformative phase in technology, refining the precision with which machines understand and interpret visual data.
Computer vision integration allows for nuanced advancements in multiple domains including security surveillance, facial recognition systems, and enhancing user engagement platforms. With AI-driven algorithms, notably convolutional neural networks (CNNs), at the forefront, there is a marked enhancement in the processes of feature extraction, image processing, and the continuous evolution of scene comprehension and object identification technology.
As AI continues to evolve, the applications of AI image recognition become increasingly dynamic. Surveillance technologies have become more adept at recognizing faces in varying lights and angles, augmenting security measures. In the field of interactive media and entertainment, computer vision is used to track user interactions and improve the responsiveness of augmented reality (AR) environments, creating immersive user experiences.
Decoding Neural Networks: The Brains Behind AI Image Recognition
The intricate world of neural networks signifies a monumental leap in how AI is changing industries, particularly in the realm of AI image recognition. Originally proposed in 1944, these networks have evolved from simple structures to complex models simulating human cognitive functions. They are structured in three fundamental parts: the input layer, various hidden layers, and the output layer. Each neuron in these layers processes data through activation functions, replicating aspects of human intelligence.
One of the standout capabilities of neural networks includes unsupervised learning, which allows them to detect hidden patterns in unlabelled data autonomously. This facet of deep learning is crucial in handling unstructured data across numerous sectors like social media and online shopping, enabling features like facial recognition on Facebook and personalized recommendations on Amazon.
The progression in neural networks is propelled by the heightened processing power of advanced graphics chips, which has rejuvenated research efforts and actual applications. The travel from theoretical to practical application sees neural networks contributing vastly to areas like speech recognition and natural language processing. Despite their widespread utilizations, these networks face issues like overfitting and the black box nature, which obscures their decision-making processes from easy interpretation.
Transforming Healthcare with AI-Powered Diagnostic Imaging
The intersection of artificial intelligence (AI) and healthcare is revolutionizing the way medical professionals approach diagnostics and treatment, particularly through advanced AI applications in medical image analysis. This fusion not only enhances the accuracy of diagnoses but also streamlines the process of drug development, vastly improving patient care and healthcare outcomes.
Enhancing Medical Diagnosis Accuracy
One of the standout healthcare AI applications involves leveraging AI to improve the precision of medical diagnoses. Utilizing sophisticated algorithms, AI-powered medical imaging tools are capable of analyzing complex visual data, allowing them to detect anomalies that might be overlooked by the human eye. Research has shown that AI models are achieving accuracy rates as high as 98.56% in brain tumor classification, a testament to their efficacy in enhancing medical diagnostic processes. This significant advancement in medical image analysis not only supports doctors in making more accurate diagnoses but also contributes to better prediction and early intervention in diseases such as cancer and cardiovascular conditions.
Streamlining Medical Imaging Analysis
AI's role in medical image analysis is invaluable in managing the high volumes of data generated by modern diagnostic imaging techniques. AI technologies help in the real-time tracking of changes in a patient's condition, considerably reducing the time needed for diagnosis. For instance, in stroke cases, AI tools have cut down the time between CT angiography and treatment intervention, significantly enhancing patient recovery rates. Additionally, these AI-driven systems alleviate the strain on medical staff by reducing workloads and preventing burnout, thereby addressing both healthcare efficiency and employee well-being.
Accelerating Drug Discoveries and Development
In the realm of pharmaceuticals, AI in drug development is proving to be a transformative force. AI algorithms are not only speeding up the drug discovery process but are also making it more cost-effective. By predicting the efficacy of certain drug compounds earlier in the drug development cycle, AI minimizes the risk of late-stage failures. Moreover, AI applications extend to personalized medicine, where they analyze patient genetic data to tailor treatments that are much more effective, ushering in a new era of customized healthcare solutions.
The integration of AI in healthcare applications, especially in diagnostic imaging and drug development, promises a future where medical practice is safer, quicker, and much more precise. This reliance on advanced AI technologies not only forecasts a significant reduction in diagnostic errors but also a revolution in how treatments are approached and administered globally.
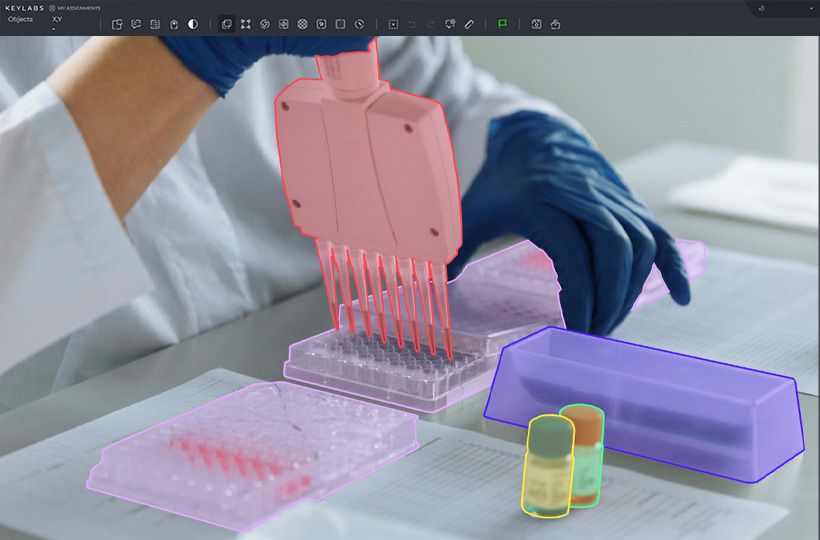
Revolutionizing Finance & Banking: Risk Management and Fraud Detection
In the ever-evolving financial sector, AI in finance is playing a pivotal role in reshaping risk management and fraud detection strategies. Its ability to process and analyze vast amounts of data with accuracy and speed is unmatched, enabling institutions to handle complex risk assessments and secure financial transactions more effectively.
AI Application | Impact |
---|---|
Fraud Detection | Banks using AI have seen a dramatic decrease in economic losses related to financial fraud. |
Credit Risk Assessment | Diverse data sources such as financial statements and credit histories are analyzed to accurately gauge credit risks. |
Money Laundering Detection | Enhanced detection of illicit activities through sophisticated pattern analysis of transaction data. |
Cybersecurity | Abnormal network behavior is rapidly identified, protecting sensitive data from cyber threats. |
Market Risk Analysis | Data from various sources is synthesized to predict potential market risks effectively. |
Operational Risk Management | Streamlined processes lead to reduced manual errors and improved operational efficiency. |
The applications in various industries, especially finance, are vast and continuously expanding. For instance, platforms like Wells Fargo's personalized account insights and RBC's Aiden for trading decisions showcase AI's transformative capabilities in banking.
Retail and E-Commerce Evolutions: Personalized Shopping with AI
The rapid integration of AI in retail and e-commerce is not just transforming but revolutionizing the landscape. Through advanced AI image recognition use cases, the sector is experiencing a shift towards more personalized shopping experiences and efficient inventory management. This adoption not only enhances customer satisfaction but also streamlines operations, making shopping seamless and more intuitive.
Enriching Customer Experience with Visual Searches
In the digital shopping realm, AI-powered visual search technologies have become indispensable tools. These technologies allow customers to upload images to search for products, bypassing the need for text-based queries and enhancing the shopping experience. This sophisticated search capability, driven by AI image recognition, taps into the consumer's desire for convenience, speed, and personalization, significantly boosting e-commerce revenue.
Optimizing Inventory Management Through Image Recognition
Efficient inventory management is critical to the success of any retail operation. AI excels in this area by deploying predictive analytics to forecast demand and manage stock levels effectively. By analyzing vast amounts of historical sales data and external factors like market trends and seasonality, AI supports retailers in maintaining just the right amount of stock to meet consumer demand without overstocking, thereby reducing waste and saving costs.
Below is a comparative analysis of how AI is transforming inventory management:
Feature | Impact | Outcome |
---|---|---|
Predictive Analytics | Forecasts demand accurately | Reduces overstock and understock scenarios |
Visual Search | Enhances product discovery | Increases sale conversion rates |
Real-time Data Processing | Adjusts inventory dynamically | Optimizes supply chain efficiency |
Enhancing Manufacturing Excellence: Quality Control with AI Vision
The advent of AI in manufacturing has redefined traditional quality control systems, bringing about a transformative shift in operational efficiency and product accuracy. Employing AI vision technology integrates advanced image recognition capabilities, substantially lowering error rates and facilitating a leaner manufacturing process.
Automating Defect Detection in Real-Time
AI vision systems revolutionize the way manufacturers handle quality control by automating the detection of defects in real-time. This not only accelerates the production timelines but also enhances the accuracy of inspections, substantially outperforming traditional human checks. The integration of real-time data analytics aids in immediate decision-making and process adjustments, ensuring continuous quality enhancement across the manufacturing sector.
Enabling Intelligent Automation in Assembly Lines
Incorporating AI vision systems in assembly lines allows for intelligent automation, fostering a seamless flow in manufacturing processes. These systems are adept at recognizing complex patterns and executing precise assembly tasks, leading to a redefined workspace that prioritizes safety and efficiency. Such applications in various industries highlight AI's capability to adapt and evolve in response to specific manufacturing environments.
Feature | Impact on Quality Control | Impact on Productivity |
---|---|---|
AI Vision Accuracy | Enhanced precision in defect identification | Minimized disruptions, smoother workflows |
Data Analytics | Real-time insights for immediate corrections | Optimized processes based on analysis |
Pattern Recognition | Consistent and reliable defect detection | Reduction in manual re-checks and delays |
By leveraging cutting-edge AI vision systems, manufacturing sectors witness a steep decline in operational costs and enhanced product quality. The trend towards integrating AI in quality control not only exemplifies the practical applications in various industries but also sets a new standard in manufacturing excellence.
Safeguarding the Urban Landscape: AI Image Recognition in Surveillance
The integration of AI image recognition use cases in urban surveillance with AI has significantly advanced the capabilities of monitoring and security systems globally. Employing sophisticated computer vision technologies ensures a robust defense against threats, providing a sense of security to urban populations.
As pivotal tools for law enforcement, AI-driven surveillance systems utilize facial recognition technology to identify persons of interest across crowded public spaces swiftly. This application is supported by global statistics indicating a significant drop in identification error rates over recent years, enhancing the reliability of security efforts in dense urban areas.
Moreover, smart city initiatives utilize these technologies to manage crowd densities and monitor suspicious activities, a direct response to the growing demands for smarter, safer urban environments. AI technologies are competent in interpreting vast amounts of visual data from surveillance cameras, turning the collected footage into actionable insights.
Feature | Application | Impact |
---|---|---|
Facial Recognition | Identification in public spaces | Reduces criminal activities and enhances public safety |
Object Recognition | Monitoring compliance (e.g., social distancing) | Supports public health and safety regulations |
Behavior Analysis | Detecting suspicious activities | Prevents potential threats in real-time |
The advancements in AI image recognition use cases, particularly in urban surveillance with AI, continue to revolutionize security measures and foster a safer urban environment worldwide. This technology not only enhances the efficiency of surveillance but also supports the crucial function of law enforcement in maintaining public safety.
Charting New Frontiers: Advanced Analytics with AI Visual Data
As industries pivot towards a data-driven approach, advanced analytics powered by AI have become indispensable. Harnessing AI for data visualization not only enhances decision-making but also unlocks the vast potential of visual big data. The integration of AI advanced analytics is witnessing unprecedented adoption across sectors, facilitating more nuanced and swift insights into vast and complex datasets.
With the capability to process and analyze visual data in real-time, industries are tapping into real-time video analytics to derive actionable insights that were previously inconceivable. This technological leap is bolstering fields as diverse as autonomous driving and urban planning, where immediate data interpretation is crucial.
Driving Innovations with Real-Time Video Analytics
Real-time video analytics represent a significant leap forward in monitoring and response strategies. Whether it's optimizing traffic flow in smart cities or enhancing security protocols, the ability to analyze video data on the fly is revolutionizing how operations are conducted. AI's role in processing real-time data ensures not only speed but also accuracy, pushing the boundaries of traditional surveillance and operational monitoring.
Unlocking Patterns in Visual Big Data
The fusion of AI with visual big data analytics is forging pathways that were once blocked by the sheer scale and complexity of the data involved. This synergy is crucial in sectors like healthcare, where visual data can provide insights into patient diagnostics and treatment plans, or in retail, where consumer behavior analytics shape the future of shopping experiences. AI advanced analytics play a pivotal role in distilling this vast amount of data into comprehensible and actionable insights, driving efficiency and innovation.
The application of AI in visual data is not just transforming existing industries but also creating new opportunities in fields such as sports analytics and entertainment, where enhanced analytic capabilities can provide a deeper understanding of performance dynamics and audience preferences. As AI continues to evolve, its integration with advanced analytics will further empower professionals and revolutionize industry standards.
Understanding the Complexities: Ethical Challenges of AI Image Recognition
As AI image recognition technology continues to evolve, it increasingly raises a myriad of ethical challenges that must be navigated with caution and responsibility. From privacy concerns to issues of bias and fairness, the use of AI in image recognition is a double-edged sword, offering both remarkable capabilities and significant ethical dilemmas.
Addressing Data Privacy and Security Concerns
The integration of AI in image recognition heightens the potential for invasive surveillance and privacy invasions, challenging the balance between innovative advancements and individual privacy rights. Companies incorporating these technologies need a framework ensuring that data usage adheres strictly to data protection regulations to prevent misuse.
Contending with Bias and Transparency in AI Systems
Another significant ethical challenge of AI is the risk of perpetuating existing biases. Skewed training data can lead AI systems to develop biased algorithms, affecting fairness and impartiality in automated decisions. Moreover, the lack of transparency in how these algorithms operate can make it difficult to ascertain and rectify these biases, making it essential for companies to commit to diverse data sources and clear, interpretable decision processes.
Issue | Description | Impact | Recommended Actions |
---|---|---|---|
Privacy Concerns | Potential for invasive surveillance | Compromise of personal privacy | Implement stringent data protection measures |
Bias and Fairness | Algorithmic bias due to skewed data | Unfair treatment and discrimination | Ensure diversity in training datasets |
Misuse and Manipulation | Rising use of deepfakes | Spread of misinformation | Enhance verification processes |
Transparency and Accountability | Opaque AI decision-making processes | Trust issues among users | Adopt clear and interpretable algorithms |
Regulatory Compliance | Rapid evolution of AI technologies | Legal and ethical violations | Stay updated with evolving regulations |
To truly benefit from the advantages of AI image recognition and handle the associated ethical challenges of AI, a collaborative effort among developers, policymakers, and the community is essential. It ensures that AI advancements improve society commensurately with maintaining ethical standards and social trust.
Summary
With visual search statistics indicating that a majority of the information our brains process is visual and millennials' strong preference for visual search capabilities, it's no surprise that retailers in regions like the UK are increasingly incorporating this technology to stay competitive and cater to evolving consumer behaviors.
Indeed, the future of image recognition in business looks incredibly promising. Implementations range from advanced object recognition systems in consumer apps like Google Lens to the utilization of facial recognition for bolstering security at airports and ensuring customer authenticity in the banking sector. As these applications become more widespread, the ethical implications surrounding AI image recognition necessitate a concerted effort for responsible innovation and adherence to privacy standards. This ensures that as businesses harness the efficiency and accuracy of AI – up to a remarkable 99% in some models – they also build trust and maintain accountability to their stakeholders.
FAQ
What are some real-world applications of AI image recognition across industries?
AI image recognition is making an impact in healthcare with diagnostic imaging, in finance by detecting fraud, in retail with personalized shopping experiences and inventory management, in manufacturing for quality control, and in public safety with enhanced surveillance capabilities.
How is computer vision integration changing the technology landscape?
Computer vision integration enables machines to process and interpret visual data with human-like perception, but at a faster speed and with greater accuracy. This transformation is crucial in fields like facial recognition, surveillance, and enhancing user engagement.
Can you explain how neural networks contribute to AI image recognition?
Neural networks, which mimic the structure and function of the human brain, process complex patterns in data for tasks like image recognition. By using multiple layers, they enable hierarchical data representation, improving AI's capability to identify features and process visual information.
How is AI transforming healthcare, particularly in diagnostic imaging?
AI in healthcare, particularly through deep learning models, enables more accurate disease diagnosis and efficient drug discovery. It also contributes to personalized treatment plans by analyzing medical images and patient data quickly and more precisely.
In what ways is AI enhancing risk management and fraud detection in finance and banking?
AI in finance is pivotal for analyzing large datasets to detect unusual patterns that may indicate fraudulent activity and for automating risk management processes. These advancements help in making more informed investment predictions and in maintaining secure transactions.
How is AI image recognition reshaping the retail and e-commerce industry?
AI image recognition is revolutionizing retail by enabling visual search capabilities and augmented reality experiences, thereby influencing consumer behavior. It also plays a vital role in optimizing inventory management and enhancing customer experience.
What are the use cases of AI image recognition in business intelligence?
AI image recognition in business intelligence includes real-time object detection, movement tracking, pattern recognition, and advanced data analytics. These capabilities aid in better decision-making and in operational efficiency across various industries.
How does AI vision contribute to quality control and defect detection in manufacturing?
In manufacturing, AI-driven image recognition systems facilitate the automation of defect detection, leading to a significant decrease in error rates and an increase in production quality. They also promote intelligent automation along production lines, enhancing manufacturing excellence.
How is AI improving public safety and security through urban surveillance?
AI image recognition is advancing urban surveillance by enhancing monitoring capabilities, supporting law enforcement in behavioral analysis, and contributing to a safer public environment with its constantly evolving technology.
Can you discuss the impact of AI image recognition on advanced analytics and decision-making?
Advanced analytics are greatly impacted by AI image recognition as it unlocks patterns within visual big data and provides insights for real-time video analytics. This is essential for driving operational efficiencies, autonomous driving, sports analytics, and strategic decision-making in various fields.
What ethical challenges arise with the use of AI image recognition?
Ethical challenges include concerns around data privacy, security, user consent, transparency, and the potential for algorithmic bias. Addressing these challenges is crucial for companies to ensure the ethical application of AI image recognition technologies.
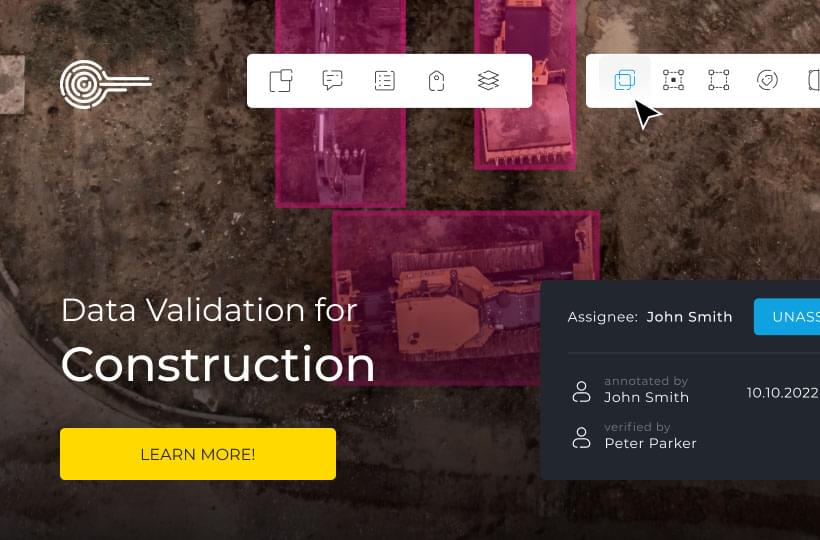