Why data annotation precision is critical important and how to provide 100% quality?
Data annotation is an essential aspect of machine learning and artificial intelligence. It involves labeling and categorizing data to help machines understand and analyze it accurately. However, the quality of data annotation is crucial. Inaccurate or imprecise data annotation can lead to serious consequences, including incorrect predictions, biased models, and even ethical concerns. Therefore, it is critical to understand the importance of accurate data annotation and how to provide it.
In this article, we will delve into the significance of data annotation precision and explore the consequences of inaccurate data annotation. We will also discuss the different types of data annotation and the quality control and evaluation of annotation results. Finally, we will look at how continuous improvement of annotation processes can help ensure high-quality data annotation. By the end of this article, you will have a better understanding of the importance of data annotation precision and how to provide it.

The Importance Of Accurate Data Annotation
Accurate data annotation is critical for ensuring high-quality data that can improve user experiences and offer relevant recommendations or content. Poor data quality can lead to algorithmic problems, resulting in inaccurate results and poor customer service. Data annotation involves assigning labels to data points to make it easier for computers to interpret, analyze, and respond appropriately.
Business owners understand the importance of reliable and accurate data for better decision-making and confidence in business decisions. Annotated data is crucial for detecting input data accurately, allowing AI and ML models to learn from it effectively. Human-annotated data provides a reliable scalable solution as humans can comprehend the context of the information being presented within its parameters.
Precision in labeling is particularly important for object recognition within contexts such as medical diagnosis or autonomous driving vehicles where accuracy may impact human safety. The challenge is providing consistently precise annotations across large datasets with minimal errors while interpreting specific labeling guidelines.
In conclusion, accurate data annotation plays a vital role in creating good quality training sets required by machine learning systems. It relies heavily on precise labeling by trained staff with an understanding of varying contexts deepening on use cases. By using annotated annotated datasets efficiently we are able to generate highly effective algorithms making them intelligent enough to draw conclusions independently increasing efficiencies reducing errors leading us towards greater reliability proving invaluable especially when considering most modern automated systems rely on these annotated insights giving us confidence they’re performing correctly
Consequences Of Inaccurate Data Annotation
Accurate data annotation is crucial for modern machine learning and artificial intelligence models. Inaccurate annotations can negatively impact the performance of these models, leading to poor decision-making and even outright failures. The consequences of inaccurate data annotation are far-reaching and can affect businesses across industries.
One key consequence of inaccurate data annotation is reduced precision and recall in machine learning algorithms. This means that the model may not be able to accurately classify or predict outcomes, leading to incorrect decisions. Furthermore, poorly annotated data can lead to overfitting, which occurs when a model perfectly fits a particular dataset but performs poorly on new datasets.
Another consequence is that incorrectly labeled data can lead to biased algorithms. For example, if specific demographics are underrepresented in the training dataset, it can result in discriminatory outcomes for those demographics when the algorithm is applied in real-world scenarios.
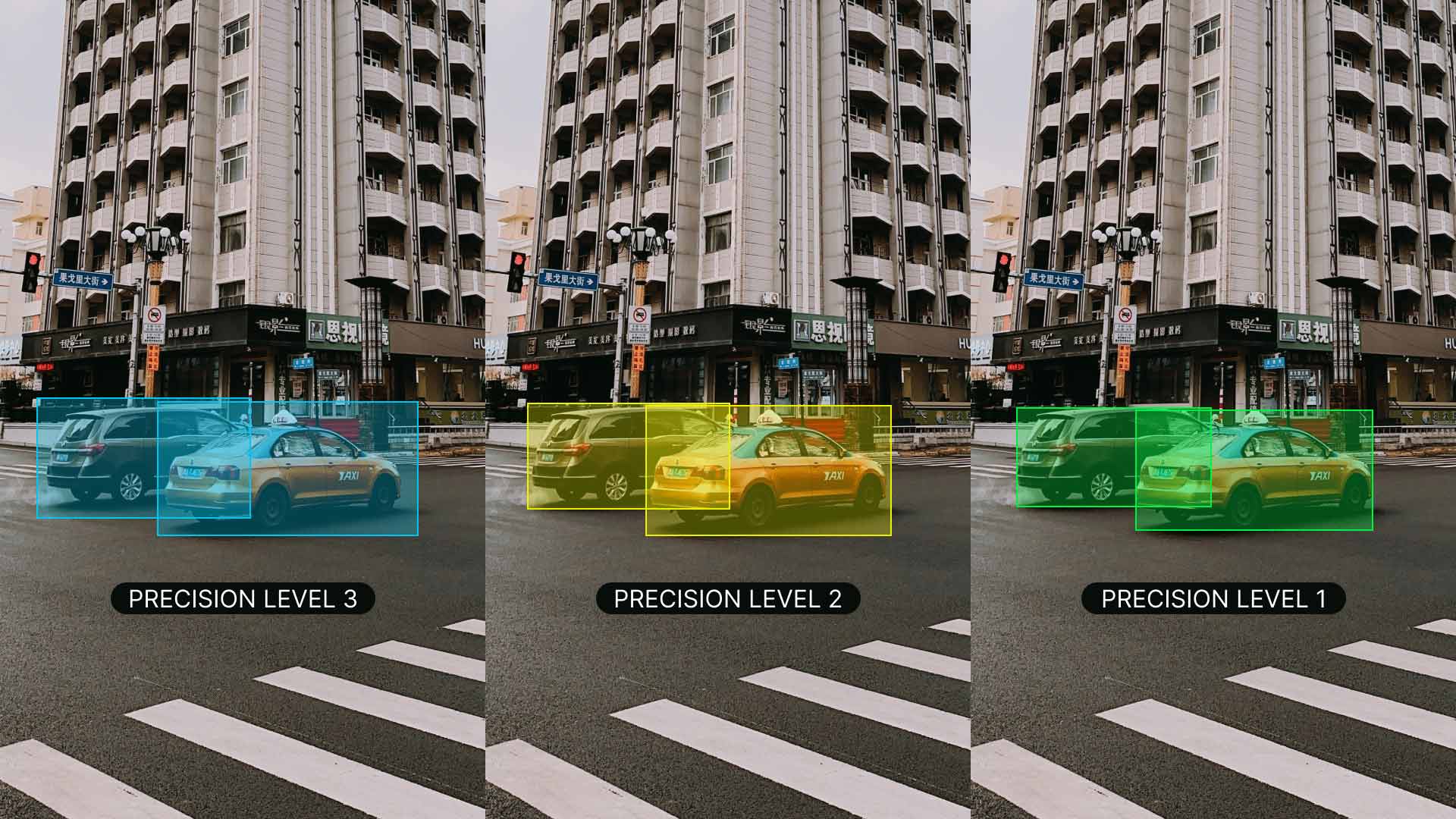
Accurate data annotation is essential for businesses relying on machine learning models to make informed decisions. The importance of calibration cannot be overstated as inaccuracies during this process will lead to imprecise datasets which ultimately impact accuracy and effectiveness between predictions vs actual results obtained by systems using these datasets. With meticulous attention given towards machinery on this process through selecting an appropriate labeling technique with reliable quality assurance will yield better training sets with higher accuracy rates hence forming a solid foundation towards developing AI systems that time over time prove necessary for various applications such as image recognition software used by first responders on rescue missions or medical research systems aimed at mitigating disease outbreaks globally
Understanding The Different Types Of Data Annotation
Data annotation has become increasingly important in recent years as the rise of artificial intelligence (AI) and machine learning (ML) requires large amounts of high quality data for these algorithms to work effectively. Annotation is the process of labeling data in order to make it easier for computers to interpret, and there are several different types of data annotation available, each suited for different kinds of information.
One type is polygon and polyline annotation, which provides pixel-perfect precision when annotating objects in images. This method involves outlining an object with a series of connected points or lines in order to create a specific shape that can be recognized by computer algorithms. Another type is text annotation, which involves adding labels and instructions to raw text so that AI can understand how human sentences are structured for meaning.
Audio and video annotation are also vital components in certain industries, such as healthcare where medical data must be labeled accurately by specialized experts. Regardless of the type of annotation needed, precision is key when training machines to recognize objects or context within datasets. The more precise the labeling is done, the more accurate the subsequent recognition will be.
Understanding the different types of data annotation methods available can help companies choose which approach will provide maximum precision while optimizing resources during their AI development projects. It's important that businesses select an experienced partner for their unique needs who can recommend appropriate strategies based on industry best practices coupled with advanced technical know-how.
Quality Control And Evaluation Of Annotation Results
Ensuring the quality of annotated data is crucial for achieving the desired performance of natural language processing (NLP) algorithms. As such, annotations need to go through quality testing before deployment. This process can involve using simple statistical methods to detect and prevent errors from recurring in a project.
To maintain quality standards, data annotation needs to be valid, precise, reliable, timely and have integrity. These standards should guide the selection and evaluation of annotators as well as make sure that raw data is accurately transformed into usable data for machine learning models.
In addition to selecting high-quality annotators, quality control methods should also be applied throughout all phases of an NLP project. This ensures consistency and accuracy in output while mitigating any errors before they become more difficult or impossible to fix later on in the process.
Evaluation is crucial for monitoring local projects and identifying issues that may affect the annotation output's accuracy and precision. Regular evaluations help improve future iterations of NLP models by refining training processes and identifying areas where performance could improve further.
Ultimately, ensuring high-quality annotations requires a combination of statistical methods at each phase while providing adequate feedback channels for annotator training programs. Laboratory Quality Assurance methodologies are often used as stand-alone frameworks or integrated into broader workflow services like cloud-NLP solutions to enhance analytical results' quality documentation throughout annotation procedures. With accurate annotation results generated using rigorous QA/QC protocols embedded in advanced workflows, developing NLP systems that meet strict industry standards become possible even with huge volumes of incoming text documents.
Continuous Improvement Of Annotation Processes.
Data annotation is a crucial step in many industries, including healthcare, finance, and autonomous vehicles. However, it can also be a time-consuming and tedious task that requires high precision. Inaccurate labeling can have severe consequences on the results of data analysis models. Therefore, it is essential to continually improve annotation processes to ensure the quality of annotated data.
One way to achieve continuous improvement is by making it easy for employees to innovate and share feedback regarding the annotation process. Employee suggestions could identify areas that require improvement, such as reducing manual labor or streamlining repetitive tasks. Identifying outcome goals and metrics that can measure those outcomes will ensure that each optimization targets specific objectives.
Another critical aspect of ensuring accurate annotation is understanding the type of data and annotations required for processing. It is vital to determine the appropriate information at hand before creating an appropriate annotation system or procedure. Operational intelligence about this matter would help you build better systems for continuous improvement cycles.
To facilitate accurate data labeling continually, several process discovery tools assist analysts in optimizing their workflows from initial capture through final output generation seamlessly. Statistical methods are used once performance baselines have been established in order to find ways to improve products or services further accurately; these decisions should be based on precise insights into operational efficiencies gathered from aggregating event logs.
There are several key factors involved when planning how best to continuously improve your annotation processes pipeline's efficiency sustainably - starting with understanding what's necessary from both touch points above all else! By defining clear goals together with measuring your outcomes using meaningful KPIs (Key Performance Indicators), team members will work towards better fit compliance increasingly over time with greater productivity too!
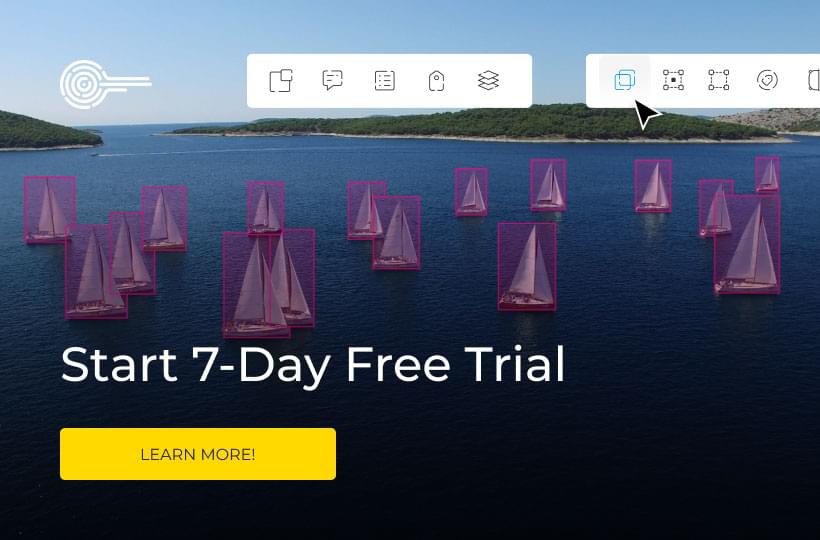