Seeing Through Machines: A Comprehensive Guide to AI Image Recognition
In 2017, the fastest real-time object detector was the Mask RCNN algorithm, clocking in at 330ms per frame on the MS COCO benchmark. Yet today, image recognition through AI has made leaps so profound that models like the YOLOv8, launched in 2023, now deliver state-of-the-art performance, shattering previous records in real-time detection. This rapid advancement within such a short span is a testament to the relentless progress in AI image recognition explained through sophisticated algorithms and machine learning.
On the cusp of this technological revolution, it is remarkable to see how machine learning models have evolved to use computer vision with AI. From early reliance on networks taught to categorize imagery into programmed labels, these algorithms have grown into self-optimizing systems capable of image classification, object detection, and intricate image segmentation. These advances are not just academic milestones but are driving real-world solutions, powered by high-quality training datasets and enhanced by platforms like Keylabs.
Key Takeaways
- The evolution of AI image recognition manifests in advancing algorithms from Mask RCNN to YOLOv9.
- Current AI systems rely on extensive, labeled datasets that inform the machine's learning algorithms.
- Speed and accuracy benchmarks in AI image recognition are continuously redefined, showing rapid technological progress.
- The success of image recognition hinges on both the quality of the dataset and the architecture of the neural network.
- The dynamic journey from RCNNs to convolutional neural networks highlights the transformation in machine vision capabilities.
- Real-world applications of AI image recognition have far-reaching implications across a variety of sectors.

The Dawn of AI Image Recognition
From the simple rule-based algorithms of the past to the sophisticated YOLO algorithms of today, AI image recognition has undergone transformative evolution. The integration of deep learning and neural networks in AI has paved the way for these advanced technologies capable of parsing complex visual inputs more accurately than ever before.
Understanding AI Image Recognition
AI image recognition is based on the ability of computer vision systems to interpret images and videos using artificial intelligence. This process employs deep learning models that analyze thousands of image data points to extract and learn features automatically. YOLO algorithms, especially in their newer versions, employ neural networks in AI to categorize and predict objects within images with remarkable speed and accuracy.
The Evolution from Human to Machine Vision
Initially inspired by the cognitive capabilities of the human eye, machine vision has fundamentally shifted to a model heavily reliant on data-driven learning. Unlike human vision, which is intuitive and relies on organic neural pathways, machine vision develops through structured learning from vast datasets of labeled images. Tools like Keylabs are used for labeling thousands of images to create datasets for computer vision.
Foundational Technologies Powering Image Recognition
At the core of modern image recognition, technologies lie advanced deep learning frameworks capable of detailed image analysis. The training process involves feeding neural networks with large datasets. These neural networks, refined by layers of deep learning, learn to identify and predict visual elements with increasing precision. The progression from YOLOv4 to YOLOv9 over just a few years underscores the rapid advancements achieved in this domain, highlighting the critical role of evolving algorithms in driving forward the capabilities of AI image recognition.
In developing these complex systems, the labeling of data remains a significant challenge, requiring extensive manual effort to prepare datasets for training. However, the benefits are clear, as the latest advancements in YOLO algorithms demonstrate their ability to leverage deep learning to significantly improve performance and efficiency, making them superior to traditional image processing methods.
Breaking Down How AI Image Recognition Works
The realm of AI image recognition is driven by a synergy of complex neural network algorithms, sophisticated object detection methodologies, and refined image classification mechanisms. To understand these intricate processes, it's essential to consider how traditional computer vision has evolved into an AI-dominated landscape.
Traditionally, computer vision involved a series of stages including filtering, segmentation, feature extraction, and finally classification. Each step required expert knowledge and significant manual effort to tune algorithms for decent performance. However, the introduction of neural network algorithms revolutionized this field, simplifying and enhancing these processes dramatically.
Modern image recognition uses deep learning models where neural networks learn from vast datasets. These networks, layered like an onion, each layer's processing contributing uniquely towards the final output, allow for the automatic detection and classification of objects within images. Machine learning, a critical backbone of this technology, enables systems to improve over time, learning from new data and prior mistakes, thus enhancing accuracy in tasks like real-time object detection.
The need for powerful computational resources and extensive data for training these models presents notable challenges. Innovations in data compression techniques and improved algorithmic efficiency continue to make AI image recognition more accessible and cost-effective. Below is an overview of key sectors where AI image recognition is making a substantial impact:
- Ecommerce platforms utilize image recognition for enhancing user experiences through personalized recommendations and visual search capabilities.
- Automotive industries rely on this technology for advanced driver-assistance systems (ADAS).
- In healthcare, AI facilitates faster, more accurate diagnostics through tools like automated MRI and CT image analysis.
- The gaming industry uses AI to create more immersive, interactive environments that react to player movements and actions in real-time.
Advancements in neural networks have not only enabled the enhancement of basic image processing techniques but also fostered the integration of AI image recognition into various applications from secure access control in buildings using biometrics to quality control in manufacturing. As such, the potential of AI image recognition continues to grow, promising to reshape numerous industries profoundly.
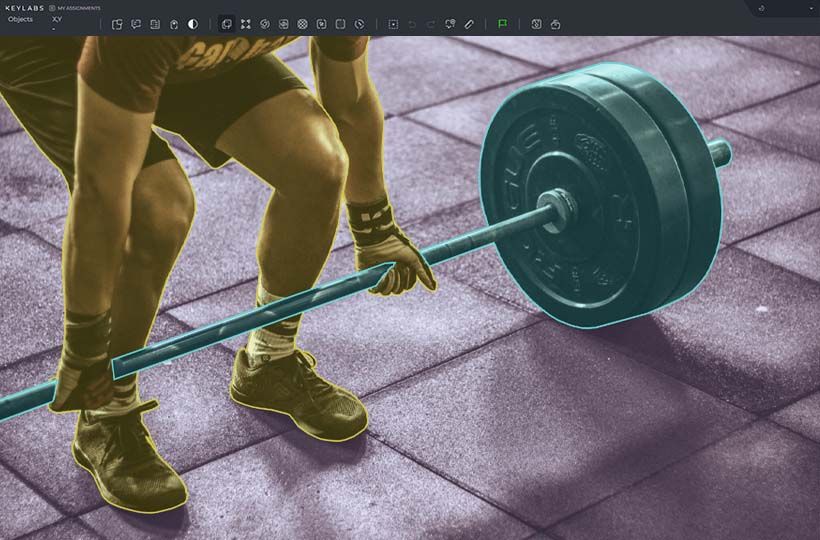
Capturing the Visual World: The Role of Data in AI Image Recognition
In the realm of AI image recognition, the success of a model largely hinges on the robustness of the dataset acquisition, image preprocessing, and feature extraction processes it relies on. This section explores how data serves as the cornerstone for creating effective and intelligent recognition systems that can mimic and even surpass human visual capabilities.
The Importance of Diverse Data Sets in Training AI
To train AI systems that perform with high reliability and accuracy across various scenarios, a meticulously curated dataset is essential. Such datasets not only help in refining the algorithm's ability to generalize across different visual inputs but also reduce biases which might otherwise occur with less diverse data. Proper dataset acquisition should aim to represent a wide array of characteristics such as different lighting conditions, angles, and backgrounds which the AI is expected to handle in the real world.
Data Annotation and Its Impact on Machine Learning
Data annotation, the process of labeling data, is a pivotal part of preparing data for machine learning in AI image recognition. Labels must accurately reflect the content of images so that the trained models can learn precise patterns and nuances. This meticulous process enhances the model's ability to not only recognize but also understand the context of the images, a critical factor in applications such as autonomous driving and healthcare diagnostics.
From Raw Pixels to Meaningful Patterns
The transformation from raw visual data into meaningful patterns through image preprocessing and feature extraction is a marvel of modern AI technology. Image preprocessing often includes resizing, converting to grayscale, or normalizing images to a consistent scale. Subsequently, feature extraction, usually performed through advanced convolutional neural networks, allows the AI to detect and learn complex patterns in the data. This stage is crucial for the success of machine learning models, enabling them to make accurate predictions or categorizations in real-world applications.
Each stage of dataset preparation—acquisition, annotation, preprocessing, and extraction—plays a critical role in the effectiveness of AI image recognition systems. By strengthening these foundational aspects, the technology advances closer to creating systems that seamlessly integrate into various aspects of human activity, backed by data that mimic the depth and complexity of the real visual world.
AI Image Recognition Explained: An Overview of Key Processes
The realm of AI image recognition has become a cornerstone technology across various industries, powered by robust machine learning applications and sophisticated modelling techniques. Central to these advancements are convolutional neural networks (CNNs), which excel in analyzing visual imagery by segmenting and classifying detailed components within the images.
One of the most critical aspects of this technology is object segmentation, a process that isolates and categorizes each object within an image. This method has transformed how machines perceive and interact with visual data, turning raw images into a structured understanding that can be further analyzed and acted upon.
Technological milestones in recent years underscore the progress and impact of AI image recognition. For instance, the introduction of the YOLOR algorithm in 2021, a successor to the famed YOLO algorithm series, demonstrated inference times as short as 12ms per frame on benchmarks like MS COCO—a remarkable enhancement that pushed the boundaries of real-time object detection.
Furthermore, the Segmentation Anything model, introduced in 2023, became the gold standard for image segmentation tasks, showcasing the continuous innovation in machine learning applications. Following this, YOLOv9's release in early 2024 presented a new model architecture that has dramatically refined the efficiency and accuracy of training object detection AI models.
These strides are not just about speed or accuracy; they also reflect a reduction in the dependency on extensive training data sets, a common constraint in early machine learning scenarios. Modern techniques can perform with fewer data but higher precision, a benefit significantly powered by advancements in convolutional neural networks.
Algorithms at Play: Traditional vs. Deep Learning Approaches
The landscape of image processing has witnessed a significant paradigm shift, transitioning from manual extraction methods to advanced self-learning systems. Traditionally, techniques like Support Vector Machines (SVM), Histogram of Oriented Gradients (HOG), and Local Binary Patterns (LBP) dominated the field. However, the advent of deep learning technologies, particularly Convolutional Neural Networks (CNNs), has set new benchmarks in the efficiency and effectiveness of image recognition tasks.
Comparative Analysis of Traditional Image Processing Techniques
Traditional image processing techniques, such as SVM, HOG, and LBP, have been fundamental in laying the groundwork for pattern recognition. SVM has been invaluable for classification tasks, HOG played a pivotal role in object detection scenarios, and LBP was instrumental for texture classification. However, each of these techniques required extensive manual tuning and expert intervention to achieve desired outcomes, making them less adaptable to new, unstructured data without significant reconfiguration.
Unlocking Potentials with Deep Learning Models
Deep Learning, ignited in the realm of image processing in 2012, has surpassed traditional methods in terms of adaptability and accuracy. Unlike its predecessors, DL thrives on end-to-end learning capabilities that eliminate the need for manual feature selection. This ability to self-learn and improve over time allows it to manage unstructured data effectively, making it a potent tool in current and future applications of AI in image analysis.
One of the most significant advantages of deep learning models is their minimal requirement for expert analysis, which contrasts sharply with traditional techniques that often hinge upon the nuanced expertise of seasoned practitioners. Moreover, CNNs, a class of deep learning, have been revolutionary, recognizing and learning complex patterns directly from the data without human-encoded guidelines.
Case Study: The Rise of Convolutional Neural Networks
The development of CNNs has dramatically enhanced the field of computer vision, empowering systems to achieve nuanced recognition tasks that mimic and often surpass human capabilities. These networks automatically detect and prioritize the most informative features without explicit programming, a stark departure from methods that demand explicit feature definition.
Equipped with multiple layers, CNNs have enabled advancements in numerous real-world applications, ranging from automatic photo tagging in social media to enhancing autonomous vehicle technologies. This adaptability across diverse domains showcases the robust utility and scalability of deep learning models over traditional approaches.
In conclusion, as deep learning continues to evolve and refine, its applications seem boundless compared to the more static traditional computation methods. Not only do these technologies improve the efficiency and accuracy of image processing, but they also propel the underlying hardware advancements, enabling more complex and comprehensive image recognition tasks across industries.
Real-World Applications of AI in Image Analysis
The integration of AI in image analysis has significantly transformed multiple sectors by enhancing visual data interpretation, streamlining trend analysis, and optimizing AI-enhanced decision-making. From healthcare to retail, AI image recognition technologies play a pivotal role in daily operations and strategic planning.
In healthcare, AI excels in identifying anomalies in medical images, providing earlier diagnoses, and supporting preventive medicine strategies. Precision agriculture benefits as well, where AI-driven image analysis aids in monitoring crop health, predicting yields, and optimizing resource distribution, thus making farming more sustainable and efficient.
The automotive industry leverages this technology for safer navigation solutions in autonomous vehicles through real-time pedestrian and obstacle detection. Retailers use AI image recognition to analyze customer behavior, optimize store layouts, and manage inventories effectively, leading to enhanced customer experiences and business operations.
Moreover, the security domain utilizes these advancements for surveillance and threat detection, drastically improving the safety and security protocols in public and private spaces. Each application not only showcases the technology's broad usability but also illustrates its role in driving forward-looking decisions in business and governance.
Industry | Application | Benefits |
---|---|---|
Healthcare | Anomaly detection in scans | Early diagnosis, enhanced patient care |
Agriculture | Crop health monitoring | Increased yield, resource efficiency |
Automotive | Pedestrian and obstacle detection | Improved navigation, vehicle safety |
Retail | Store layout optimization | Enhanced shopping experience, inventory management |
Security | Surveillance and threat detection | Improved public and private security measures |
These diverse applications highlight the critical role of AI in not only interpreting visual data but also in harnessing this information to forge data-driven strategies that propel industries towards efficiency, sustainability, and security.
Tools of the Trade: Software and Hardware in AI Image Recognition
In the realm of AI image recognition, choosing the right combination of hardware and software is crucial for achieving optimal performance and efficiency. Recognizing this intertwining dependence, we delve into the advanced realms of GPU computing, AI hardware requirements, and specialized software for image recognition that empower today's applications.
Selecting the Right Hardware for Image Processing
AI hardware requirements stipulate robust processing capabilities to handle extensive computations, particularly deep learning tasks. GPUs are at the heart of this process, providing the necessary speed and efficiency. GPU computing accelerates the algorithmic calculations needed for training and running deep learning models, which is essential in analyzing vast amounts of image data.
Review of Popular AI Image Recognition Software
Navigating through the complexities of software for image recognition reveals a range of platforms designed to cater to various industry needs. Software solutions not only need to adequately leverage the raw power of the hardware but also provide versatility and customization options that can be tailored to specific tasks. TensorFlow and PyTorch have gained popularity for their end-to-end functionalities that facilitate from image data handling to model deployment.
Customizing AI Tools for Specific Image Recognition Tasks
Each image recognition task carries unique challenges and requirements, which necessitates customized software tools. This customization is possible through modular software architectures that integrate seamlessly with existing systems and allow for the extension with specific modules to enhance functionality, such as object detection, facial recognition, or pattern analysis.
Feature | Viso Suite | TensorFlow | PyTorch |
---|---|---|---|
Real-Time Processing | Yes | Limited | Yes |
Hardware Compatibility | High | High | Varies |
Customization Level | High | Medium | High |
AI Model Integration | Extensive | Extensive | Extensive |
Data Handling | Optimal | Needs Optimization | Optimal |
The interplay between hardware's GPU computing capabilities and the flexible, powerful software for image recognition is what ultimately drives the field forward, enabling faster processing times and enhanced performance metrics critical to modern AI applications.
Transformative Impact: AI Image Recognition across Industries
In the evolving landscape of technology, the innovation in digital imagery has notably transformed the functionality and reach of various sectors, notably healthcare, agriculture, and retail. AI image recognition, an intricate orchestration of data, algorithms, and computational power, is playing a pivotal role in this transformation.
In the realm of healthcare, medical image analysis employs advanced AI techniques to significantly improve diagnostic accuracy and patient outcomes. These systems enable healthcare professionals to detect anomalies and diseases with greater precision, often at much earlier stages. The use of AI for analyzing X-rays, MRIs, and other medical scans has demonstrated a high success rate, particularly in areas like skin cancer diagnosis where precision is paramount.
Turning to the agricultural sector, automation in agriculture through AI has seen innovative applications in monitoring crop health and managing farm operations. Utilizing drones and satellite imagery, AI-driven systems analyze crop conditions across vast areas, informing farmers about optimal planting times, soil health, and crop needs. This automation isn't just about saving time; it's about enhancing yield and sustainability through precise resource management.
Moreover, these advancements in AI image recognition also stimulate considerable economic growth and sector efficiency. For instance, the retail industry is using AI to refine customer experiences through personalized shopping and inventory management, proving that the potential applications of AI image recognition extend far beyond just identifying and analyzing images.
The impact of AI image recognition is rounded out by its role in other fields such as automotive for enhancing navigation and safety, and in environmental monitoring where it aids in wildlife conservation and pollution control. Each application underscores the technology's broad applicability and potential to revolutionize traditional practices across various industries.
While the technological feats are impressive, it's crucial to balance innovation with considerations of privacy, data security, and ethical implications. The growth of AI in sectors like healthcare and agriculture presents not just opportunities but also challenges that need thoughtful navigation.
Indeed, the transformative impact of AI image recognition is reshaping industries, driving them towards more efficient, precise, and innovative futures. The continued integration of this technology promises not only to enhance operational efficiencies but also to pioneer new methods of business and scientific engagement that were previously unimaginable.
Redrawing Limits: Advances in Computer Vision with AI
The progression of computer vision empowered by AI technologies unfolds before us, bringing with it astonishing advancements that continue to redraw the boundaries of what machines can perceive and understand. With the inception of breakthrough AI models, each iteration outperforms the last, a testament to the relentless pursuit of excellence in the realm of artificial intelligence. Notably, the Mask RCNN algorithm set the stage in 2017 with a frame inference time of 330ms, but it was soon eclipsed by the likes of the YOLOR algorithm, YOLOv7, and the more recent YOLOv8 and YOLOv9, which have pushed the envelope in real-time object detection and model training efficiency.
The Incredible Progress in Algorithm Performance
The data speaks volumes: from the YOLOR algorithm's impressive 12ms inference time in 2021 to the launch of YOLOv9's architecture, AI image recognition has transitioned from requiring vast datasets to learning from merely tens of samples, enhancing model training processes drastically. Determined by parameters that gauge accuracy confidence and error rates, models have become highly adept at interpreting complex visual data. These strides in computer vision have also sparked heightened interest and investment in AI research and development, which, according to sources like PwC, is projected to surge over 30% globally, solidifying AI's role in future innovation.
Future Directions in AI-Driven Computer Vision
The implications of these progresses in meta-learning in image processing and neurosymbolic AI are profound, promising enhanced interpretability and adaptability in AI systems. Not only do these advances bolster the effectiveness of artificial cognition, but they also open up avenues for personalized and preventive applications, such as in medical diagnostics where AI's pinpoint precision can be life-saving. To further understand the potential, one only needs to consider the 50% uptick in adoption of Advanced Language Models across industries for functions ranging from customer interaction to translation—a glimpse into a future enriched by AI.
Emergence of Semi-Supervised and Generative Models
As we edge closer to a more nuanced and autonomous AI, semi-supervised and generative models enter the spotlight. These paradigms promise to further minimize human intervention, shaping a landscape where AI can perform at unprecedented levels with less reliance on labeled data—a crucial step in realizing AI's scalability across diverse applications. AI-powered image recognition now boasts unparalleled accuracy, revolutionizing sectors and setting a standard for what we can anticipate as the seamless integration of AI continues to transform our world.
FAQ
What is AI image recognition and how does it work?
AI image recognition is a process where computers use artificial intelligence and computer vision technologies to analyze digital images. It involves identifying and classifying objects in images, which is accomplished through the use of machine learning algorithms like neural networks. These algorithms compare pixel patterns to recognize and categorize different elements in the image.
How has AI image recognition evolved from traditional methods?
Traditional image recognition methods required manual feature extractions and rule-based systems. With the evolution of AI, image recognition now predominantly uses machine learning and deep learning to learn from large datasets. Algorithms like the YOLOv8 and YOLOv9 have surpassed older methods with their capabilities to process images with greater speed and higher accuracy.
Why are neural networks important in AI image recognition?
Neural networks, especially convolutional neural networks (CNNs), are crucial in AI image recognition because they are adept at handling complex visual data. They mimic the way the human brain processes images and are able to learn hierarchically, extracting features from raw pixels without needing explicit programming, which enhances the recognition process significantly.
What are some applications of AI in image analysis?
The applications of AI in image analysis are vast and diverse. They range from precision agriculture, where it's used to monitor crop health, to the medical field for anomaly detection in scans. AI is also used in autonomous vehicles for detecting pedestrians, in retail for store layout optimization, and in entertainment for creating visual effects, among many others.
How does machine learning fit into AI image recognition?
Machine learning is a foundational component of AI image recognition. It involves training algorithms to learn from data, improve through experience, and make decisions based on new images they encounter. Machine learning applications facilitate tasks such as image classification, object detection, and object segmentation, making AI systems more accurate and efficient.
What is the role of data in training AI for image recognition?
Data acts as the learning material for AI models. A diverse collection of labeled images is crucial as it exposes the AI to various instances it needs to recognize. These datasets are used for training the model, with processes like preprocessing and annotation being key to accurately teach the AI to find patterns and make correct identifications and classifications.
How do traditional image processing techniques compare to deep learning approaches?
Traditional image processing techniques, such as Support Vector Machines (SVMs) and Local Binary Patterns (LBP), rely on manual feature extraction and are generally less flexible. Deep learning approaches, particularly convolutional neural networks (CNNs), offer superior performance by automatically learning and extracting hierarchical features from images, thus providing better flexibility and results in complex tasks.
What hardware and software are needed for AI image recognition?
Efficient AI image recognition requires high-performance hardware and sophisticated software. GPUs are commonly used for their computational power, which is necessary for processing large amounts of visual data by deep learning models.
How is AI image recognition transformative across different industries?
AI image recognition is changing the landscape of multiple industries by introducing unprecedented levels of automation and enhanced decision-making. It enables detailed crop monitoring in agriculture, advanced diagnostics in healthcare, and safer navigation systems in automotive applications. In retail and entertainment, AI is used to enhance customer experiences, showcasing its versatility and impact across sectors.
What are the latest advancements in computer vision with AI?
The field has seen remarkable advancements such as the development of highly efficient algorithms like YOLOv8 and YOLOv9, the exploration of neurosymbolic AI for combining learning with reasoning, and the advent of meta-learning which allows AI to adapt to new tasks more effectively. Additionally, semi-supervised and generative models are reducing the dependency on extensive labeled datasets, making AI image recognition more accessible and powerful.
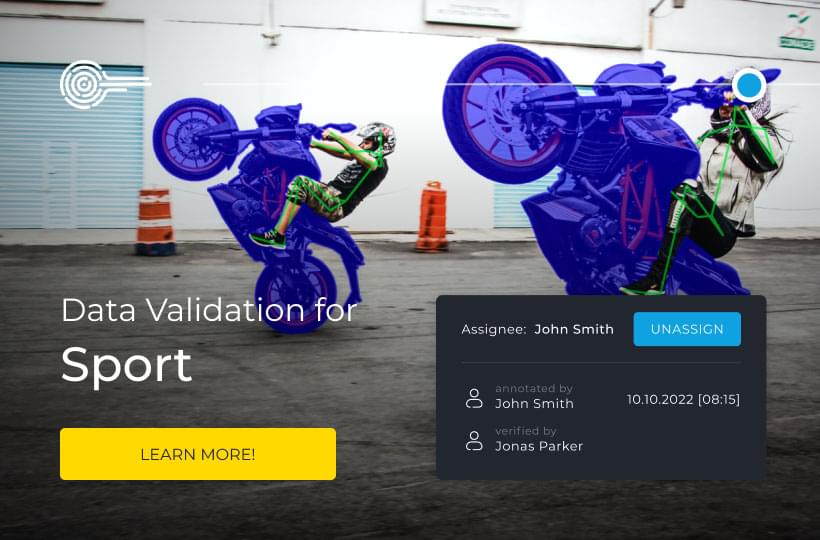