The Recognition Giants: Comparing Leading AI Image Recognition Platforms
AlphaCode from OpenAI has proven to be a fierce competitor. It has surpassed 45% of human coders in contests. This achievement highlights AI's significant role across different sectors, especially in visual data analysis. The emergence of powerful tools like GPT-4o has increased the importance of selecting the best AI image recognition platforms.
Recently, Macy Kuang, a top VR/AR Game Engineer and Google Developer Expert, raised eyebrows in the tech world. She did so by comparing the image recognition prowess of ChatGPT-4 and Gemini Pro within her professional circles. Her actions have sparked a deep dive into the capabilities and applications of these two renowned tools.
Key Takeaways
- Selecting the right AI image recognition tools is vital for effective data analysis.
- GPT-4 highlights the significance of choosing appropriate AI models for specific objectives.
- AI technology is constantly advancing and reshaping how companies handle visual data.
- Macy Kuang's insight offers a detailed look at the usefulness of ChatGPT-4 and Gemini Pro for image recognition purposes.
- Knowing the distinct advantages of each tool is essential for making wise choices based on business requirements.

Overview of AI Image Recognition Technology
AI image recognition involves complex tools. These tools analyze pictures to identify logos, objects, or scenes. Image processing algorithms are key. They help handle the huge number of images we see daily. Neural networks are used here. They allow deep learning to find and categorize important information.
Sagacify, Google, Amazon, and others have pushed or advanced image recognition. They use leading artificial intelligence in image analysis. This makes the systems more precise and quicker. For example, the Mask RCNN algorithm became the top real-time object finder in 2017. It needed 330ms for each frame on the MS COCO test. By 2021, the YOLOR algorithm made a major breakthrough. It brought the time down to 12ms on the same test, beating YOLOv4 and YOLOv3.
In 2022, the YOLOv7 algorithm was a big leap forward, surpassing YOLOR in both speed and accuracy. By 2023, YOLOv8 set a new standard for spotting objects quickly. It became the leader in image segmentation. The announcement of YOLOv10 at the beginning of 2024 shows the continuous drive for better tech.
Deep learning really changed the game in image recognition. Unlike old methods, it doesn't need a lot of data for training. Normally, 80-90% of the image dataset is for teaching the model. The rest is used to check the model's performance.
Artificial intelligence in image analysis is more than just tech improvements. It also boosts the market. In 2024, it's worth $2.55 billion. Experts predict it'll grow to $4.44 billion by 2029. At its heart are neural networks and image processing algorithms. The future of AI image recognition looks even more promising. It aims for better accuracy, efficiency, and use in different industries.
Importance of AI in Image Recognition for Businesses
In today's data-driven world, businesses are turning to AI image recognition. This step is vital to face the abundance of image data efficiently. With the ability to learn from labeled datasets, machines understand visual patterns. They then automate tasks, notably improving productivity and efficiency.
Recognized leaders such as Sagacify offer advanced solutions. These go beyond recognizing logos. Their systems provide deep insights into a brand's marketing reach. This is crucial for strategic decision-making and fully utilizing image data. AI marketing tools allow companies to analyze vast visual content. This helps them better comprehend their customers' behaviors and preferences.
AI's value extends beyond managing data. It plays a key role in protecting a brand's image. By ensuring all visual content complies with brand guidelines, it stops unauthorized logo and imagery use. This action safeguards the brand's reputation. Moreover, AI's ability to accurately identify objects across various environments boosts business security.
The AI image recognition market is poised for significant growth. Valued at $2.55 billion in 2024, it's expected to reach $4.44 billion by 2029. This forecasted growth of 11.76% emphasizes the increasing reliance on AI across industries for precise analysis and operational efficiency.
AI image recognition has a wide range of uses, from healthcare to public safety. For businesses, these tools open doors to new opportunities. They also help strengthen connections with customers. This leads to innovation and a competitive edge.
Key Benefits | Description |
---|---|
Efficiency | Automates manual, time-consuming tasks for faster analysis. |
Accuracy | Eliminates human subjectivity, reduces errors, and enhances precision. |
Personalization | Enables tailored content and recommendations based on visual data. |
Brand Protection | Ensures consistent use of brand imagery and detects unauthorized use. |
Market Insights | Provides actionable insights for strategic marketing decisions. |
Implementing AI in image recognition helps companies stay adaptable. It does so in a fast-changing digital environment. By using AI, firms can enhance customer engagement, protect their brand, and improve their market strategies.
Key Features and Capabilities of Leading Platforms
Top AI image recognition platforms excel in advanced features, catering to varied needs. They utilize cutting-edge models for object detection and image classification. This ensures they perform with both high efficiency and a wide range of applications.
Object Detection and Image Classification
These platforms are powered by object detection models and image classification techniques at the forefront of technology. The Mask RCNN, celebrated for its swift inference in 2017, was succeeded by the even quicker YOLOR in 2021. Subsequently, YOLOv7, YOLOv8, and YOLOV9 now pushing into v10, all expanded the boundaries of rapid, accurate detection. Their updates showcase the ongoing evolution and enhanced performance in this sector.
Deep Learning Architectures and Neural Networks
Deep learning architectures and neural networks define the superiority of these solutions. They learn from extensive datasets, needing less data to excel compared to older methods. In 2023, the Segment Anything approach dominated image segmentation. The introduction of YOLOv9 further diversified the capabilities by bringing in new architecture for training object detection models more effectively.
Integration and Customization Options
One standout feature of these platforms is their adaptable integration and customization options. They allow tailored use to meet a business's precise tech needs. This is further supported by access to advanced libraries and commercial models, streamlining custom developments. Such support minimizes the time needed to implement these powerful tools.
Algorithm | Inference Time | Release Year | Remarks |
---|---|---|---|
Mask RCNN | 330ms | 2017 | Fastest real-time object detector on MS COCO benchmark |
YOLOR | 12ms | 2021 | Surpassed YOLOv4 and YOLOv3 in efficiency |
YOLOv7 | N/A | 2022 | Exceeded YOLOR in speed and accuracy |
YOLOv8 | N/A | 2023 | State-of-the-art performance for real-time object detection |
YOLOv9 | N/A | 2024 | New architecture for efficient model training |
Segment Anything | N/A | 2023 | State-of-the-art image segmentation |
Amazon Rekognition: A Detailed Analysis
Amazon Rekognition is a standout player in the realm of AI-driven image recognition. It boasts deep functionality and a smooth fit within the AWS infrastructure. This platform leverages cutting-edge machine learning to provide a robust set of features. As such, it has become a premier choice in the commercial image recognition space.
Core Features and Strengths
At the heart of Amazon Rekognition are its numerous and varied capabilities. It combines object and scene detection with content moderation and the ability to identify celebrities. With its facial and object recognition, it can pinpoint emotions, approximate age ranges, and even recognize facial hair in media.
In addition, it excels in identifying textual content in various orientations. This is vital for tasks like reading license plates or processing documents.
Use Cases and Applications
Amazon Rekognition finds broad application across different industries, making it a Swiss Army knife for varied business needs. One example is Pinterest, employing its content moderation feature to uphold community guidelines for image uploads. PBS benefits from its object detection abilities to enhance its media workflows.
Companies such as SDG&E use Amazon Rekognition to spot damaged transformers in drone footage. This application boosts operational efficiency and safety, showcasing the product's wide versatility.
Pricing and Accessibility
What makes Amazon Rekognition a viable option for businesses of all sizes is its competitive and scalable pricing. This model is particularly attractive for those already integrated into AWS environments. With a pay-as-you-go scheme, businesses avoid sizable initial costs. They also enjoy the flexibility of paying based on their actual usage.
Feature | Use Case | Benefit |
---|---|---|
Object and Scene Detection | Content Categorization | Automates organization of large datasets |
Facial Analysis | Security Surveillance | Improves accuracy and reliability |
Text Detection (OCR) | Logistics | Streamlines workflows and increases efficiency |
Video Analytics | Real-Time Monitoring | Enhances media and public safety operations |
Google Cloud Vision API: Exploring Its Functionality
The Google Cloud Vision API is a beacon of excellence within the Google Cloud Platform. It stands out with its impeccable image recognition capabilities. This is made possible by the essential API integration. This allows developers to enrich their applications with the power of image analysis effortlessly. The API leverages advanced machine learning techniques to comprehend images in various formats, such as GIF, BMP, and WebP.
As for bulk image processing, the Google Cloud Vision API is an excellent choice. It can handle multiple images within one request. However, it's important to note a size restriction of 8MB per image. Videos pose a bit of a challenge, as their analysis requires frame-by-frame manual extraction before use in either the Google Cloud Vision or Amazon Rekognition. Still, its flexibility and ease of use make it ideal for diverse applications requiring image analysis.
The throughput and accuracy of this API are worth praise. It can identify up to 125 labels within an image, with a remarkable 93.9% relevance. This showcases its superiority over similar services like Amazon Rekognition. Moreover, the API smoothly interacts with applications through its JSON output. This ensures easy data processing across different platforms.
To shed light on key aspects, let's compare it:
Feature | Google Cloud Vision API | Amazon Rekognition |
---|---|---|
Image Formats Supported | GIF, BMP, WebP, Raw, Ico | JPG, PNG |
Batch Processing | Supported (8MB limit) | Not Supported |
Label Detection | 125 Labels (93.9% relevance) | 111 Labels (87.3% relevance) |
Initial Free Units | 1,000 units/month | Not Available |
The Google Cloud Vision API goes beyond basic services by smoothly joining forces with other Google Cloud features. It offers a competitive starting price of only $0.10 per minute for select models. Nevertheless, the expenses could rise significantly for users dealing with large-scale image datasets. This is due to the API's usage-based pricing model.
IBM Image Detection: Customization and Flexibility
IBM Image Detection stands out for its ability to be shaped to fit a wide range of industry needs. It offers tailored solutions through custom image recognition. This lets companies align the technology with their specific goals and requirements, making it highly versatile for diverse sectors.
Advanced Analytical Functions
Deep within IBM Image Detection lies a set of advanced tools for image analysis. These tools use deep learning and neural networks for sophisticated image processing. With more than 80% of data within companies being unstructured, these tools play a key role in extracting valuable insights. They help organizations make better, data-driven decisions faster, boosting operational efficiency.
Industry-Specific Applications
IBM Image Detection serves a broad spectrum of sectors with tailored functionalities. In healthcare, for example, it refines diagnostic processes by providing accurate image recognition. In the e-commerce sphere, it enhances the shopping experience with swift visual searches. Major retailers, like H&M and ASOS, leverage its capabilities to improve their search functions.
Across security, automotive, and fashion sectors, its power to manage and categorize large volumes of visual data shines. This optimization ensures enhanced operational efficiencies in any industry it serves.
Open-Source vs. Commercial Solutions in AI Image Recognition
The decision between open-source and commercial software for image recognition lies in several crucial factors. These include flexibility, cost, support, and innovation. Businesses have to carefully consider the advantages and challenges of each, aligning them with their needs.
Advantages and Disadvantages
Open-source frameworks for AI image recognition are known for their cost-effectiveness and the ability to tailor solutions. This approach benefits projects with limited budgets, given that open-source options are generally free or low in cost. Despite this, they might not offer the same level of reliability and support seen in commercial software, like Amazon Rekognition and Google Cloud Vision API. These paid solutions ensure consistent technical support, robust infrastructure, and the latest technology, albeit at a higher cost.
Community Support and Development
Open-source thrives on the strength of its community-driven development. This dynamic encourages ongoing enhancements and improvements. Users gain from shared insights, regular updates, and a wealth of community resources, leading to accelerated innovation.
Contrarily, commercial software suppliers provide organized professional services and support through models like SaaS. This approach guarantees users access to the newest advancements and tailored help. Furthermore, commercial partners incorporate cutting-edge technologies, including advancements in deep learning, such as the YOLOR, YOLOv7, YOLOv8, and YOLOv9 models. These models greatly enhance the speed and precision of image recognition.
Comparison | Open-Source Frameworks | Commercial Software |
---|---|---|
Cost | Free or low-cost | Subscription-based; higher cost |
Support | Community support | Professional, dedicated support |
Flexibility | High, customizable | Moderate to high, depending on the vendor |
Innovation | Community-driven development | Incorporates latest advancements quickly |
The Role of Deep Learning and Transfer Learning
Deep learning is crucial for the advanced features in AI's image recognition. It surpasses traditional machine learning by utilizing models like convolutional neural networks. These are better at tasks such as image classification and understanding written or spoken language. I will explore how deep learning gets enhanced in performance and how transfer learning is applied innovatively.
Enhancing Performance with Convolutional Neural Networks
Convolutional neural networks are at the heart of deep learning's image analysis. They work in layers to break down images into features automatically. In contrast to the manual work needed in traditional machine learning, CNNs do feature selection on their own. This difference boosts the effectiveness of recognizing images and handling various data types.
Adapting Pre-trained Models for Specific Applications
Deep learning has made significant steps by using transfer learning. This approach lets us adjust existing models for new jobs without starting from scratch. This means less time, power, and data are necessary. By tweaking already extensive models, we can make them excel at focused tasks, enhancing efficiency without the need for more massive data sets or powerful computers.
Traditional Machine Learning | Deep Learning |
---|---|
Requires manual feature selection | Automates feature engineering |
Suited for simpler tasks | Excels in complex tasks |
Easier to interpret | Mathematically complex |
Lower data and computational requirements | Higher data and infrastructure needs |
Contrasting deep learning and traditional methods highlights how CNNS and transfer learning reshape AI. By applying these, image recognition systems see a significant rise in accuracy and speed. This alters the way we understand and process visual information.
AI Image Recognition Platforms Comparison
Comparing AI image recognition platforms like Google Cloud Vision, IBM Image Detection, Amazon Rekognition, shows their diverse capabilities. They vary in features, prices, flexibility, and learning ability.
The Google Cloud Vision API stands out in its ability to accurately identify items, text, and faces. This is achieved through advanced machine learning and neural networks. It's highly regarded for its strong link-up with API, making it perfect for developers.
IBM Image Detection shines in its customization and adaptability. It focuses on different business sectors with its advanced analytical tool evaluation. For those needing specific image recognition solutions, this flexibility is key.
Amazon Rekognition's strong suit is in its precise recognition of faces, objects, and environments. It also boasts top-notch text extraction features. This makes it a go-to AI image recognition option for many commercial uses.
Deep learning methods have notably advanced in flexibility and speed. For example, in 2017, Mask RCNN showcased superior real-time object detection. By 2021, the YOLOR algorithm significantly surpassed these speeds. Even now, the YOLOv7 and YOLOv8 have pushed further, leading up to the YOLOv9 introduction by early 2024/-.
Future Trends in AI Image Recognition Technology
About 80-90% of image sets goes into training AI models. The rest is for testing the performance, accuracy, and usability of the model. These painstaking steps lead to today's advanced image recognition systems - created by carefully annotating thousands of images with platforms like Keylabs.
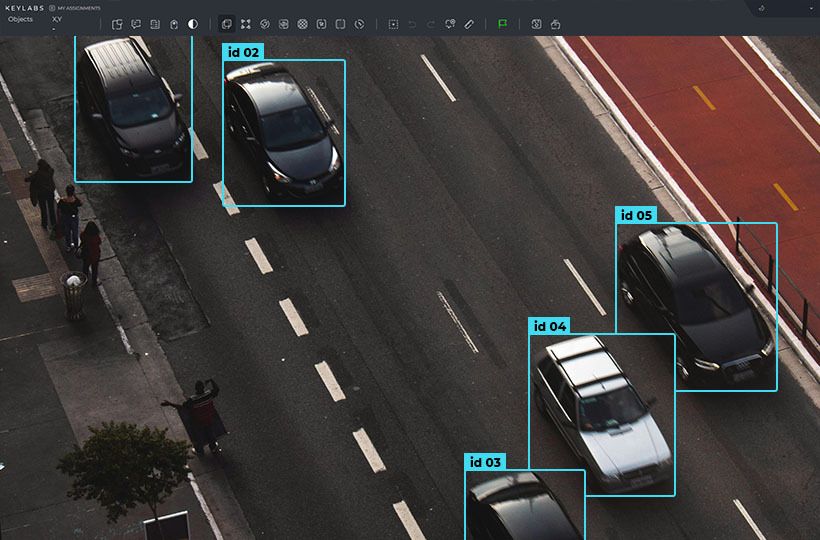
Future trends in AI image recognition will boost security, personalize learning, advance robotic workforces, and monitor environments in real-time. With this, ethical concerns like privacy, misuse risks, and algorithm biases will be significant.
Summary
AI image recognition platforms offer extensive business benefits through cutting-edge technology. Recognized platforms such as Google Cloud Vision and Amazon Rekognition demonstrate AI's potential. They excel in handling visual data and providing essential insights for smarter decision-making. Each platform comes with distinctive features, such as deep learning for spotting objects and specialized analytics for tailored needs. This variety empowers businesses to match their goals with the most suitable AI tool.
When comparing AI platforms, the issue of price is crucial. Advanced solutions, though more expensive, offer high-tech capabilities. On the other hand, Optical Character Recognition (OCR) stands out as an economical option for tasks like document scanning and data entry. Its accuracy in converting text to digital ensures its value and affordability for a wide range of companies.
It's essential to keep pace with the advancing trends in AI and OCR. AI's prowess in analyzing data at scale is fundamental for businesses looking to enhance their efficiencies. Meanwhile, OCR improves accessibility by turning physical text into digital content. Choosing the right technology means considering their offerings, costs, and application scenarios. This thought process is key to fully embracing the advantages of both AI and OCR in image recognition.
FAQ
What are the key features of leading AI image recognition platforms?
Leading AI platforms are equipped with advanced object detection and image classification. They leverage deep learning, including structures like convolutional neural networks. Additionally, they use methods such as transfer learning to deeply understand visual content.
How does Amazon Rekognition assist businesses with image recognition?
Amazon Rekognition offers essential features like facial and item recognition. It also ensures content moderation and user verification. With a competitive price and diverse use cases, it's a prime choice for businesses looking to exploit AI in image recognition.
What makes Google Cloud Vision API a compelling choice for developers?
The Google Cloud Vision API is known for its robust machine learning models. It is easy to integrate for developers. This API excels in tasks like text extraction from images, leveraging Google's expertise in reverse image search.
How does IBM's image detection platform cater to industry-specific needs?
IBM's platform is unmatched in its deep analytics and application flexibility for various industries. They offer solutions that are tailored to meet specific business objectives. This makes it a unique choice for industry-specific image recognition requirements.
How do open-source frameworks compare to commercial solutions in AI image recognition?
While open-source frameworks offer community-driven flexibility, they often lack commercial solutions' infrastructural strength. Tools like Amazon Rekognition and Google Cloud Vision API stand out with their support and cutting-edge technologies.
What role does deep learning play in AI image recognition?
Deep learning, facilitated by convolutional neural networks, greatly improves image recognition system performance. This technology allows for continuous learning, enhancing both accuracy and the system's ability to analyze visual information.
How does transfer learning benefit the development of AI image recognition models?
Transfer learning streamlines the development of new, application-specific models. It bypasses the need for vast datasets or extensive computing power. This method is effective in quickly creating reliable image recognition applications.
What are the future trends in AI image recognition technology?
The future of AI image recognition will witness more sophisticated models and broader application variety. Expect significant progress in accuracy, speed, and capability to gain deeper insights from visual data. These advances will revolutionize how businesses use image recognition technologies.
Why is AI-driven image recognition important for businesses?
AI-powered image recognition is vital for handling vast amounts of image data. It offers valuable insights for understanding marketing reach and safeguarding brand identity. These technologies empower companies to tap into new markets while ensuring their visual content effectively engages their target audience.
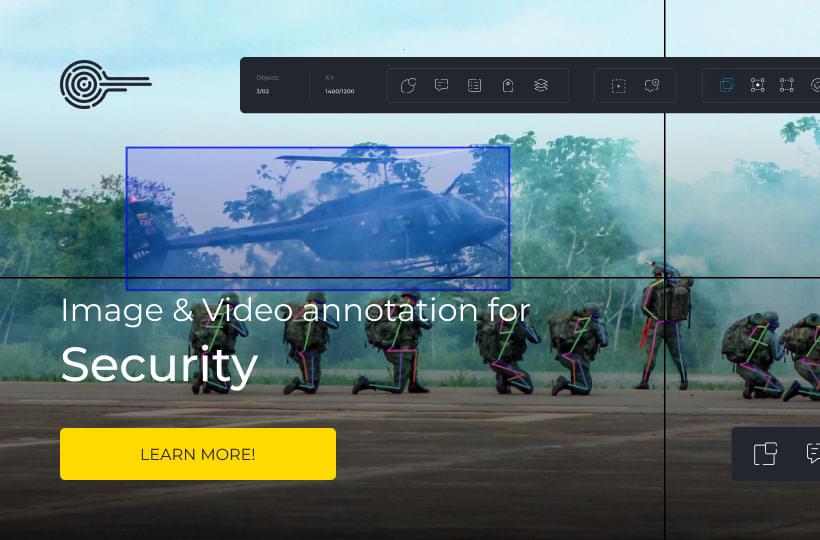