Streamlining Media Production with AI-Driven Image and Video Annotation
The integration of AI-driven annotation in media production is transforming content creation, management, and delivery. Our technology boosts media professionals' ability to handle vast visual content efficiently and accurately. AI-driven media content annotation automates tasks like tagging, categorizing, and analyzing visual data. This not only improves media product quality but also enhances viewer engagement through personalized content and better discoverability.
Manual video annotation is labor-intensive, requiring annotators to label thousands of frames in large datasets. Human error can affect data accuracy due to fatigue and repetitive tasks. As AI applications expand, annotating large-scale video data becomes resource-intensive and costly. Automated tools alleviate human annotators' workload by handling repetitive tasks like object tracking, making real-time video annotation essential for surveillance and live events. Techniques like 3D and keypoint video annotation capture more complex data.
Key Takeaways
- AI-driven media production improves efficiency and precision in content creation.
- Automated image annotation enhances viewer engagement through personalized recommendations and better content discoverability.
- Manual video annotation is prone to human error and time-consuming.
- Automated tools reduce workload and increase the accuracy of AI systems.
- Real-time and advanced annotation techniques support complex applications like surveillance and sports analytics.

Understanding Media Content Annotation
Media content annotation is the act of labeling media like videos and images with metadata. This metadata describes the content or context of the media. It's essential for organizing large amounts of media and making content search and retrieval effective. As unstructured data grows, so does the need for annotation in data processing, critical for machine learning systems.
Definition and Importance
This process involves categorizing media files with metadata, providing detailed insights into each file's content. It's vital for streamlining media workflows, enabling quick and accurate content tagging and retrieval. For instance, in autonomous vehicles and medical imaging, precise image classification and object detection are critical for safety and efficiency.
The New York Times and other media giants have adopted annotated approaches in journalism. This method is highly relevant in today's digital landscape. It benefits journalism and media production broadly.
Key Benefits for Production Teams
Media content annotation brings numerous advantages to production teams. Here are some key benefits:
- Improved Efficiency: Content tagging automates much of the organizational workload, reducing manual intervention in media workflows.
- Enhanced Searchability: Tagging makes content retrieval precise and swift, simplifying the search for specific media files in large datasets.
- Targeted Content Delivery: Annotated media enables personalized and targeted content delivery, effectively catering to specific audiences.
The Role of AI in Media Annotation
In today's fast-paced media landscape, AI technologies have revolutionized how we analyze and categorize content. Advanced methods like machine learning, computer vision, and deep learning have transformed media analysis. They automate tedious annotation tasks and significantly enhance accuracy.
Types of AI Technologies Used
AI technologies include various tools and methods that streamline media annotation:
- Machine Learning: Enables systems to learn from data and improve over time, ensuring more precise automated annotation.
- Computer Vision: Allows for the automatic identification and tagging of objects and scenes in images and videos, broadening the scope of media analysis.
- Deep Learning: Utilizes neural networks to process and understand complex patterns in media files, boosting the AI accuracy of annotation.
By leveraging these technologies, media companies can efficiently handle vast amounts of data. They categorize and tag content with unparalleled precision.
How AI Enhances Accuracy
The infusion of AI into media annotation has greatly improved accuracy levels:
- Consistent Learning: AI systems continuously adapt to new data, ensuring that annotations become increasingly accurate over time.
- Reduced Human Error: Automated annotation minimizes the inconsistencies often found in manual processes, providing more reliable results.
- Advanced Recognition: Technologies like computer vision and deep learning identify and tag media content with a level of detail that surpasses human capabilities.
Common Use Cases for Media Content Annotation
Media content annotation is vital across various sectors. It aids in managing social media content, refining marketing strategies, and boosting sports analytics. With the daily generation of 328.77 million terabytes of data, these applications are key for efficient data use.
Social Media Management
In social media, annotation is essential for organizing and tagging content. It boosts user engagement by ensuring the right metadata is attached to each post. Platforms like Facebook and Instagram employ AI for content suggestions, sentiment analysis, and brand tracking. By using ML-assisted annotation, creators can streamline their work and make content more accessible.
Advertising and Marketing
Marketing success depends on precise audience targeting and thorough campaign analysis. Media annotation helps marketers tag videos and images to understand consumer preferences. This leads to better audience segmentation and more personalized ads. Companies like Google and Amazon use this data to create more effective ads.
Sports Analytics
Sports analytics greatly benefit from video annotation. It allows for in-depth analysis of games and player performance. By annotating videos, analysts can uncover insights on player movements and game strategies. Professional teams like the NBA and FIFA use this data to improve coaching and training, leading to better game strategies and player performance.
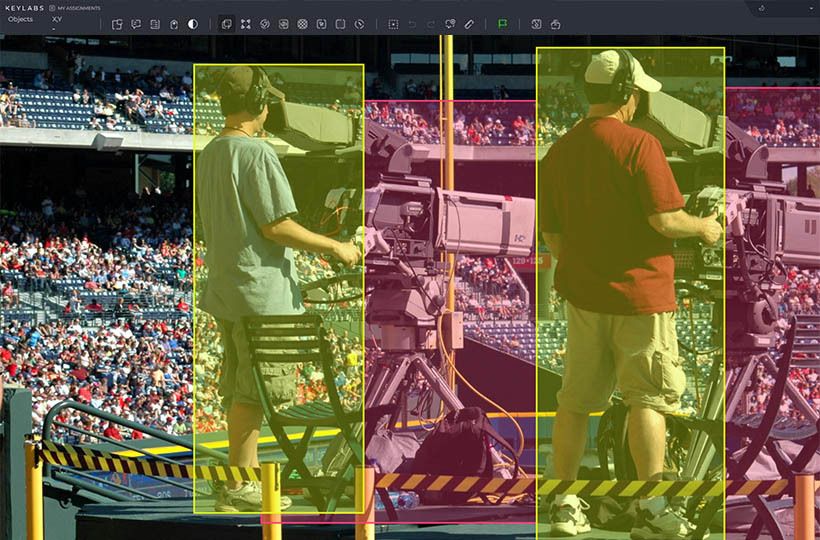
Comparison of Manual vs. AI-Powered Annotation
In media production, manual annotation and AI-powered annotation show stark contrasts in efficiency in media production and effectiveness. This section explores these differences, highlighting the unique characteristics of each approach.
Time Efficiency
Manual annotation is time-intensive, requiring extensive human effort. Large datasets can lead to significant delays as each item needs to be labeled by hand. In contrast, significantly cuts down labeling time. Automated tools can handle vast data sets at speeds manual methods cannot match, providing a notable advantage in speed. While manual methods falter with big tasks, AI solutions offer quick content processing, boosting media production efficiency.
Cost-Effectiveness
Manual annotation is costly due to the need for large human resources. For big projects, the financial strain is considerable. On the other hand, AI-powered annotation offers cost reduction strategies. The initial AI technology cost is high, but long-term savings from lower labor costs are substantial. Automated annotation reduces operational costs and scales well for large datasets.
The table below compares manual and automated annotation methods based on several key criteria:
Criteria | Manual Annotation | AI-Powered Annotation |
---|---|---|
Time Required | High | Low |
Cost | High | Low (long-term) |
Scalability | Limited | High |
Consistency | Variable | High |
Adaptability | High | Moderate |
The decision between manual annotation and AI-powered annotation depends on factors like dataset size, complexity, budget, and project timeline. Knowing the strengths and weaknesses of each method helps in creating strategies that boost media production efficiency.
Best Practices for Implementing AI Annotation
Integrating artificial intelligence into media annotation processes can drastically enhance efficiency and accuracy. To ensure a seamless transition and effective utilization, it is vital to follow specific best practices in AI implementation.
Setting Clear Objectives
Defining clear objectives at the onset is fundamental for successful implementation of AI annotation. We must establish what we need from AI—whether it's speeding up processes, improving accuracy, or handling various data formats. Objectives act as a guiding framework for strategic AI deployment.
- Speed: Tools like Snorkel Flow can accelerate annotation by generating labeled data programmatically.
- Accuracy: Methods such as bounding box annotation, polygonal segmentation, and key point tracking enhance image annotation.
- Diverse Data Formats: We must consider tools capable of handling text, video, and audio annotations efficiently.
Choosing the Right Tools
Choosing appropriate AI tools for media annotation is critical for meeting production needs. When considering AI tools for media, factors such as scalability, user-friendliness, and alignment with existing workflows should be evaluated. Implementation should be accompanied by thorough staff training and a gradual integration to ensure a smooth transition.
Annotation Type | Tool Features | Benefits |
---|---|---|
Image Annotation | Supports bounding boxes, polygonal segmentation, and key point tracking. | Increases accuracy and consistency in labeling. |
Video Annotation | Frame-by-frame labeling for object tracking and activity recognition. | Provides thorough analysis of video content. |
Text Annotation | Handles sentiment analysis, entity annotation, and intent classification. | Enhances natural language processing capabilities. |
Audio Annotation | Enables training for voice recognition and speech-to-text models. | Vital for developing advanced audio processing tools. |
By adhering to these best practices in AI, we ensure that the strategic AI deployment aligns with organizational objectives and maximizes the use of AI tools for media.
Challenges in Media Content Annotation
AI-driven annotation brings many benefits, but it also faces challenges in media annotation. These hurdles mainly revolve around data privacy and the management of diverse media formats. Overcoming these obstacles is key to achieving efficiency and security in media production settings.
Managing Diverse Formats
Managing media formats is another significant challenge. Media files vary widely, requiring software that can handle this diversity. This ensures consistent quality and reliability in annotated content. Commercial solutions like Keylabs provide advanced, automated support for various formats.
Considerations for overcoming these challenges include:
- Setting up transparent industry standards for secure media management
- Ensuring consistent quality across different media formats
- Finding the right balance between manual and automated annotation to optimize cost and accuracy
Aspect | Challenges | Solutions |
---|---|---|
Data Privacy | Handling sensitive content without proper consent | Use AI to blur sensitive images; establish transparent standards |
Diverse Formats | Supporting various media types and codecs | Employ robust software tools like Keylabs |
Evaluating AI Annotation Tools
The world of AI tools evaluation is rapidly changing. This is due to the growing need for precise and efficient annotation across industries like autonomous vehicles, healthcare, and retail. Choosing the right annotation tools involves several key factors. These include accuracy, speed, scalability, and integration capabilities.
Criteria for Selection
When picking annotation tools, several important factors must be considered:
- Accuracy: Tools need to show high precision compared to the actual data to maintain data quality.
- Speed: How fast a tool can annotate data is vital for meeting deadlines.
- Scalability: Solutions should grow with larger datasets without losing performance.
- Integration: Tools should integrate smoothly with current systems and other apps for a seamless workflow.
- Quality Assurance: The ability to keep annotation quality high through advanced features is essential.
Future Trends in Media Content Annotation
The future of media content annotation is bright, with 2024 and beyond promising significant advancements. Machine learning is at the forefront, improving AI tools' accuracy and efficiency. These tools are becoming more reliable and capable, thanks to these innovations.
Advancements in Machine Learning
Machine learning is revolutionizing media content annotation. Techniques like deep learning, including CNNs and GANs, enhance image and video annotation. They identify complex patterns, improving accuracy. Real-time annotation is also becoming key, vital for autonomous driving and surveillance.
Self-learning annotation tools are gaining traction. They refine their accuracy with user feedback, reducing manual effort. Transfer learning further speeds up training on new tasks, benefiting smaller projects. This efficiency is a game-changer for many industries.
Predictions for Industry Growth
Unstructured data and Large Language Models (LLMs) are driving this growth. Generative AI is also making data labeling more efficient. Sectors like autonomous vehicles and healthcare will see significant benefits from these advancements.
Automation is transforming annotation workflows, promising scalability and consistency. Outsourcing annotation services offers flexibility and cost savings. This approach accelerates model training and boosts accuracy.
As AI technologies evolve, they will shape the future of media annotation. Businesses are focusing on innovation and scalability, opening up new opportunities.
FAQ
What is media content annotation?
Media content annotation involves adding metadata to videos and images. This metadata describes the content or context. It makes searching and retrieving content easier, streamlines editing and archiving, and boosts workflow efficiency.
How does AI-driven image and video annotation benefit media production?
AI-driven annotation automates tasks like tagging and categorizing visual data. This improves content quality and viewer engagement through personalized recommendations. It also enhances content discoverability, reducing manual effort and saving time and money.
What types of AI technologies are used in media annotation?
Media annotation uses machine learning, computer vision, and deep learning. These technologies automatically recognize and tag objects, scenes, and activities. They offer high accuracy and efficiency in categorizing content.
Can AI-driven annotation improve accuracy beyond manual methods?
Yes, AI-driven annotation significantly boosts accuracy. AI systems learn and improve from new data, leading to more precise annotations. This refines search algorithms and content recommendations over time.
What are the common use cases for media content annotation?
Common use cases include social media management, advertising and marketing, and sports analytics. These areas benefit from accurate and efficient content sorting and analysis.
How does AI-powered annotation compare to manual annotation?
AI-powered annotation is more efficient and cost-effective than manual methods. While initial AI technology costs are high, long-term savings from reduced labor and faster content turnaround are significant.
What are the best practices for implementing AI annotation in media production?
Start with clear objectives and determine specific needs. Choose AI tools that meet these needs, train staff properly, and integrate AI gradually. This ensures a smooth transition from existing systems.
What challenges might media content annotation face?
Challenges include data privacy and managing diverse media formats. Balancing AI enhancement and user privacy is essential. Developing systems that handle various media files ensures consistent quality.
How should media companies evaluate AI annotation tools?
Evaluate tools based on accuracy, speed, scalability, and integration ease. Choose specialized tools for real-time annotation or multi-format support. This ensures the best fit for operational needs.
What future trends are expected in media content annotation?
Future trends include advancements in machine learning algorithms. These will drive more accurate and capable AI annotations. Industry growth is predicted due to demand for streamlined content management and advanced analytics.
Are there any real-world case studies showing the benefits of AI-driven media annotation?
Yes, numerous case studies highlight efficiency gains, cost savings, and improved audience engagement. These examples emphasize the importance of customizing AI tools and continuously training AI systems for optimal performance.
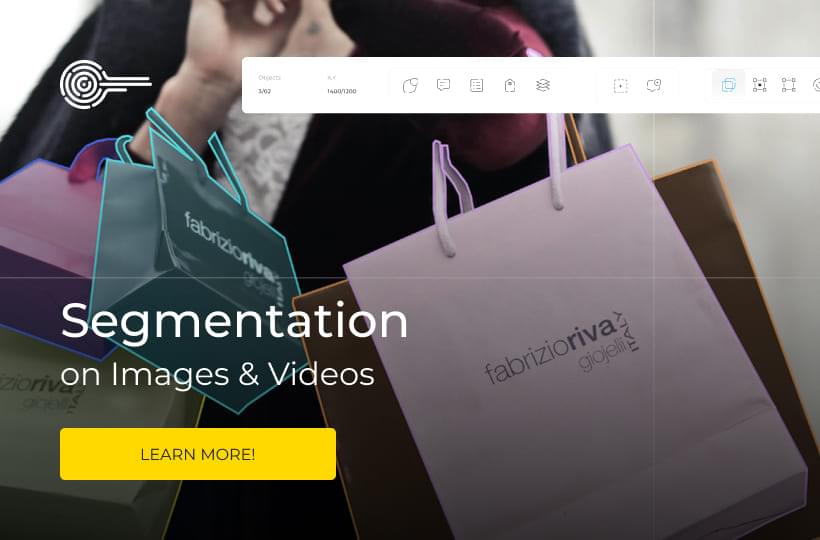