Why We Need An Automated Labeling Tool To Replace Manual Data Annotation
In the optimism of the 60s, researchers expected image-interpreting computers to surpass us. We still can’t take our hands off the wheel of automated data annotation. Human input is always needed at some stage. Automated and manual data annotation are players on the same team, not substitutes for each other.
Looking around the world today, it's not always clear where we use data annotation. Also called data labeling, you’ll see it applied more and more to the consumer experience. It will also have far-reaching impacts in the medical sphere, autonomous driving, and advertising, just to name a few areas.

What Are Manual And Automated Data Annotation?
Manual data annotation is the human element. The data, in this case, are images, video, documents, and even music. We need to categorize and label certain elements of these content sources, something that AI hasn’t perfected. It's unlikely to become obsolete any time soon, even as machine deep learning progresses. The human eye remains the gold standard, for now.
Automated data annotation is a focus for many cutting-edge AI companies. Using algorithms and model training, the hope is that we can expedite the data annotation process. Programs often receive input from expert annotators, called the Human Machine Interface. The HMI sets parameters, monitors, and adjusts an auto labeling tool throughout the automated process. Human supervision delays the process, but it's necessary. An AI labeling tool can label multitudes faster than its mortal counterparts.
Typical Uses Of Manual Data Automation
Technical terms like these can slow down our understanding sometimes. We’re going to provide you with a few real examples of manual data annotation.
Self Driving Technology
Annotators manually label vehicles and other important information from video sources. They also define the areas of objects and note characteristics, such as turn signals, with a manual image labeling tool. At the same time, they’ll make sure that the vehicle’s radar system has noted the objects.
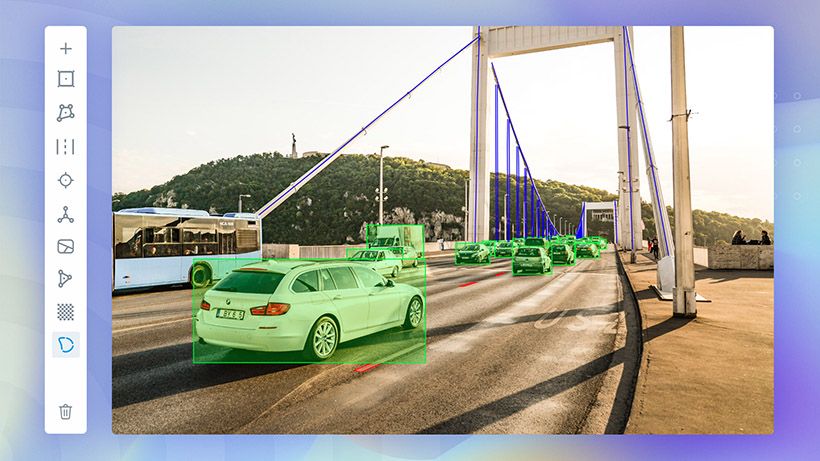
Medical Labeling
Every level of medical care will be positively affected by data annotation. X-rays, CT scans, MRIs, and even surgery videos are being labeled by experienced medical annotators. This means that things like robotic surgery and advanced pre-screening will be automated in the future.
Document Labeling
Raw text needs to be labeled and classified if it’s going to be properly used for machine learning. Natural language processing highlights important sections of text and tags them. Thanks to manual labeling, the data can be used for machine learning.
Dialogue Labeling
Chatbots are more prevalent in online support than ever, and they need training that comes from labeling conversations. Manual data annotation is still used, but fully automated programs are applicable here. User responses are recorded and used to enhance the chatbot’s support capabilities, saving businesses on manpower costs.
Why We Still Need Manual Data Annotation [For Now]
Automated data labeling isn’t a dog that we are fully ready to take off the leash. AI learning advances in recent history have been monumental, but humans still need to supervise. Self-driving technology badly needs automation, but it also requires a high level of precision. The cost of an automated process making a mistake on public roads could have major consequences.
One model for automated data annotation works on a confidence level. Whenever the program is slightly unsure about a section, it’s passed on to human annotators. In most processes, labeling starts automatically with machines before being polished by mortals.
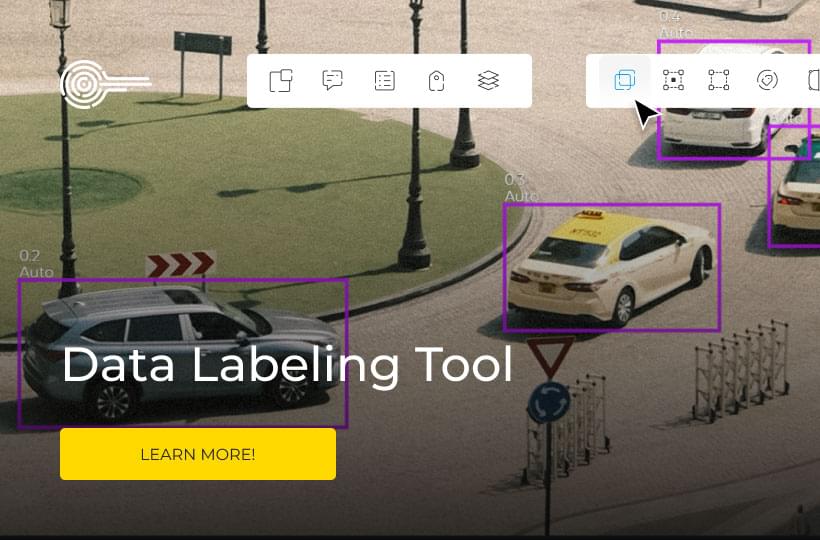
The Importance Of Automated Data Annotation
A professional in the automated annotation field gave an example of where billions of objects needed labeling for a self-driving program. He estimated a single project of 300,000 km of driving footage would require more than fifteen million human labor hours. This perfectly illustrates the problem manual data annotation faces and the need to safely automate the process.
Crafting a labeling tool for AI poses a few obstacles, but the industry is making huge strides. Once we’ve built programs with a high enough level of labeling accuracy, the tech advancements will be rapid. Some sources already use fully automated processes that independently accept or reject labels without human input. Whether or not we can use a program like that depends on the real-world application. There’s less leniency with medical or self-driving vehicle data, but document labeling lends itself well to full automation.