Using cuboids to enhance automotive artificial intelligence
Autonomous vehicles are a rapidly growing industry. AI models are suggested to increase public trust for future generations of autonomous cars as they are considered to be more reliable. Artificial intelligence contributes to the safety of our roads, and in the near future, these vehicles will be able to drive more reliably than humans.
They can't be tired, drunk, or asleep while driving. At the same time it is possible that failures in this area could result in high-profile accidents that may have a negative impact on public belief in the technology in the future.
As a result, annotations of training images and videos are imperative for matching computer vision artificial intelligence models. For the training data to be applied accurately in a three-dimensional world, it must be both complex and spatially oriented. Our service includes Cuboids as part of our suite of labeling techniques.
We dedicate this review to cuboid annotation and will demonstrate to you how it can be for production of more useful training datasets. In addition, we want to show how it can contribute to enhancing the quality of training datasets. And after that, we will see how cuboid annotation can be used in cooperation with professional annotation tools in order to facilitate the development of automotive AI projects.
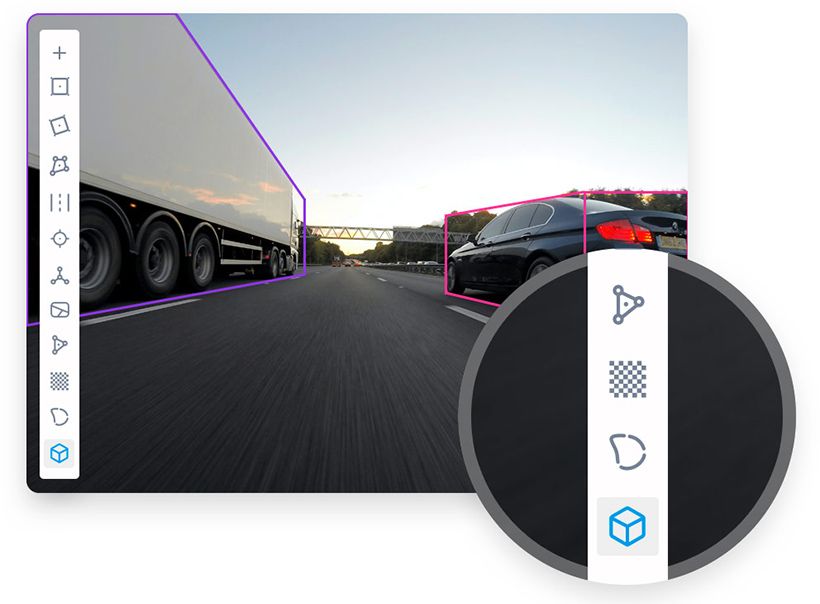
AI applications in automotive industries
It is challenging to generate representative data in a traffic environment with complex movements and interactions. Our team is sure that the usage of cuboids in these cases is a guarantee of higher quality data. For automated travel to be successful, these abilities are essential, enabled by data annotation.
Object detection techniques allow detecting and identifying objects and make them visible to the driver. There can be wide varieties of objects that need to be labeled: cars, buses, people, animals, — all of them should be involved in the image and video annotation process. A computer vision model that is trained on data that is annotated in this manner will be able to recognize objects in the real world.
As part of the training process, each part in an image or video frame must be labeled to create semantic segmentation data for annotators. Using contextual information, AIs can learn more about their environment.
Road signs are automatically detected and responded to by autonomous vehicles. This use case can be supported by annotation tools.
There is a necessity for public road vehicles to be able to distinguish lane markings on the road. Safety is ensured with annotations because they provide parallel shapes of lanes.

Cuboid annotation
In order to train autonomous vehicles, 2D images and 3D frames of space are used as training data. Bounding boxes help us locate people and objects when annotating for AI training. These objects can be given dimensions by using bounding boxes. For instance, an image of a car.
In order to identify the front of the vehicle, annotations will place a bounding box around it. When corners of these boxes are together, we will be able to form an approximate shape that reflects the dimensions of the vehicle. AI models can use this kind of annotation to figure out where they are in three dimensions. Adding this level of detail will make these models safer and perform better.