The Ethical Gaze: Examining Bias and Privacy Concerns in AI Image Recognition
AI algorithms designed for facial recognition often show biases, especially against darker-skinned women. These biases can result in error rates as high as 35%, whereas lighter-skinned men's errors stay under 1%. This reveals a critical need for ethical considerations in artificial intelligence development. Whilst our technological progress in AI image recognition is vast, it brings along substantial ethical dilemmas.
The foundation of many AI systems is datasets mainly featuring male, white, and Western individuals. This setup can skew results and potentially foster discrimination towards minorities and other marginalized groups. In tackling this, the framework for AI governance must be built on inclusivity and transparency. Such an approach is essential to prevent these biases from further entrenching societal inequalities. Development in AI needs to be both responsible and inclusive, serving the entire global population without amplifying existing forms of discrimination.
Key Takeaways
- AI image recognition error rates are significantly higher for darker-skinned women compared to lighter-skinned men.
- Predominant training datasets skew towards male, white, and Western populations, leading to biased AI outcomes.
- Lack of diversity in AI personnel and inclusive datasets results in a narrow perspective embedded in AI systems.
- Opaque AI decision-making processes, often termed the "black box" problem, hinder transparency and accountability.
- Ethical AI development must incorporate inclusivity, transparency, and fairness to mitigate discriminatory practices.

Introduction to AI Image Recognition
AI image recognition technology is now critical in numerous fields. Its ability to comprehend visual data has revolutionized healthcare, security, retail, and transportation. These systems must be meticulously designed and trained to guard against facial recognition bias, AI transparency concerns, and uphold ethical AI principles.
Understanding AI Image Recognition
Computer vision utilizes deep learning to classify images through AI. It has shown better accuracy than older techniques in various areas. Despite its superiority, it relies heavily on large training datasets and demanding computations. The concern lies in dataset selection. If AI models learn from data that mainly represents certain groups, they might unjustly discriminate. This is particularly problematic in applications like facial recognition.
Impact and Applications
Its uses are broad:
- In healthcare, it aids in detecting conditions like cancer and heart disease, and in analyzing medical images.
- Security systems use it for identification and monitoring, raising ethical AI worries due to potential biases.
- Within retail, it helps with inventory management, fraud prevention, and crafting personalized customer experiences.
- In transportation, it contributes to safety improvements, traffic management, and the evolution of autonomous vehicles.
Despite its clear benefits, AI transparency is crucial. Decision-making by AI must be understandable and fair to meet ethical standards and curb bias. Actions like Adversarial Debiasing are key steps towards creating fairer AI.
Negative outcomes, though, are real. Biased AI can unfairly target people of color in facial recognition, for example. Google's advertising has also deepened economic disparities by favoring certain groups. Overcoming these challenges is essential for more equitable AI that serves everyone.
Establishing strong ethical guidelines is paramount. These ensure the creation of AI systems that are fair and free from bias. With these principles in place, the potential benefits of AI image recognition in diverse fields can be fully realized.
The Rise of AI Bias
AI's growing use brings to light algorithmic bias. This issue affects AI fairness, especially in image recognition. It can come from various origins, influencing AI inclusivity.
Sources of AI Bias
To make AI fair, knowing where algorithmic bias comes from is vital. It arises from several causes:
- Biased Training Datasets: Datasets can mirror society's prejudices, impacting AI.
- Lack of Diversity among Developers: A lack of team diversity limits inclusive thinking, affecting AI development.
- Black Box Algorithms: AI's opaque algorithms hide biases, making them hard to root out.
Types of Bias in Image Recognition
In machine learning fairness, image recognition faces varied biases. Each type poses its own challenges:
- Data Bias: Unrepresentative training data skews AI results, affecting outcomes.
- Algorithmic Bias: The creator's presumptions can embed bias into AI design.
- Label Bias: Mislabeling or biased data labels affect AI's learning, leading to incorrect conclusions.
- Interaction Bias: User-AI interactions can reinforce prejudice, worsening bias.
Implications for Marginalized Groups
Biased AI, like in image recognition, deeply impacts marginalized people. It can reinforce inequality, leading to discriminatory outcomes. For instance:
"In May 2023, Geoffrey Hinton left Google to raise awareness about the risks associated with AI, particularly related to the potential dangers posed by AI chatbots being exploited by 'bad actors' and the existential risk of AI surpassing human intelligence."
This bias affects fields such as law, hiring, and health, often targeting the vulnerable. To combat this, embracing machine learning fairness and inclusive AI is vital.
Case Studies: Facial Recognition Failures
Law enforcement globally has incorporated facial recognition, aiming at improving crime control. Nevertheless, these tools often confront major AI ethical concerns. For instance, a July 2018 ACLU test found that Amazon Rekognition misidentified 28 US Congress members as criminals. It was noted that close to 40 percent of these erroneous identifications were of people of color, though they make up only 20 percent of Congress.
In January 2019, MIT's study brought to light racial and gender disparities in facial recognition. It showed Amazon Rekognition struggled more to pinpoint the gender of women and darker faces. Facial recognition bias is thus a pressing issue in these systems.
IBM later aimed to reduce mistakes in its facial recognition by a considerable margin through the "Diversity in Faces" dataset. Even with this progress, concerns over privacy arose given that the dataset was obtained from Flickr without user permission. This incident has underscored the importance of addressing AI ethical concerns and ensuring strong data protection norms.
The examination also highlighted the regulatory obstacles in the USA, UK, and EU. These areas are striving to navigate through the complexities surrounding these tech tools. They are especially concentrating on ensuring fairness and protecting human rights via algorithmic justice and DPIAs.
Such persistent issues call for enhanced transparency, regulation, and continuous assessment in deploying facial recognition. Law enforcement entities must tackle these issues to prevent privacy violations, false charges, and discriminatory acts. Striving for equitable AI practices is paramount.
Bias in AI Training Data
Training data biases are key origins of discrimination in AI systems, potentially causing unfairness. To combat this, responsible AI requires focusing on inclusivity within datasets. It also demands the use of robust strategies to prevent bias in AI.
The Role of Training Data in AI Ethics
The composition of training data significantly influences the ethical nature of AI. Datasets that are overwhelmingly male, white, and from Western origins can lead to models that do not serve all people equally. This was illustrated in Amazon's controversial hiring algorithm, which favored male job applicants over females. IBM's Watson AI also faced scrutiny for showing racial bias against black defendants in criminal recidivism predictions.
Examples of Biased Datasets
The Facebook-Cambridge Analytica scandal, which affected over 87 million users, drew attention to major privacy breaches caused by biased AI systems. Similarly, the 2016 Uber data breach, involving information from 57 million users and drivers, emphasized the critical need for heightened data protection.
For responsible AI, a multi-faceted approach is imperative. It entails the formation of AI governance frameworks within organizations, the cultivation of ethical cultures, and the championing of diversity in both workforces and data inputs. This strategy also involves clearly defining fairness, curating well-balanced datasets, and regularly auditing AI systems for bias. Such measures are vital to ensuring that AI is fair and accessible to everyone.
Ethics in AI Image Recognition
The fast-paced advancement of AI in recognizing images demands strict ethical norms. These guidelines ensure that AI growth respects societal morals. Ethical AI is founded on inclusivity, accountability, and transparency. They cultivate trust and equality within AI systems while lessening the hazards of bias and privacy breaches.
Principles of Ethical AI
Upholding ethical standards is keystroke to developing AI that is both responsible and inclusive. It stresses the:
- Inclusivity: Diverse data usage is essential to AI equity and the reduction of biases.
- Accountability: There must be ways to make AI developers and users answer for AI system outcomes.
- Transparency: Openly sharing AI decision processes is crucial for building public trust.
Challenges in Implementing Ethical Standards
Progressing toward ethical AI confronts significant hurdles. Harmonizing precision with justness can be intricate, as prioritizing one might weaken the other. Moreover, the lack of transparency in AI processes adds complexity to comprehending and clarifying AI reasoning. Abiding by laws such as the GDPR and the CCPA adds another layer of complexity.
Real World Examples
Instances from daily life highlight the potential and drawbacks of AI image recognition:
- Healthcare: AI's application in medical imaging has enhanced tumor detection beyond human capabilities. Yet, the fairness of these systems depends on being trained with diverse and comprehensive datasets.
- Autonomous Vehicles: Image recognition in autonomous vehicles is crucial for their navigation and safety features. Access to varied and extensive data on road signs is essential for their optimal function.
- Industrial Drones: In industrial domains, drones can pinpoint defects in critical infrastructures with exceptional precision, elevating safety and productivity.
- Deepfake Technology: This technology, although providing new avenues for creativity, brings to light significant ethical dilemmas by enabling the spread of false or harmful content.
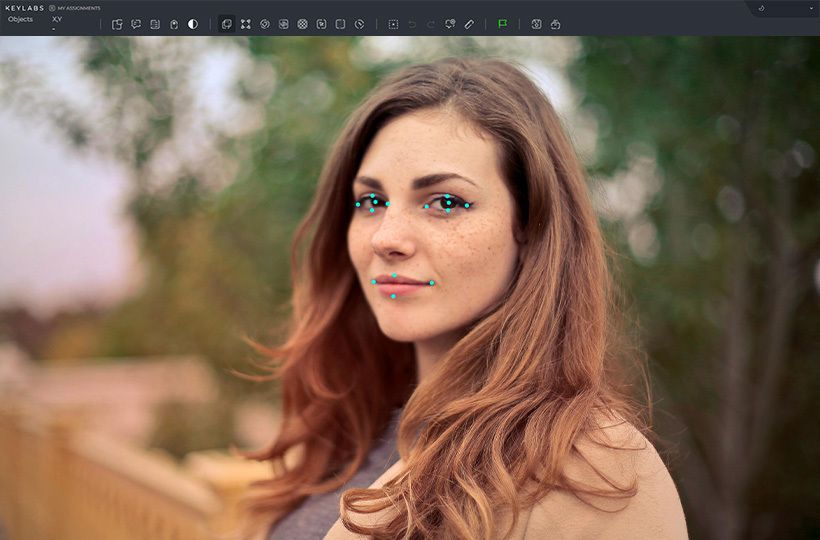
Transparency in AI Systems
AI systems, now more than ever, are affecting various parts of our lives. This makes the need for AI transparency critical. Transparency is key to solving the "black box" problem, allowing for explainable AI. It also fosters responsible AI development.
The "Black Box" Problem
In AI, the "black box" issue means we can't see how decisions are made. This leads to AI making choices without us understanding why. The hidden processes can lead to issues, such as in autonomous cars. They are not as good at seeing children or darker-skinned people.
Facial recognition technologies are another example. They make five times more mistakes with identifying black people. This highlights the need to fix the black box problem.
Importance of Explainability
Being able to explain AI is crucial for its approval and for fair results. A study shows 75% of firms fear losing customers due to this. Clear AI systems help us check decisions better and improve fairness. They meet these three key requirements for transparent AI:
- Explainability
- Interpretability
- Accountability
One way to better AI transparency is through laws like New York City’s Local Law 114. It requires employers to check their AI tools for bias. Such laws are key to making AI ethically sound. UNESCO's establishment of global AI ethics standards in 2021 is also a big step towards more transparency.
Aspect | Statistic | Highlight |
---|---|---|
Autonomous Driving Systems | 20% worse at recognizing children than adults | Children often under-recognized |
Facial Recognition | 5 times more false detections for black people | Significant racial bias |
Customer Retention | 75% believe lack of transparency increases churn | Business risk with opaque AI |
Finally, real AI transparency means being open about how algorithms work and their impact. It is a joint effort between businesses and lawmakers. Together, they can achieve explainable AI. This is essential for responsible AI development, leading us towards a more just and open future.
Evaluating Fairness in AI
Ensuring AI systems are fair demands thorough evaluation. This includes using machine learning fairness metrics and AI validation strategies. Through these methods, one can achieve algorithmic accountability. This helps to reduce biases, thus enhancing the fairness of AI outcomes.
Metrics for Fairness
Measuring the fairness of an AI system involves several key metrics. These include demographic parity, equal opportunity, and disparate impact ratio. They aim to ensure the AI system treats all demographic groups equally. Furthermore, some metrics focus on individual fairness. They analyze how consistently the AI makes decisions for similar people.
Type of Bias | Definition | Example Scenario |
---|---|---|
Data Bias | Bias inherent in the training data | A dataset that overrepresents one demographic group |
Algorithmic Bias | Bias introduced by the AI model itself | Facial recognition systems with higher error rates for certain races |
Label Bias | Bias due to incorrect or skewed labels | Labels that reflect societal stereotypes |
Interaction Bias | Bias from the interaction between AI and users | Users' feedback that reinforces existing biases |
Testing and Validation Methods
AI validation relies on various techniques to check and fix biases. The process spans pre-, in-, and post-processing. Pre-processing tackles data preparation, seeking to eliminate biases. In-processing adjusts the learning algorithm itself, introducing fairness-aware learning methods. Finally, post-processing employs strategies such as calibration and reweighting, ensuring fairness after training.
Testing for fairness also demands algorithmic transparency, emphasizing the importance of explainable AI (XAI). XAI elucidates decision-making processes, promoting algorithmic accountability. Through a holistic approach, we can establish a solid framework for AI fairness evaluation. This is critical in fields like justice or employment, which have far-reaching implications.
Regulating AI: Policies and Guidelines
Artificial intelligence's rapid evolution highlights the crucial need for broad AI governance. The existing rules on ethical AI and privacy often fall short. To fill these gaps, we must create strong policies. Such guidelines are essential for the responsible development of AI, especially in critical areas like image recognition.
Existing Regulations
The Council of Europe's AI treaty, with 46 country members, and the OECD's nonbinding AI principles from 2019 mark the start of global AI policy. These initiatives show mixed effectiveness. However, the AI Act launched by the European Union in 2021 is highly regarded. It scored 5/5 for its comprehensive regulation of high-risk AI uses. Technical standards endorsed by international entities also play a crucial role, achieving a 4/5 rating for their part in ensuring AI law adherence.
Need for New Policies
Although some guidelines like the UN's 2021 AI ethics framework exist, their impact is limited (rated 2/5). This points to an urgent need for new, enforceable policies. Just look at cases like Amazon's biased hiring tool and the skewed sentencing in the U.S. These instances highlight the critical necessity for better regulations. Already, some states are tackling issues like deepfakes with focused laws on election integrity and media truthfulness.
Key aims of future policies should include a focus on ethics. This means prioritizing fairness, openness, and public oversight to prevent AI misuse. By evaluating current global regulations, we can develop new AI policies to meet ethical challenges. Doing so will ensure AI's contribution to a fair and inclusive society.
Recent breakthroughs in 2024 coming from the US and the EU, introducing more comprehensive frameworks for AI training and data governance may help. The AI Act in particular is expected to significantly shift the landscape in the European Union.
FAQ
What are the main ethical concerns in AI image recognition?
The core ethical issues in AI image recognition center on biased training data, opaque AI decision-making, and privacy invasions. These issues may lead to unfair actions, particularly against those already marginalized by society.
How does AI bias affect marginalized communities?
AI bias mirrors and exacerbates social prejudices, causing errors and discrimination against marginalized groups. For example, it can lead to wrongful accusations or biased access to opportunities and services.
What causes bias in AI image recognition systems?
Biases in AI systems often stem from skewed training data, a lack of diversity in development teams, and the mysterious workings of AI algorithms. These factors contribute to the development of discriminatory technologies.
Why is transparency important in AI systems?
Transparency within AI is vital for enabling external review and promoting fairness. It's key to validating AI decisions, promoting equal outcomes, and fostering trust in these advancing technologies.
What is the "black box" problem in AI?
The "black box" issue highlights AI algorithms' inscrutable nature, which conceals their decision processes. Such opacity not only masks biases but also hinders accountability and trust.
What are the principles of ethical AI?
Guiding ethical AI are values like inclusivity, accountability, transparency, and fairness. To uphold these principles, developers must navigate the challenges of ensuring accuracy and equity, including addressing the "black box" problem.
How can AI training data be made more ethical?
Creating more ethical AI data involves using varied, unbiased datasets and adhering to responsible development practices. It also necessitates regular auditing and updating to check for and rectify biases.
What are the current regulatory challenges in AI governance?
Present hurdles in AI governance comprise insufficient regulation over privacy, consent, and fairness. Addressing these issues is paramount for preventing misuse and ensuring AI's ethical evolution through public oversight.
Why is it important to have new policies for AI technologies?
Enacting fresh AI policies is critical to tackling its intricate ethical dilemmas, such as fairness and accountability. These policies aim to steer AI to benefit all, rather than entrenching societal disparities.
How do you measure fairness in AI?
Assessing AI fairness depends on using rigorous metrics and testing protocols to identify and rectify biases. This calls for ongoing validation and transparency in algorithmic decision-making to promote fair treatment across all groups.
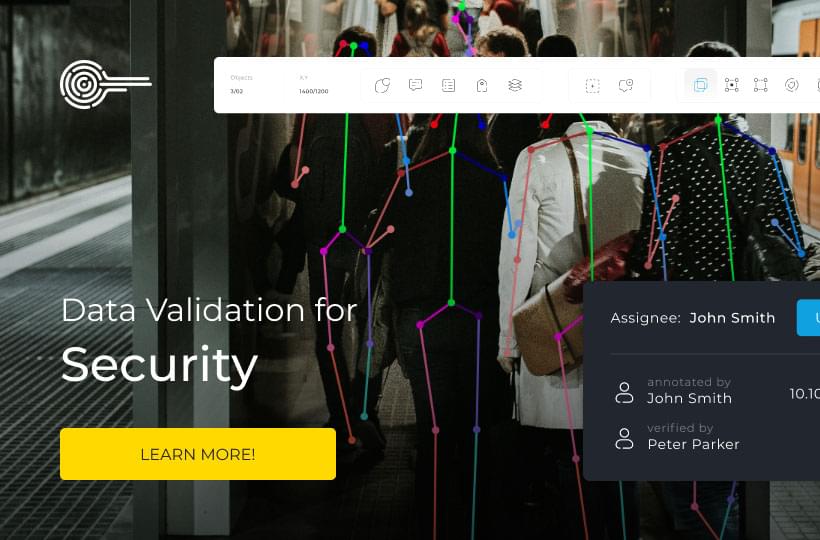