The Top 3 ML Data Labeling Tools for Agriculture
Data annotation is becoming essential in agriculture. It allows for the efficient collection and organization of crop and field data. And the quantities are multiplying thanks to precision agriculture and the increased use of drones. First, data requires labeling with an ML data labeling tool. Next, you can analyze and use it. Finally, you can improve crop yields, reduce costs, and make farming more sustainable.

Ways to Use Data Annotations for ML in Agriculture
Some ways you can use data annotation for machine learning in agriculture include:
- Object classification and detection – Identify and classify different objects. Examples include crops, weeds, and other field features.
- Crop counting and yield prediction – Predict crop yields and count fruits.
- Detection of crop stress and disease – Detect crops' signs of stress or disease. Then, take proactive measures to address these issues.
- Crop growth monitoring – Identify potential problems and decide when to harvest.
- Detection of pests and weeds – Detect issues and take appropriate action.
- Autonomous field navigation – Guide autonomous tractors and other vehicles through fields. Reduce human labor and increase efficiency.
Your model's success correlates to the data's quality and quantity. Therefore, by using accurate annotations, you can train better models.
The 3 Best ML Data Labeling Tools for Agriculture
The three best ML data labeling tools available for agriculture include:
- Bounding boxes
- Polygons
- Polylines
You'll also learn about their features, pros, and cons. Finally, you'll get tips on using them to enhance your data annotation’s accuracy.
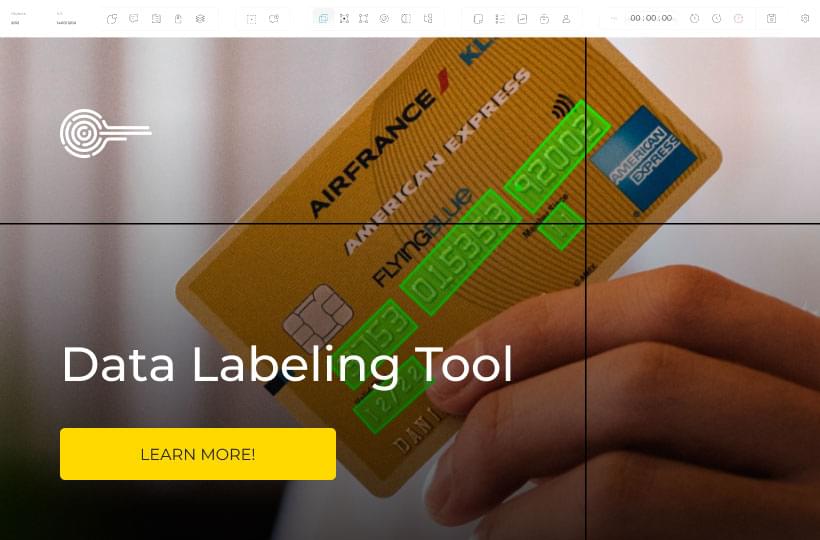
Object Detection Using Bounding Boxes
Bounding boxes are useful for identifying and labeling specific objects. It involves drawing a rectangular box around something. Next, you label it with a class or category. This tool is valuable for detecting objects in agriculture. It identifies and classifies specific crops, fields, or other objects.
Some advantages of this tool include its simplicity and ease of use. Because their shape, you can easily use them to draw and adjust. The annotation can result in faster model training. Bounding boxes are also suitable for object identification and classification.
There's one potential disadvantage of using bounding boxes. They are not always suited for objects with irregular shapes. In this case, a polygon annotation tool may be better.
Before you use bounding boxes in agriculture for object detection, it is vital to ensure two things. First, make the boxes as accurate as possible and use the correct class or category. Review the annotations and check for errors and ensure consistency across images.
Irregular Shapes Detection with Polygon Annotations
The polygon tool can help you identify and label objects with irregular shapes. Examples include irregular-shaped crops, fields, or other things. It can be a better option than the bounding box. Draw forms around objects and tag them with classes or categories.
One advantage of using polygon annotation is that it can fit around irregular shapes. Another advantage is that it's more adaptable than a bounding box. It allows you to capture the form. Creating custom shapes provides for more accurate annotation of these types of things.
But, one disadvantage of using polygon annotation is that it requires more time. You need more precision and care when drawing the shapes. Additionally, polygon annotation tools are more complex than bounding box tools.
To use polygon annotation for irregular shape detection in agriculture. It's vital to ensure the shapes are as accurate as possible and labeled correctly. Checking for errors is also a good practice. Another tip is to use shortcuts and hotkeys.
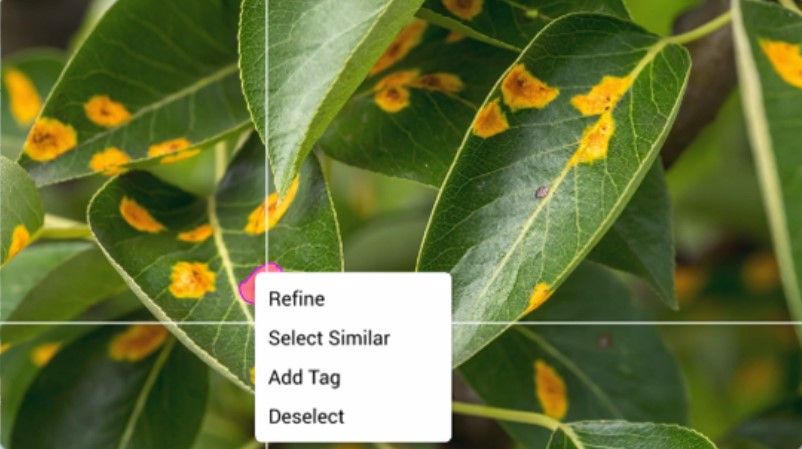
Polyline Annotation for Lanes and Boundaries
Polyline annotation identifies and labels specific lines or paths within an image. This tool helps identify lanes, boundaries, and other linear features. It involves drawing a series of connected points around the line or route. Then label it with a particular class or category. Examples of agriculture include roads, irrigation systems, and limits of fields.
One of the advantages of using polyline annotation is its precision. It allows you to annotate linear features very precisely. This precision can be helpful in cases where the accuracy of the annotation is essential. Examples include identifying specific boundaries or roads in an image. Additionally, polyline annotation can be better at dealing with simple linear features.
A disadvantage is that it is not well-suited for complex shapes. Also, compared to bounding box annotation, it can be more time-consuming. It requires more precision when drawing the lines. In these cases, polygon annotation would be a better choice.
Final Thoughts
Data annotation is an essential part of modern agriculture. Various ML data labeling tools are available to help farmers and researchers label their data. Bounding boxes, polygons, and polylines are powerful techniques for object detection, irregular shape detection, and lane and boundary identification.
If you're looking for a robust data annotation tool, try Keylabs annotation tool. Keylabs tool offers a range of powerful features, such as bounding boxes, polygons, and polylines, to help label your data.