The Role of Data Annotation in Developing Safer Autonomous Vehicles
High-quality annotated data is the foundation of automotive AI data preparation. It ensures AI models can navigate complex real-world scenarios with precision. Techniques like semantic segmentation and bounding boxes are key to improving the perception systems of autonomous vehicles. The ongoing evolution of these annotation techniques is essential to keep up with the increasing complexity of urban environments and variable weather conditions. This is all while striving for safer and more reliable autonomous driving solutions.
Data annotation is vital for creating dependable and safe autonomous driving technology. It gives AI-driven vehicles the context they need to understand their surroundings. This is essential for them to accurately perceive and interpret their environments. The ability to identify and label different elements within sensor data—such as pedestrians, vehicles, and traffic signs—is critical for their operational safety and effectiveness.
Key Takeaways
- Data annotation is critical for autonomous vehicle systems to understand their environment accurately.
- Techniques like semantic segmentation and bounding boxes enhance perception and decision-making.
- High-quality annotated data mitigates risks and boosts the safety and reliability of AI-driven vehicles.
- The complexity of driving scenarios necessitates evolving and improving annotation techniques.
- Accurate perception of various objects, including vehicles, pedestrians, and traffic signs, is achieved through meticulous annotation efforts.

Understanding Autonomous Vehicle Technology
Autonomous vehicles are designed to handle all driving tasks without human input. They use a mix of sensors, cameras, and AI algorithms. The key role of self-driving car data labeling is to ensure these systems work well in different conditions. This technology is changing how we commute and transport goods, making travel safer and more reliable.
What Are Autonomous Vehicles?
Autonomous vehicles, or self-driving cars, can drive on their own without human help. They use advanced tech to make decisions and stay safe by constantly monitoring their surroundings. The use of automotive AI data preparation helps these vehicles understand and respond to various road situations, boosting their performance.
The Levels of Driving Automation
The levels of autonomous driving range from Level 0, where there's no automation, to Level 5, where there's complete automation with no need for human input. These levels are categorized as follows:
- Level 0: No Automation. The driver is fully responsible for all driving tasks.
- Level 1: Driver Assistance. The system can assist with either steering or acceleration, but the driver remains engaged.
- Level 2: Partial Automation. The vehicle can control both steering and acceleration, but human supervision is necessary.
- Level 3: Conditional Automation. The vehicle manages most driving functions, requiring human intervention only when necessary.
- Level 4: High Automation. The vehicle can operate independently in certain conditions without human interference.
- Level 5: Full Automation. The vehicle is capable of performing all driving tasks under any conditions.
By focusing on levels of autonomy, car makers can create systems for different needs, from city driving to highway use. The quality of self-driving car data labeling is critical for training AI, ensuring it can recognize objects and make decisions accurately. This structured approach is seen in the investments of companies like Tesla, Waymo, and Uber.
Preparing large datasets through automotive AI data preparation is key to advancing this field. It's not just about improving algorithms but also about better AI interaction with the driving environment.
The Importance of Data Annotation
In the world of autonomous vehicles, the need for precise and detailed data annotation is immense. Accurate data annotation is the cornerstone upon which machine learning models are constructed. It empowers self-driving cars to understand their environment, make decisions, and navigate safely.
Defining Data Annotation
Data annotation is the act of labeling data to make it comprehensible for machine learning algorithms. This involves tagging images, video frames, and sensor data. For autonomous vehicles, it means identifying pedestrians, vehicles, traffic signals, and lane markings. A deep grasp of vehicle sensor data annotation boosts the vehicle's perception and predictive abilities.
How Data Annotation Supports Machine Learning
Data annotation's role in machine learning for autonomous vehicles is critical. It trains models to identify and categorize various road objects and scenarios. Annotated datasets facilitate efficient object detection and classification, vital for safe driving. They aid in:
- Accurate detection of road status, obstacles, and objects.
- Improved lane and road sign recognition capabilities.
- Enhanced obstacle and pedestrian detection.
Techniques like radar and LiDAR annotation, instance-level and semantic segmentation, and 3D bounding boxes offer detailed insights. These details enable autonomous vehicles to make precise and safe driving decisions. Continuous learning and adaptation through data annotation ensure models remain accurate over time.
Automation is key in accelerating the data annotation process, making it more accessible and speeding up model development. The combination of real-world and synthetic data in simulation environments is essential for robust training datasets. In the rapidly advancing field of autonomous vehicle technology, the continuous enhancement of vehicle sensor data annotation is critical for ensuring the safety and reliability of autonomous driving systems.
Types of Annotation Techniques for Autonomous Vehicles
Creating safe and efficient autonomous vehicles requires various annotation techniques. 2D and 3D bounding boxes, semantic segmentation, and lidar annotation are key. Each plays a unique role in the vehicle's perception system.
2D and 3D Bounding Box Annotations
3D bounding box labeling defines volumes around objects in 3D space. It helps identify and classify objects from different angles. This is vital for detecting vehicles, pedestrians, and cyclists.
Modern autonomous vehicles use over 15-20 external sensors for data capture. Cameras are annotated with bounding boxes, lines, and polygons. This enhances object and obstacle detection, making the process more efficient and cost-effective.
Semantic Segmentation in Data Annotation
Semantic segmentation labels each pixel in an image, showing what it represents. This could be a road, pedestrian, or vehicle. It provides accurate environmental perception, essential for autonomous navigation. High-quality semantic segmentation boosts scene understanding. It aids in quicker and more reliable decision-making.
Lidar Annotation for Object Detection
Lidar sensors create high-resolution 3D point cloud data. Lidar point cloud annotation labels these data points for accurate depth perception. This is critical for navigating complex environments.
Waymo's vehicles record 1.2 GB of sensor data every second. Efficient lidar annotation is vital for real-time analysis.
For a deeper understanding of these methods in autonomous vehicle development, read more about data annotation essentials.
Comparison of Annotation Techniques
Technique | Usage | Advantages | Limitations |
---|---|---|---|
2D Bounding Box | Object Detection in 2D Space | Cost-Effective, Simple | Basic Accuracy |
3D Bounding Box | Object Detection in 3D Space | Multi-Angle Detection | Complex Annotation Process |
Semantic Segmentation | Pixel-Level Labeling | High Environmental Perception | Time-Consuming |
Lidar Annotation | Obstacle Detection and Avoidance | Accurate Depth Perception | High Volume of Data |
Challenges in Data Annotation for Autonomous Vehicles
Data annotation for autonomous vehicles faces several hurdles, mainly due to the complexity of urban settings and the variability in lighting and weather. These challenges affect AV training data labeling and driverless vehicle image annotation significantly. Let's dive into these obstacles and their implications for the development of autonomous vehicles.
Visual Complexity in Urban Environments
Urban areas are filled with activity, making data annotation a daunting task. The numerous objects, from pedestrians to vehicles, require detailed labeling. Techniques like 2D and 3D bounding boxes, semantic segmentation, and contextual annotation are essential. Yet, the visual complexity of urban environments poses a significant challenge for autonomous vehicle image annotation.
To overcome this complexity, annotation providers like Keymakr are vital. They offer customized datasets that capture the essence of urban life. Their work is critical in ensuring the safety of self-driving vehicles as they navigate complex cityscapes.
Variability in Lighting and Weather Conditions
Autonomous vehicles must operate reliably under various lighting and weather conditions. Research indicates that while they perform well in daylight and good weather, their performance drops in low light and poor visibility. Precise image annotation is essential for training algorithms to handle these conditions. Techniques such as interpolation and segmentation are invaluable in accurately labeling data under different lighting and weather scenarios.
Global variations in road conditions also present a challenge. Different regions have unique road markings, traffic rules, and signage. This diversity affects the adaptability of AVs, highlighting the need for robust data annotation. Smart annotation services provide labeled images and videos from around the world, helping AI models adapt to diverse driving etiquettes and infrastructures.
Annotation Challenges | Impact | Solutions |
---|---|---|
Visual Complexity | Mislabeling and Misidentification | Detailed Annotations, Smart Annotation Providers |
Lighting and Weather Variability | Reduced Reliability in Poor Conditions | Robust Annotation Techniques, Diverse Dataset Labeling |
Global Variations | Difficulty in Adapting AI Models | Global Image and Video Annotation |
The accuracy of AV training data labeling and driverless vehicle image annotation is critical for the development and safety of autonomous vehicles. By addressing these challenges, we move closer to achieving safer, more reliable autonomous driving experiences across diverse environments.
The Impact of Quality Annotation on Safety
In the realm of autonomous vehicles, the importance of high-quality vehicle data annotation is immense. The precision of training data directly affects the safety of these advanced machines. It ensures that artificial intelligence models operate with reliability. Exploring the core of quality annotation reveals its critical role in making roads safer.
Accurate and detailed annotations are essential for machine learning models' success. Data annotators carefully label various elements, such as road markings, signs, vehicles, pedestrians, cyclists, and obstacles. This meticulous labeling process enables AI systems to effectively recognize, interpret, and respond to real-world scenarios.
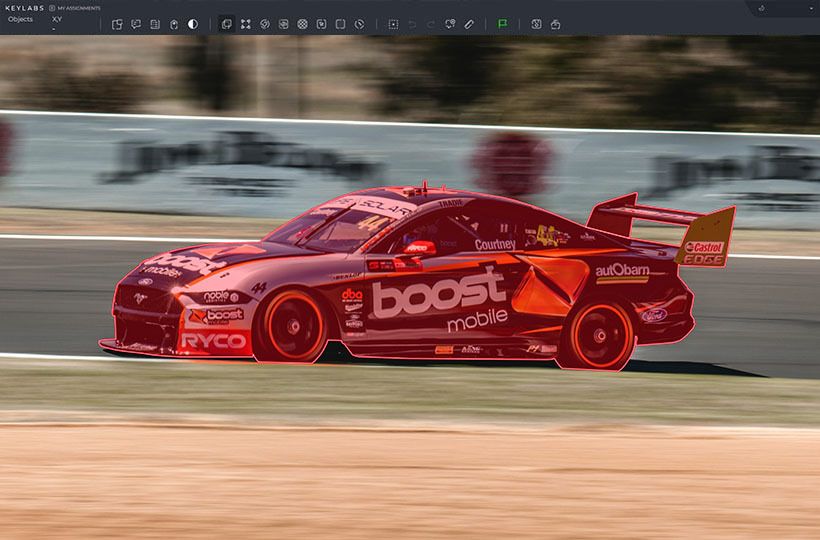
Ensuring Accuracy in Training Data
To ensure autonomous vehicle safety, precision in data annotation is the focus. This involves:
- Engaging skilled annotators with a keen eye for detail.
- Implementing rigorous quality assurance protocols.
- Utilizing advanced AI-equipped annotation tools for consistency.
High-quality vehicle data annotation boosts the accuracy of object detection and classification. It also enhances the prediction of pedestrian and cyclist movements. This contributes to safer self-driving systems.
Consequences of Poor Data Annotation
On the other hand, poor data annotation can have severe consequences. Mislabeling or incomplete annotations can cause AI models to misinterpret their environments. This may lead to dangerous outcomes, including accidents. A failure in autonomous vehicle safety can result in the vehicle's inability to correctly identify and react to obstacles or unexpected objects on the road.
High standards in data annotation are essential. Techniques such as annotating environmental contexts like weather conditions are vital. Using services like 3D cuboids and video annotation is also critical. By prioritizing quality annotation, we advance the development of autonomous vehicles toward safer and more reliable mobility solutions.
Tools and Technologies for Data Annotation
Data annotation is key to improving autonomous driving perception. It ensures the accuracy of machine learning models in self-driving vehicles. To make this process smoother, various popular data annotation tools are used.
Popular Annotation Software Platforms
- Keylabs: Offers precision of up to 99.9% in video annotation projects. It features object interpolation and a magic wand tool that accelerates the annotation process by automatically detecting closed shapes.
These platforms are vital for managing, annotating, and optimizing datasets. They are critical for developing reliable autonomous driving systems.
Machine Learning Support in Annotation Tools
The integration of machine learning (ML) capabilities in annotation tools offers a significant advantage. These features enhance precision and reduce data preparation time
Keylabs employs ML-assisted data annotation for quick, accurate data preparation. It includes hierarchical attributes and multilayer annotations, enriching dataset richness.
Such ML support tools ensure datasets are annotated accurately and enriched with detailed, contextually relevant information. This is essential for building robust autonomous driving models.
By using popular data annotation tools and leveraging ML capabilities, we maintain high data quality standards. This is critical for advancing autonomous driving perception.
The Role of Human Annotators vs. Automation
In the evolving landscape of autonomous vehicles, the intersection between human-powered data annotation and automated annotation technology becomes significant. Both methodologies bring distinct advantages. Their integration is key to achieving high-quality, accurate annotations.
Benefits of Human Annotation
Human annotators play a critical role in ensuring the quality and reliability of data annotation. Their ability to provide contextual understanding and identify nuanced patterns is indispensable in complex tasks:
- High accuracy in delicate tasks such as sentiment analysis and medical diagnosis.
- Contextual understanding in industries requiring nuanced interpretation, such as manufacturing and medicine.
- Identification of subtle patterns, anomalies, and inconsistencies that automated systems might miss.
- Mitigation of bias by ensuring diverse and representative data annotations.
- Enhanced data quality for critical applications like autonomous vehicles and medical imaging.
Given these strengths, integrating human expertise with current technologies provides a holistic approach to data annotation.
The Rise of Automated Annotation Tools
As automated annotation technology advances, its application has grown, meeting the demands for speed and large-scale data handling:
- Speed and consistency in annotating vast datasets.
- Cost-effective solution for repetitive and straightforward annotation tasks.
- Scalability in managing large volumes of data quickly and efficiently.
Yet, relying solely on automated annotation poses challenges, such as missing contextual subtleties that humans grasp easily. This highlights the need for a hybrid approach:
- Combining human precision with automated efficiency to optimize both cost and quality.
- Leveraging automated tools for initial annotations followed by human review for refinement.
This strategic integration ensures that data annotations are not only accurate but also contextual and reliable. The quality of annotated data directly impacts the performance of machine learning models. This emphasizes the importance of a balanced approach. With up to 80% of AI development time devoted to annotating data, according to industry statistics, the hybrid method truly maximizes value.
Ultimately, while automated annotation technology offers remarkable advancements in speed and scale, human-powered data annotation brings irreplaceable depth and accuracy. The combination of both methods is the optimal strategy for data annotation in autonomous vehicle technology.
Future Trends in Autonomous Vehicle Annotation
The field of data annotation is undergoing rapid transformation, with a significant focus on autonomous driving. The integration of AI in data annotation processes is at the forefront, driving towards more efficient and precise technologies. These advancements will lead to safer and more reliable autonomous vehicles in the future.
The Role of Artificial Intelligence in Annotation
The advent of AI in data annotation is transforming how training data is processed and managed. Automation in this area enables the annotation of complex data sets with enhanced precision. By 2024, we expect a significant increase in the demand for AI-driven annotation tools, catering to the complex needs of autonomous vehicle systems.
This move towards automation is influenced by several key factors:
- Improved accuracy in AI models necessitates high-quality annotations.
- The use of synthetic data for training models offers flexibility and scalability.
- Multimodal data annotation, which includes text, images, and videos, is becoming more prevalent.
For a deeper dive into the future of automatic annotation technology, explore our detailed analysis here: The Future of Automatic Annotation Technology.
Evolving Standards and Regulations
As autonomous vehicle technologies evolve, regulatory trends in autonomous driving will be instrumental in shaping data annotation practices. New standards will emerge, guiding the industry towards compliance. These regulations will ensure annotated data meets safety and ethical standards. They will influence:
- The adoption of advanced annotation methods.
- The implementation of stringent quality assurance processes.
- A focus on ethical considerations, including data privacy and bias mitigation.
The establishment of regulatory frameworks will not only boost the capabilities of autonomous vehicles. It will also ensure that innovations in data annotation meet the highest safety standards.
The Path Forward for Safer Autonomous Vehicles
The future of autonomous vehicles relies on teamwork between tech innovators, car makers, and data annotators. By combining our skills, we can improve data annotation methods. This is key for self-driving cars to recognize objects accurately.
Object detection, powered by advanced algorithms like CNNs, lets cars adjust to changing scenes instantly. This is critical for safe driving.
Emphasizing Collaboration in the Annotation Process
Working together in vehicle annotation brings together tech firms, data experts, and human annotators. High-quality datasets, created from various sensors, are the foundation of reliable self-driving tech. This method ensures a wide range of real-world scenarios are captured.
By joining forces, we can tackle biases and boost machine learning. This makes self-driving cars safer and more reliable.
Looking Ahead at Technological Advancements
AI and neural networks will keep pushing the limits of self-driving cars. They will get better at detecting and adapting to objects in real-time. This will make driving in complex situations easier.
Leaders like Tesla and Waymo are already making strides with camera and LiDAR systems. As we move forward, ADAS technologies will change the car industry. They will make roads safer by cutting down on human mistakes, which cause most serious crashes.
By focusing on these advancements and teamwork, we're set for a future where self-driving cars are safer and more efficient.
FAQ
What Are Autonomous Vehicles?
Autonomous vehicles (AVs) are designed to operate without human input. They use sensors, cameras, and AI algorithms. These vehicles range from Level 0, with minimal automation, to Level 5, needing no human intervention.
The Levels of Driving Automation
The levels of driving automation span from Level 0 (no automation) to Level 5 (fully autonomous). These levels show how much an AV can operate on its own. They depend on its technology and the quality of data used to train it.
Defining Data Annotation
Data annotation labels data for machine learning algorithms to understand. For AVs, it means labeling images, video, and sensor data. This training helps algorithms recognize objects and make driving decisions.
How Data Annotation Supports Machine Learning
Data annotation boosts machine learning models' ability to handle dynamic road conditions. It improves vehicle intelligence and safety by training algorithms with detailed data.
2D and 3D Bounding Box Annotations
2D and 3D bounding box annotations detect and recognize objects in different dimensions. They are key for developing strong perception systems in AVs.
Semantic Segmentation in Data Annotation
Semantic segmentation labels each image pixel to show what it represents. This technique improves scene understanding. It helps AVs interpret their environment more accurately.
Lidar Annotation for Object Detection
Lidar annotation labels 3D point clouds for depth perception and object detection. It's vital for navigating complex environments.
Visual Complexity in Urban Environments
Urban environments pose challenges for data annotation due to many overlapping objects. High-level detail in annotations is needed to train models effectively in these settings.
Variability in Lighting and Weather Conditions
Data annotation for AVs must handle variability in lighting and weather. Robust annotation strategies are key to ensure models perform well under any conditions.
Ensuring Accuracy in Training Data
Accurate data annotation is vital for training reliable machine learning models. It ensures algorithms are trained on correct and detailed data, leading to better decision-making.
Consequences of Poor Data Annotation
Poor data annotation can cause AI models to misinterpret, increasing accident risk. High standards in data annotation are critical for safe AV systems.
Machine Learning Support in Annotation Tools
Many annotation tools integrate machine learning to semi-automate the process. This improves accuracy and reduces dataset preparation time.
Benefits of Human Annotation
Human annotators ensure data annotations are of high quality and accuracy. They are essential in nuanced or subjective scenarios where human judgment is critical.
The Rise of Automated Annotation Tools
Automated annotation tools speed up the process and handle simple tasks consistently. The best approach combines human expertise with automated efficiency.
The Role of Artificial Intelligence in Annotation
AI is set to play a larger role in automating data annotation. This advancement will enhance the capabilities of autonomous vehicles.
Evolving Standards and Regulations
New standards and regulations will guide data annotation, ensuring safety and compliance. These will shape the future of autonomous vehicle annotation.
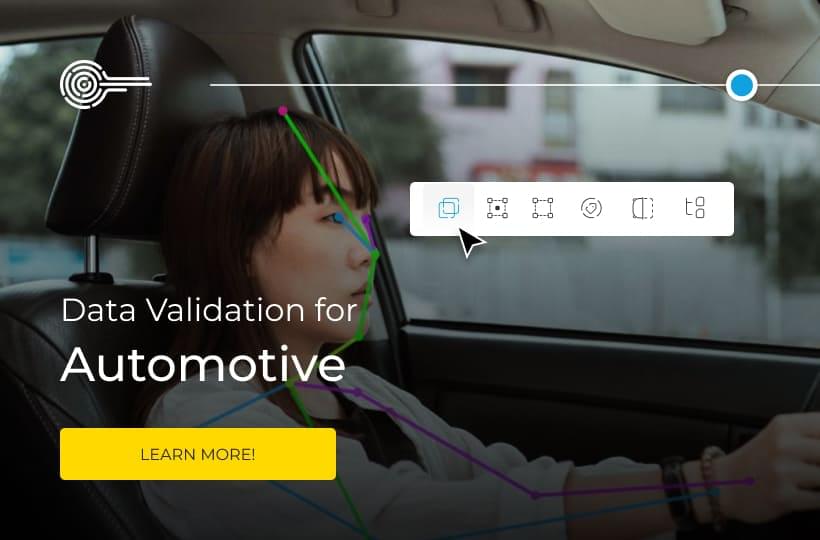