Beyond Classification: Exploring Advanced Tasks in AI Image Recognition
From the minute intricacies of healthcare diagnostics to the broad vistas navigated by autonomous vehicles, AI's eyes have become indispensable. With the power to interpret complex visual information, advanced AI image recognition tasks have significantly transformed sector after sector.
The magic doesn't end with differentiation between objects or understanding pedestrian traffic; it delves deeper into recognizing emotional cues in social media and bolstering security through nuanced facial recognition systems. However, as we advance, we encounter a myriad of challenges: ethical dilemmas, privacy concerns, and biases—all of which are critical to address as we continue to harness the visual tech capabilities granted by AI. Let us unpack the layers of AI image recognition and explore the advanced tasks that propel industries toward a more intelligent future.
Key Takeaways
- AI image recognition extends far beyond basic tasks, encompassing complex applications in healthcare, autonomous vehicles, and security.
- Deep learning, particularly CNNs, is fundamental to the evolution and effectiveness of image recognition technology.
- Advanced tasks like object detection and image segmentation have revolutionized the way AI systems interact with and understand visual data.
- Addressing challenges like data biases and privacy concerns is as crucial as the technological advancements themselves.
- With constant improvements, AI image recognition is instrumental in driving forward-looking innovation across an array of fields.

Unveiling the Potential of Advanced AI Image Recognition Tasks
Advanced AI image recognition tasks are not just about technology; they represent a transformative leap in how machines perceive and interact with the visual world. These advancements extend well beyond conventional applications, forging new paths in sectors such as healthcare, automotive, and agriculture.
Understanding the Importance of Advanced Tasks in AI
At the core of AI's evolution, tasks like object detection and image segmentation play critical roles. These technologies enable machines to parse and understand complex scenes in real-time, enhancing decision-making processes and operational efficiencies. For instance, in healthcare, AI-driven image segmentation helps in pinpointing anomalies during diagnostic assessments, thereby boosting both speed and accuracy. The transformative impact of such technologies extends to autonomous driving systems as well, where precise object detection is vital for vehicle safety and navigation.
The Evolution of Image Recognition Technology
Advanced AI image recognition has progressed significantly with the advent of sophisticated algorithms like convolutional neural networks (CNNs), which enhance machine capability in visual content analysis. These developments not only sharpen the accuracy of image recognition systems but also expand their applicability across diverse fields—from aiding radiologists in medical diagnostics to improving product recommendations in retail.
The journey from basic pattern recognition to advanced analysis illustrates AI's growing competence in understanding the visual language. This progression highlights the importance of nurturing such technologies to harness their full potential, ensuring industries can adapt to AI's capabilities and integrate them seamlessly into daily operations.
The Intricacies of Machine Learning in Advanced Image Recognition
Machine learning algorithms, especially those in the domain of deep learning like convolutional neural networks (CNNs), have revolutionized the field of advanced image recognition. By leveraging these sophisticated models, the transition from conventional computer vision techniques to more advanced methods has enabled unprecedented progress in how machines interpret complex visual information.
These machine learning algorithms enhance AI's ability to categorize images, detect and identify specific objects, and decipher scene compositions with astonishing accuracy and speed. Such capabilities are particularly evident in applications that range from facial recognition systems to autonomous vehicle navigation, illustrating the seamless integration of computer vision and deep learning.
From Basic Algorithms to Complex Neural Networks
Initially grounded in basic pattern recognition, machine learning algorithms have now grown into encompassing intricate tasks such as object detection and image segmentation. This evolution is underpinned by the algorithms' ability to recognize and extract detailed features from an image, essentially training the AI to see and understand its environment in a way that mimics human vision but with a capacity for greater scalability and precision.
Feature | Impact of Machine Learning | Impact of Deep Learning |
---|---|---|
Facial Recognition | Identification and verification of individuals | Enhanced accuracy in diverse demographics |
Medical Image Analysis | Recognition of patterns in X-rays and MRI scans | Accurate disease detection and diagnosis |
Autonomous Vehicles | Basic navigation and obstacle avoidance | Complex navigation and decision-making in real-time |
Security Systems | Standard object detection | Advanced surveillance through precise object localization |
The utility of deep learning, manifested through its core components like neural networks, is particularly substantial in areas requiring high fidelity in image and speech recognition, among other advanced AI applications. Despite the complexity and potential high costs associated with deep learning, its ability to process vast and complex datasets efficiently makes it a formidable tool in the AI toolkit, particularly when the intricacy of the problem and the volume of data justify its use over traditional machine learning algorithms.
Prioritizing Security and Ethics in AI Image Recognition
Data integrity is critical in maintaining the reliability of AI systems. Without robust validation and integrity checks, there's a risk that data may be manipulated, which could compromise the fairness and effectiveness of facial recognition technologies. This aspect of AI development highlights the need for stringent controls and transparent practices to guard against data breaches and misuse.
The use of AI in sensitive areas, such as surveillance, necessitates a balanced approach that respects individual privacy while ensuring community safety. The integration of policies that check unethical use or bias in AI algorithms is essential, in order to sustain public trust in how these technologies are applied. This is particularly true for facial recognition with AI, a tool that has stirred public debate over privacy rights and surveillance.
Creating an ethical AI framework involves establishing clear guidelines and regulations that determine the operation and limits of these technologies. This needs to work hand-in-hand with promoting a culture of responsibility among AI practitioners and organizations, emphasizing ethical AI practices in all stages of AI system development and deployment.
Ethical Issue | Strategy to Mitigate |
---|---|
Bias in AI | Implement diverse data sets to train algorithms |
Data Privacy Concerns | Use techniques such as homomorphic encryption |
Misuse in Surveillance | Regulate and set clear limits on AI surveillance applications |
Unintended Data Leakage | Rigorous data protection measures and continuous monitoring |
Tackling these challenges involves not only technological solutions but also a fundamental commitment to ethical principles at every level of system creation and implementation. Ensuring the ethical deployment of AI technologies, particularly those involving facial recognition, requires an ongoing conversation and cooperation between developers, legislators, and the global community to align on standards that protect privacy and foster trust.
Fine-Tuning Object Detection for Greater Precision
The relentless advancement in technology has ushered in a new era where enhanced object detection is not just a possibility but a necessity. Powered by deep learning, these technologies are fine-tuned to tackle the demands of processing real-time visual data efficiently. This progression is crucial for safety in autonomous vehicles and maintaining security with vigilant surveillance systems.
Real-World Applications of Enhanced Object Detection
Enhanced object detection has transformative implications across multiple sectors. In surveillance and security, it aids in the rapid identification of potential threats, while in autonomous vehicles, it is indispensable for the recognition of pedestrians and obstacles to ensure safe navigation. Notably, the retail sector uses these advancements for inventory management and tailoring customer experiences, whereas healthcare benefits from early diagnosis capabilities through medical imaging detection.
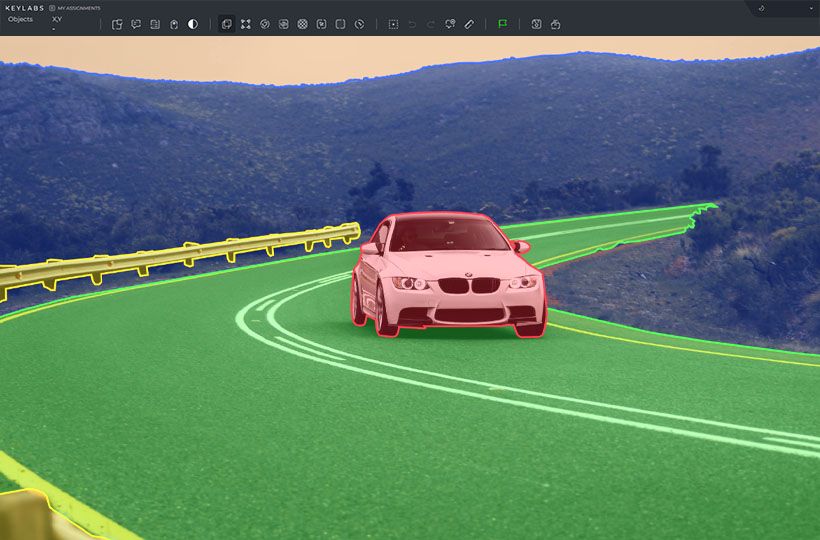
The Role of Deep Learning in Modern Object Detection Technologies
At the core of modern enhanced object detection systems lies deep learning, significantly elevating the ability to analyze complex visual data intricately. Technologies such as YOLO (You Only Look Once) and Faster R-CNN have proven instrumental. YOLO excels in real-time detection, allowing for immediate interpretation of visual data, which is critical in environments requiring rapid responses, such as in traffic management and emergency scenarios.
These deep learning models thrive on large datasets and continuously improve through reiterative training. They are adept at distinguishing finer details that evade traditional models, thereby improving accuracy and functionality in real-world applications. The convergence of advanced algorithms like CNNs and region-based CNNs enhances the precision of these systems, ensuring that enhanced object detection remains at the forefront of innovation in AI technology applications.
Mastering Image Segmentation Techniques in AI
In the realm of artificial intelligence, image segmentation is a pivotal technique that enhances machine interaction by breaking down images into distinct, meaningful regions. This capability is instrumental in fields ranging from medical imagery to autonomous navigation, offering both challenges and innovative solutions.
Case Studies: Medical Imagery and Autonomous Navigation
Medical imagery leverages image segmentation to accurately delineate boundaries within biological structures. For example, it enables the precise isolation of tumors from surrounding tissues in oncology, aiding in both diagnostics and treatment planning. In the context of autonomous navigation, segmentation techniques inform the visual systems of autonomous vehicles, allowing them to distinguish roads, obstacles, and navigable pathways efficiently, ensuring safe and effective transportation.
Challenges and Solutions in Advanced Segmentation
Despite its benefits, image segmentation presents certain challenges, such as differentiating between overlapping objects or similar textures. Advances in deep learning have led to the development of more sophisticated segmentation models that can tackle these complex scenarios with greater accuracy.
Technique | Application | Advantages |
---|---|---|
Thresholding | Basic image separation | Simple to implement, effective for high contrast images |
Graph-based segmentation | Detecting nuanced patterns | Detailed object distinction |
Instance Segmentation | Autonomous vehicles | Individual object analysis |
Semantic Segmentation | Medical imaging | Precise pixel-level labeling |
Through ongoing research and technological advancements, the field of image segmentation continues to evolve, offering profound implications across various sectors that rely on nuanced image analysis. Keylabs provides state-of-the-art tools for all kinds of annotation.
Facial Recognition with AI: Beyond the Surface
As facial recognition technology integrates more deeply into our daily lives, from unlocking smartphones to enhancing security protocols, its evolution is markedly geared towards creating an enhanced user experience and accommodating a broad spectrum of demographics. This development emphasizes not just technological advancement but also an acute awareness of the diverse needs and ethical considerations that come with widespread deployment.
Improving Accuracy in Diverse Demographics
The efficacy of facial recognition with AI pivots significantly on its performance across different demographics. Historical data and recent studies underline the necessity for algorithms that do not discriminate or exhibit bias. The ambition is to cultivate a system where technology serves everyone equitably. For example, findings from the National Institute of Standards and Technology reveal that many existing algorithms exhibit diminished accuracy when identifying people of color, which may amplify existing biases. Consequently, continuous improvements in algorithm training are critical to ensure that facial recognition with AI tools deliver unbiased and accurate results across all demographics.
Facial Recognition: A Tool for Enhanced User Experience
Facial recognition with AI is poised to revolutionize the user experience by personalizing interactions and simplifying identification processes. Its application ranges from personalized advertisements on digital platforms to enhanced security measures that allow for a seamless, secure, and rapid verification process. Integrating facial recognition systems can significantly streamline operations in various sectors such as retail, banking, and especially large events where security is paramount. This integration ultimately augments user satisfaction by making systems more intuitive, responsive, and accessible.
Exploring the Dual Nature of AI Image Recognition: Potential and Risks
The advent of AI image recognition technology promises revolutionary applications, yet it also presents a complex array of ethical dilemmas requiring thorough exploration. As we delve into the AI image recognition potential, it becomes imperative to carefully navigate the ethical landscape, considering both the benefits and the inherent risks associated with this technology.
Navigating the Ethical Landscape of AI
AI image recognition technology offers remarkable capabilities from enhancing security measures to advancing healthcare diagnostics. However, its rapid development and deployment raise significant ethical concerns that must be addressed to prevent misuse and protect societal values. Critical issues include privacy intrusions, consent for data usage, and transparency in AI operations.
Furthermore, the risk of perpetuating biases through AI systems can lead to unfair outcomes, notably in sectors like employment and law enforcement, where AI-driven decisions can significantly impact human lives. Ethical navigation in AI requires a proactive approach in developing regulations and frameworks that ensure responsible use, prioritizing human rights and fairness.
Consider, for instance, the deployment of AI in healthcare, where predictive analytics has remarkably increased early disease detection rates by 25%. While this showcases the potential of AI to save lives, it also necessitates stringent measures to guard against data mismanagement or breaches that could jeopardize patient privacy.
Similarly, in the transportation sector, while self-driving cars powered by AI promise to improve road safety and accessibility, they also pose autonomy risks that could have severe unintended consequences. It is not just about creating technology but also about ensuring it operates within ethical boundaries that do not compromise human safety and security.
To illustrate the dual aspects of AI image recognition—its potential and risks—consider the following comparative table:
AI Image Recognition Potential | Associated Risks |
---|---|
Enhanced disease detection in healthcare | Potential for privacy breaches with patient data |
Improved efficiency in transportation via autonomous vehicles | Risks of autonomous decision-making in unpredictable scenarios |
Increased productivity through AI-driven automation | Job displacement concerns, especially in manual roles |
The responsibility lies in leveraging AI image recognition's potential while diligently mitigating its risks through comprehensive ethical guidelines and robust regulatory frameworks. This ensures that as we progress with this formidable technology, its deployment remains aligned with enhancing societal well-being rather than detracting from it.
Leveraging Transfer Learning for Efficient AI Image Recognition
In today's fast-evolving digital landscape, transfer learning has emerged as a cornerstone for enhancing the capabilities of AI image recognition systems. By utilizing pretrained models, developers are able to achieve more with less—reducing the need for extensive data sets and computational power traditionally required to train AI models from scratch.
Transfer learning essentially allows developers to "stand on the shoulders of giants," leveraging the complex features that models have learned from extensive, diverse datasets. This not only shortens development cycles but also lowers the barriers for innovation in AI image recognition, making it not just more efficient but also more accessible.
Enhancing Capabilities with Pre-Trained Models
The practical applications of such enhanced capabilities are vast, ranging from real-time object recognition in mobile devices, which can transform consumer technology, to sophisticated diagnostic tools in healthcare that offer faster and more accurate diagnosis without the need for large volumes of medical imaging data.
Overall, the ability of transfer learning to utilize existing pretrained models breaks traditional barriers and sets a new efficacy standard in AI image recognition—making it an indispensable strategy in the toolkit of modern AI developers.
Summary
As we have journeyed through the dynamic terrain of AI and image recognition systems, it is evident that the future of AI is being intricately woven by the threads of deep learning, transfer learning, and progressively sophisticated algorithms. These technologies have not only carved a niche for themselves within the confines of data analysis but have expanded their reach, engaging in a silent dance with real-time object detection performances that continue to awe and inspire. The disruptive strides made from the Mask RCNN algorithm in 2017, cruising through the 12ms inference benchmarks of the YOLOR algorithm, to the inception of the pioneering YOLOv9 in 2024, have been nothing short of remarkable. Each iteration bringing us closer to real-time responsiveness—a coveted milestone in the lineage of image recognition advancements.
Our reliance on visual cognition, as highlighted by the statistic that 90% of information transmitted to the human brain is visual, is echoed in the developmental trajectory of these image recognition systems. They not only mirror this innate capacity but extend it through capabilities such as face, text, and pattern recognition—each finding its place within a wide array of sectors, from the bustling terminals of airports to the precise nuances of finance, healthcare, and beyond. The expanding global market, the enthusiastic reception of visual search technology by millennials, and the ubiquitious presence of AI in retail are testimonies to the transformative effect and adoption of such technology.
FAQ
What are advanced AI image recognition tasks?
Advanced AI image recognition tasks go beyond basic image classification to include more complex capabilities such as object detection, image segmentation, and facial recognition. These tasks help AI analyze and interact with visual data, providing insights for applications ranging from autonomous vehicles to medical diagnostics.
Why are machine learning algorithms essential in advanced image recognition?
Machine learning algorithms, particularly convolutional neural networks, are fundamental in advanced image recognition as they enable AI to learn from vast amounts of visual data, extract features, and perform complex tasks such as object detection and image segmentation with a high degree of accuracy and efficiency.
How does AI enhance object detection capabilities?
AI enhances object detection by using deep learning techniques, which improve the precision and efficiency of detecting objects in real-time visual data. Deep learning algorithms can identify specific objects within complex scenes, leading to more accurate and functional applications in various industries, including security and transportation.
What role does image segmentation play in AI?
Image segmentation plays a crucial role in dividing an image into significant regions, aiding in tasks such as medical imagery analysis and autonomous navigation. By mastering segmentation techniques, AI can more effectively interact with its environment and assist in tasks that require detailed visual understanding.
What are the main ethical concerns of facial recognition with AI?
The main ethical concerns of facial recognition with AI include privacy issues, consent, and potential biases in training data that can lead to unfairness or inaccuracies. Addressing these concerns is essential in developing responsible and trustworthy AI systems that respect individual privacy and ensure equitable treatment across different demographics.
How does transfer learning benefit AI image recognition?
Transfer learning benefits AI image recognition by utilizing pretrained models to kick-start the learning process, which drastically reduces the time and resources required to train a model from scratch. This enables the rapid deployment of AI systems across various tasks and improves the accessibility of advanced image recognition technology.
What are the challenges faced in modern object detection methods?
Modern object detection methods face challenges such as correctly identifying and distinguishing between similar objects, dealing with overlapping objects, and processing visual data in real-time with high accuracy. Ongoing research and enhancements in deep learning algorithms are aimed at overcoming these challenges.
How does image recognition technology transform industries?
Image recognition technology transforms industries by automating and enhancing tasks that require visual understanding. In healthcare, it aids in disease detection; in retail, it improves the shopping experience; in agriculture, it supports crop monitoring; and in security, it facilitates facial recognition systems, leading to a transformative impact across sectors.
What future developments can we anticipate in AI image recognition?
We can anticipate continued advancements in deep learning, more sophisticated algorithms for object detection and image segmentation, and improvements in fairness and accuracy for facial recognition. Efforts to address ethical concerns and ensure data privacy will shape the future of AI in image recognition, as will innovations that increase precision and applicability.
How can biases in facial recognition systems be addressed?
Biases in facial recognition systems can be addressed by diversifying training datasets, improving algorithmic design to prevent discrimination, and performing rigorous testing across different demographics to ensure enhanced user experience and fair treatment for all individuals, irrespective of their background.
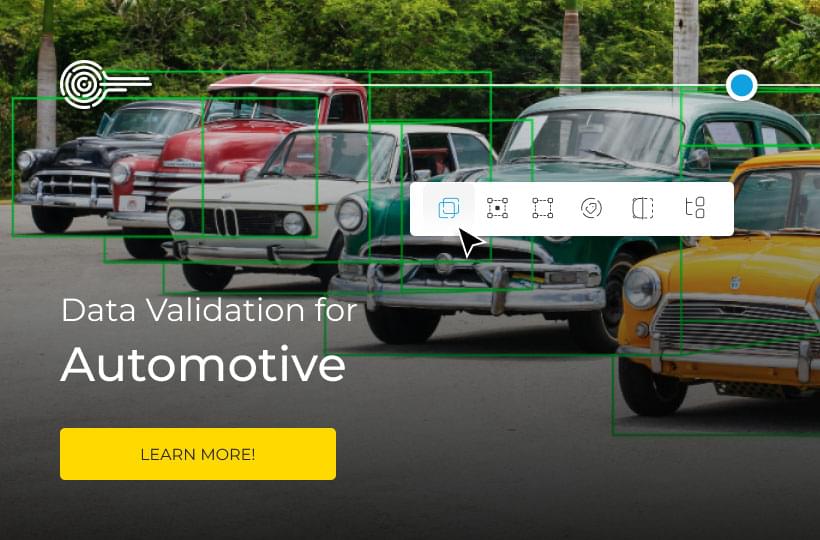